Video Corpus Moment Retrieval via Deformable Multigranularity Feature Fusion and Adversarial Training
IEEE Transactions on Circuits and Systems for Video Technology(2023)
摘要
As a new emerging task, video corpus moment retrieval (VCMR) aims to find the video segments relevant to a given natural language query from a large number of untrimmed videos. It mainly includes two subtasks, finding the most relevant video based on the query text (video retrieval), and locating the segment most relevant to a given query in a video (moment localization). At the same time, since videos often contain rich multi-modal information such as audio, text, and images, how to align and interact with the multi-modal information of videos and the text information of natural language queries across modalities is the core issue of this task. This article proposes a Deformable Multigranularity Feature Fusion with Adversarial Training Network (DMFAT), first inputs the subtitle and frame multi-modal information of the video into our Multi-Scale Deformable Attention module and performs multi-granularity feature fusion through Deformable Attention respectively. Then, guided by the query, adaptive weights are generated to fuse the two multi-granularity modality features of the video. Finally, the cross-modal representation of the query and video features is obtained through a bidirectional attention module, and an adversarial contrastive learning objective is introduced to enhance more precise moment localization. Our model is evaluated on two representative video corpus moment retrieval benchmarks: TVR and DiDeMo. Extensive experiments have been conducted to demonstrate that our method outperforms existing work.
更多查看译文
关键词
Video Corpus Moment Retrieval,Deformable Attention,Multi-granularity,Adversarial Training
AI 理解论文
溯源树
样例
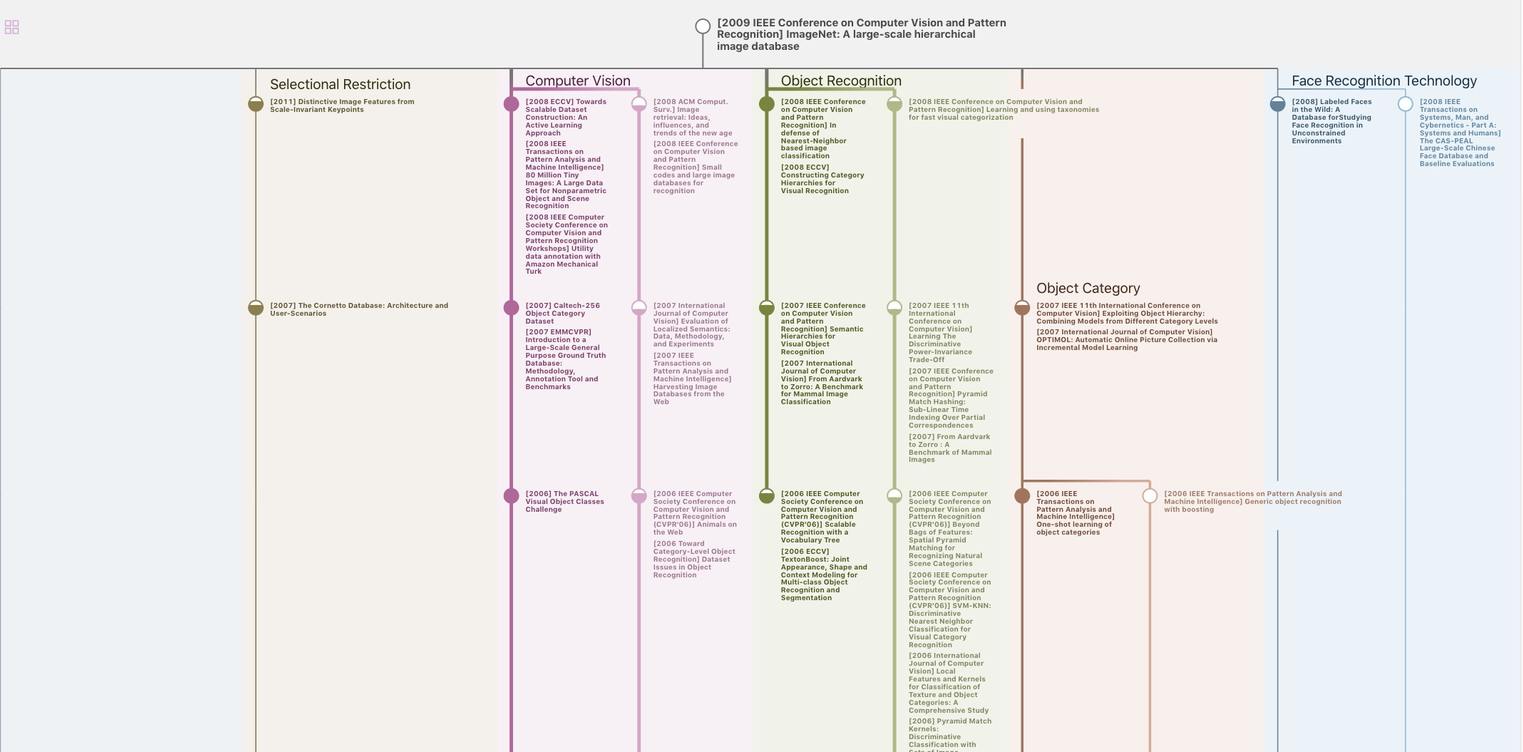
生成溯源树,研究论文发展脉络
Chat Paper
正在生成论文摘要