Flexible Isosurface Extraction for Gradient-Based Mesh Optimization
CoRR(2023)
摘要
This work considers gradient-based mesh optimization, where we iteratively optimize for a 3D surface mesh by representing it as the isosurface of a scalar field, an increasingly common paradigm in applications including photogrammetry, generative modeling, and inverse physics. Existing implementations adapt classic isosurface extraction algorithms like Marching Cubes or Dual Contouring; these techniques were designed to extract meshes from fixed, known fields, and in the optimization setting they lack the degrees of freedom to represent high-quality feature-preserving meshes, or suffer from numerical instabilities. We introduce FlexiCubes, an isosurface representation specifically designed for optimizing an unknown mesh with respect to geometric, visual, or even physical objectives. Our main insight is to introduce additional carefully-chosen parameters into the representation, which allow local flexible adjustments to the extracted mesh geometry and connectivity. These parameters are updated along with the underlying scalar field via automatic differentiation when optimizing for a downstream task. We base our extraction scheme on Dual Marching Cubes for improved topological properties, and present extensions to optionally generate tetrahedral and hierarchically-adaptive meshes. Extensive experiments validate FlexiCubes on both synthetic benchmarks and real-world applications, showing that it offers significant improvements in mesh quality and geometric fidelity.
更多查看译文
关键词
isosurface extraction,gradient-based mesh optimization,photogrammetry,generative models
AI 理解论文
溯源树
样例
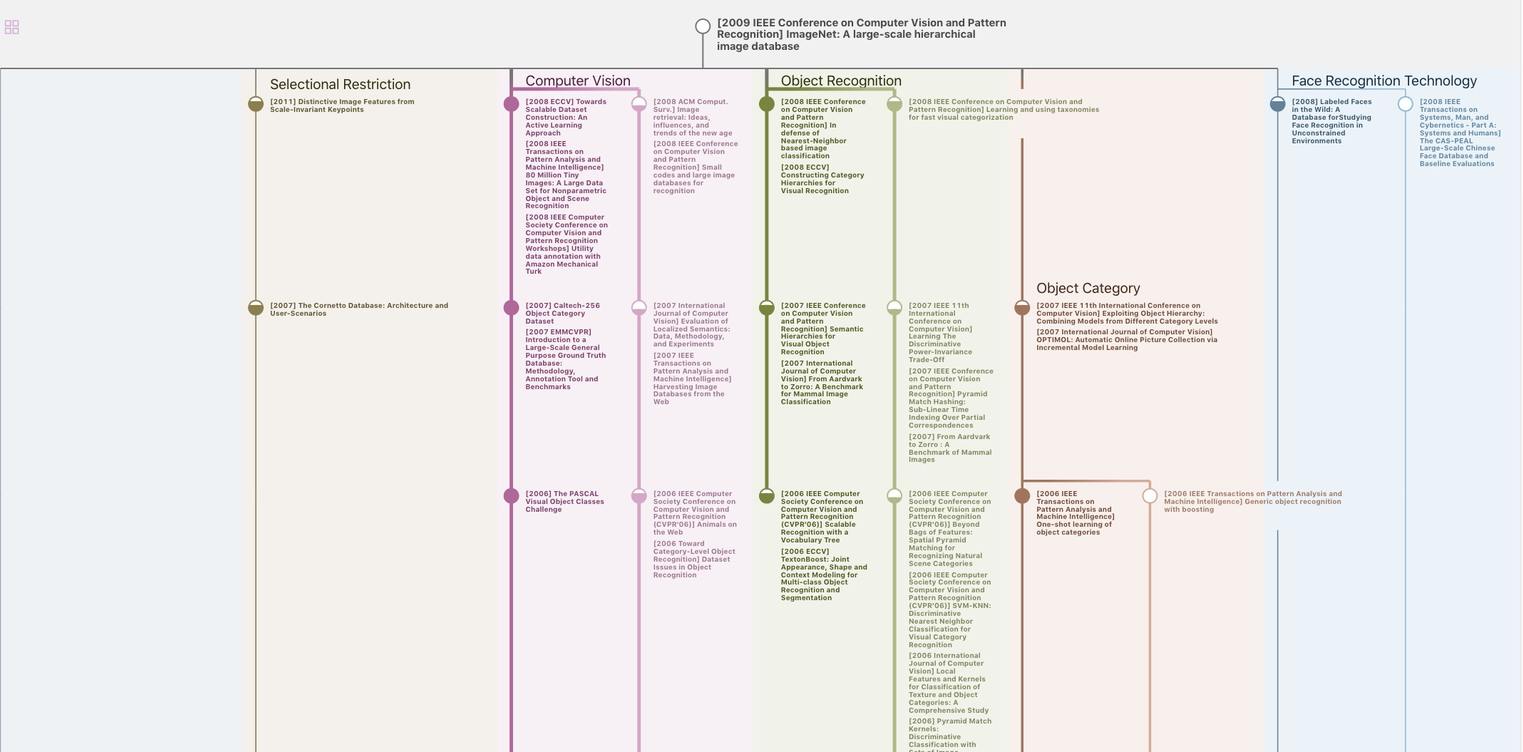
生成溯源树,研究论文发展脉络
Chat Paper
正在生成论文摘要