SPCNet: a strip pyramid ConvNeXt network for detection of road surface defects
Signal, Image and Video Processing(2024)
摘要
Road surface defect detection plays an important role in the construction and maintenance of roads. However, the irregularity of road surface defects and the complexity of the background make the extraction of road surface defects very difficult. It is a challenge to extract the road surface defects accurately. To cope with this challenge, we introduce the theory of image segmentation in deep learning. However, existing deep learning networks suffer from insufficient segmentation accuracy, low model robustness, and a lack of generalization ability. Consequently, we propose a novel deep learning network named Strip Pyramid ConvNeXt Network for detecting road surface defects. Firstly, we introduced ConvNeXt as the encoder to ensure the segmentation accuracy of the model. Furthermore, we designed a strip pyramid pooling module with excellent edge detail extraction capability and a multi-feature fusion module. We also created a cementation fissure dataset (CE dataset) to test the accuracy of the model and verify the generalization capability and robustness of the model. Finally, we compared our model with ten advanced segmentation networks in recent years on CRACK500 dataset, GAPs384 dataset, and cementation fissure dataset (CE dataset), and our model outperforms others on four metrics.
更多查看译文
关键词
Road defect detection,Deep learning,ConvNeXt,Cementation fissure dataset
AI 理解论文
溯源树
样例
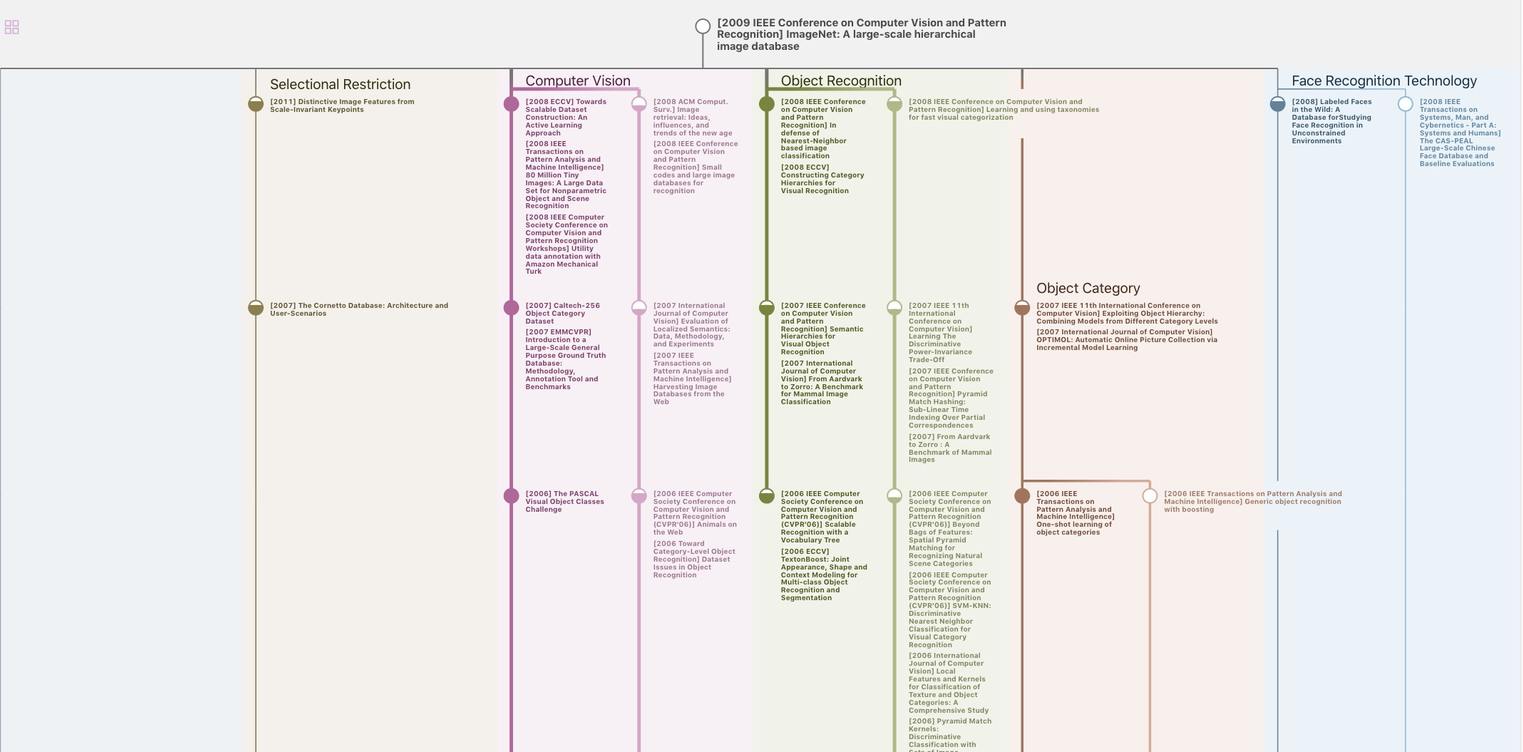
生成溯源树,研究论文发展脉络
Chat Paper
正在生成论文摘要