Evaluating ChatGPT in Information Extraction: A Case Study of Extracting Cognitive Exam Dates and Scores
medRxiv (Cold Spring Harbor Laboratory)(2023)
摘要
Background Large language models (LLMs) provide powerful natural language processing (NLP) capabilities in medical and clinical tasks. Evaluating LLM performance is crucial due to potential false results. In this study, we assessed ChatGPT, a state-of-the-art LLM, in extracting information from clinical notes, focusing on cognitive tests, specifically the Mini Mental State Exam (MMSE) and the Cognitive Dementia Rating (CDR). We tasked ChatGPT with extracting MMSE and CDR scores and corresponding dates from clinical notes.
Methods Our cohort had 135,307 clinical notes (Jan 12th, 2010 to May 24th, 2023) mentioning MMSE, CDR, or Montreal Cognitive Assessment (MoCA). After applying inclusion criteria and excluding notes with only MoCA, 34,465 notes remained. Among them, 765 were randomly selected and underwent analysis by ChatGPT. 22 medically-trained experts reviewed ChatGPT’s responses and provided ground truth. ChatGPT (GPT-4, version "2023-03-15-preview") was used on the 765 notes to extract MMSE and CDR instances with corresponding dates. Inference was successful for 742 notes. We used 20 notes for fine-tuning and training the reviewers. The remaining 722 were assigned to reviewers, with 309 assigned to two reviewers simultaneously. Inter-rater-agreement (Fleiss’ Kappa), precision, recall, true/false negative rates, and accuracy were calculated.
Results For MMSE information extraction, ChatGPT achieved 83% accuracy. It demonstrated high sensitivity with a Macro-recall of 89.7% and outstanding true-negative rates of 96%. The precision for MMSE was also high at 82.7%. In the case of CDR information extraction, ChatGPT achieved 89% accuracy. It showed excellent sensitivity with a Macro-recall of 91.3% and a perfect true-negative rate of 100%. However, the precision for CDR was lower at 57%. Analyzing the ground truth data, it was found that 89.1% of the notes included an MMSE documentation, whereas only 14.3% had a CDR documentation, which affected the precision of CDR extraction. Inter-rater-agreement was substantial, supporting the validity of our findings. Reviewers considered ChatGPT’s responses correct (96% for MMSE, 98% for CDR) and complete (84% for MMSE, 83% for CDR).
Conclusion ChatGPT demonstrates overall accuracy in extracting MMSE and CDR scores and dates, potentially benefiting dementia research and clinical care. Prior probability of the information appearing in the text impacted ChatGPT’s precision. Rigorous evaluation of LLMs for diverse medical tasks is crucial to understand their capabilities and limitations.
### Competing Interest Statement
The authors have declared no competing interest.
### Funding Statement
NYU Langone Health MCIT.
### Author Declarations
I confirm all relevant ethical guidelines have been followed, and any necessary IRB and/or ethics committee approvals have been obtained.
Yes
The details of the IRB/oversight body that provided approval or exemption for the research described are given below:
Ethics committee/IRB of New York University Langone Health gave ethical approval of this work.
I confirm that all necessary patient/participant consent has been obtained and the appropriate institutional forms have been archived, and that any patient/participant/sample identifiers included were not known to anyone (e.g., hospital staff, patients or participants themselves) outside the research group so cannot be used to identify individuals.
Yes
I understand that all clinical trials and any other prospective interventional studies must be registered with an ICMJE-approved registry, such as ClinicalTrials.gov. I confirm that any such study reported in the manuscript has been registered and the trial registration ID is provided (note: if posting a prospective study registered retrospectively, please provide a statement in the trial ID field explaining why the study was not registered in advance).
Yes
I have followed all appropriate research reporting guidelines, such as any relevant EQUATOR Network research reporting checklist(s) and other pertinent material, if applicable.
Yes
The data contains private patient information and will not be made available.
更多查看译文
关键词
cognitive exam dates,information extraction,chatgpt,study
AI 理解论文
溯源树
样例
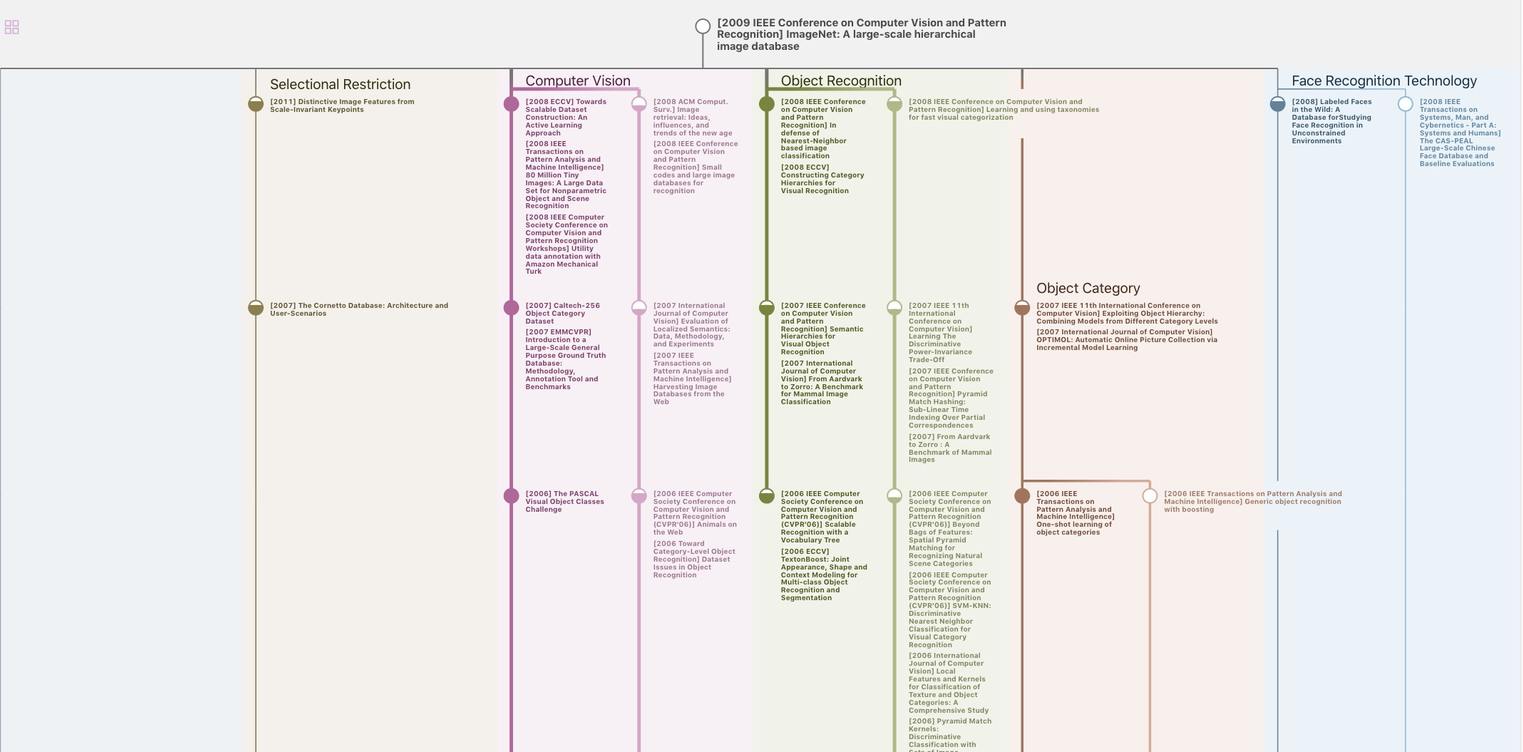
生成溯源树,研究论文发展脉络
Chat Paper
正在生成论文摘要