HarvestNet: A Dataset for Detecting Smallholder Farming Activity Using Harvest Piles and Remote Sensing
AAAI 2024(2024)
摘要
Small farms contribute to a large share of the productive land in developing countries. In regions such as sub-Saharan Africa, where 80% of farms are small (under 2 ha in size), the task of mapping smallholder cropland is an important part of tracking sustainability measures such as crop productivity. However, the visually diverse and nuanced appearance of small farms has limited the effectiveness of traditional approaches to cropland mapping. Here we introduce a new approach based on the detection of harvest piles characteristic of many smallholder systems throughout the world. We present HarvestNet, a dataset for mapping the presence of farms in the Ethiopian regions of Tigray and Amhara during 2020-2023, collected using expert knowledge and satellite images, totalling 7k hand-labeled images and 2k ground-collected labels. We also benchmark a set of baselines, including SOTA models in remote sensing, with our best models having around 80% classification performance on hand labelled data and 90% and 98% accuracy on ground truth data for Tigray and Amhara, respectively. We also perform a visual comparison with a widely used pre-existing coverage map and show that our model detects an extra 56,621 hectares of cropland in Tigray. We conclude that remote sensing of harvest piles can contribute to more timely and accurate cropland assessments in food insecure regions. The dataset can be accessed through https://figshare.com/s/45a7b45556b90a9a11d2, while the code for the dataset and benchmarks is publicly available at https://github.com/jonxuxu/harvest-piles
更多查看译文
关键词
General
AI 理解论文
溯源树
样例
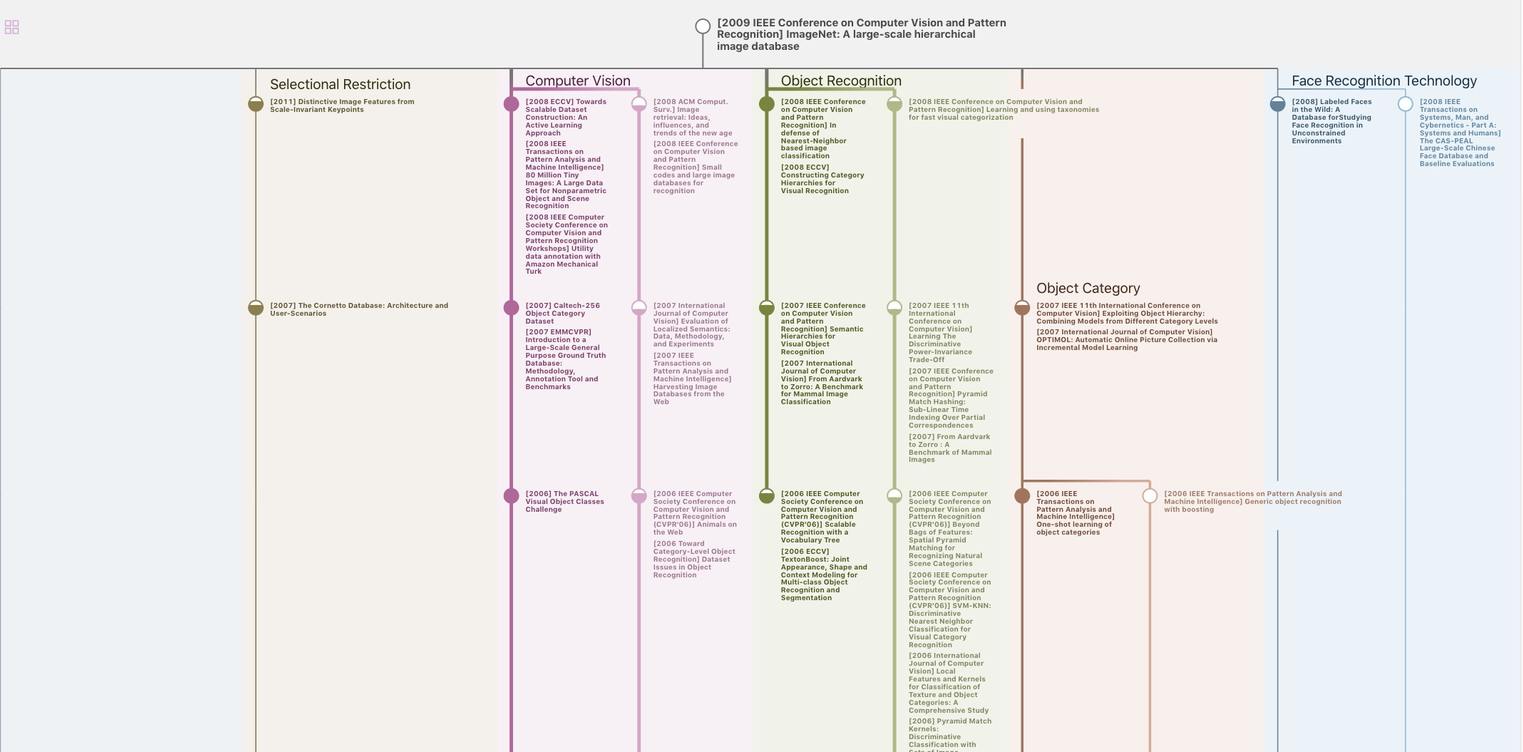
生成溯源树,研究论文发展脉络
Chat Paper
正在生成论文摘要