Watch Your Steps: Local Image and Scene Editing by Text Instructions
CoRR(2023)
摘要
Denoising diffusion models have enabled high-quality image generation and editing. We present a method to localize the desired edit region implicit in a text instruction. We leverage InstructPix2Pix (IP2P) and identify the discrepancy between IP2P predictions with and without the instruction. This discrepancy is referred to as the relevance map. The relevance map conveys the importance of changing each pixel to achieve the edits, and is used to to guide the modifications. This guidance ensures that the irrelevant pixels remain unchanged. Relevance maps are further used to enhance the quality of text-guided editing of 3D scenes in the form of neural radiance fields. A field is trained on relevance maps of training views, denoted as the relevance field, defining the 3D region within which modifications should be made. We perform iterative updates on the training views guided by rendered relevance maps from the relevance field. Our method achieves state-of-the-art performance on both image and NeRF editing tasks. Project page: https://ashmrz.github.io/WatchYourSteps/
更多查看译文
关键词
scene editing,local image,instructions,text
AI 理解论文
溯源树
样例
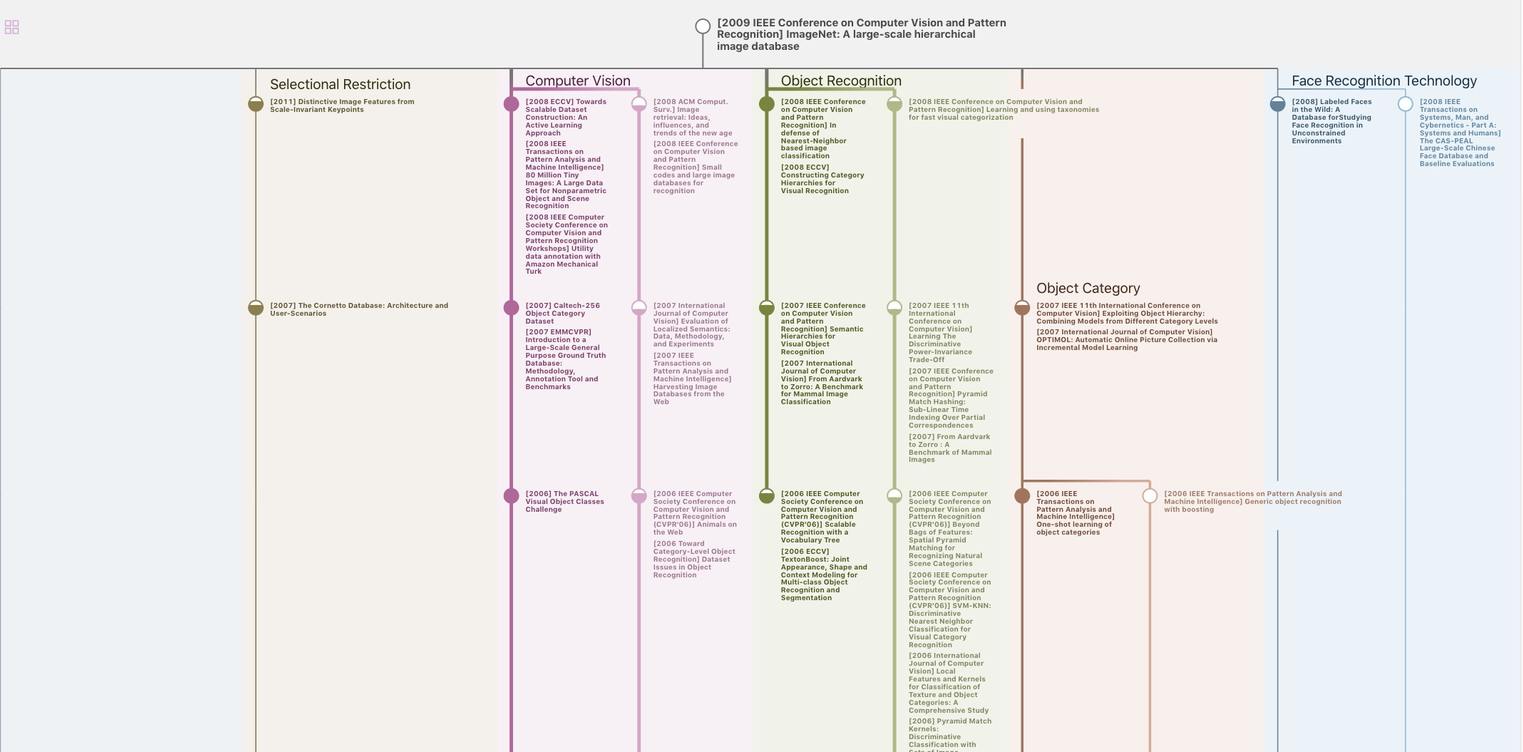
生成溯源树,研究论文发展脉络
Chat Paper
正在生成论文摘要