RoboTAP: Tracking Arbitrary Points for Few-Shot Visual Imitation
CoRR(2023)
摘要
For robots to be useful outside labs and specialized factories we need a way to teach them new useful behaviors quickly. Current approaches lack either the generality to onboard new tasks without task-specific engineering, or else lack the data-efficiency to do so in an amount of time that enables practical use. In this work we explore dense tracking as a representational vehicle to allow faster and more general learning from demonstration. Our approach utilizes Track-Any-Point (TAP) models to isolate the relevant motion in a demonstration, and parameterize a low-level controller to reproduce this motion across changes in the scene configuration. We show this results in robust robot policies that can solve complex object-arrangement tasks such as shape-matching, stacking, and even full path-following tasks such as applying glue and sticking objects together, all from demonstrations that can be collected in minutes.
更多查看译文
关键词
arbitrary points,few-shot
AI 理解论文
溯源树
样例
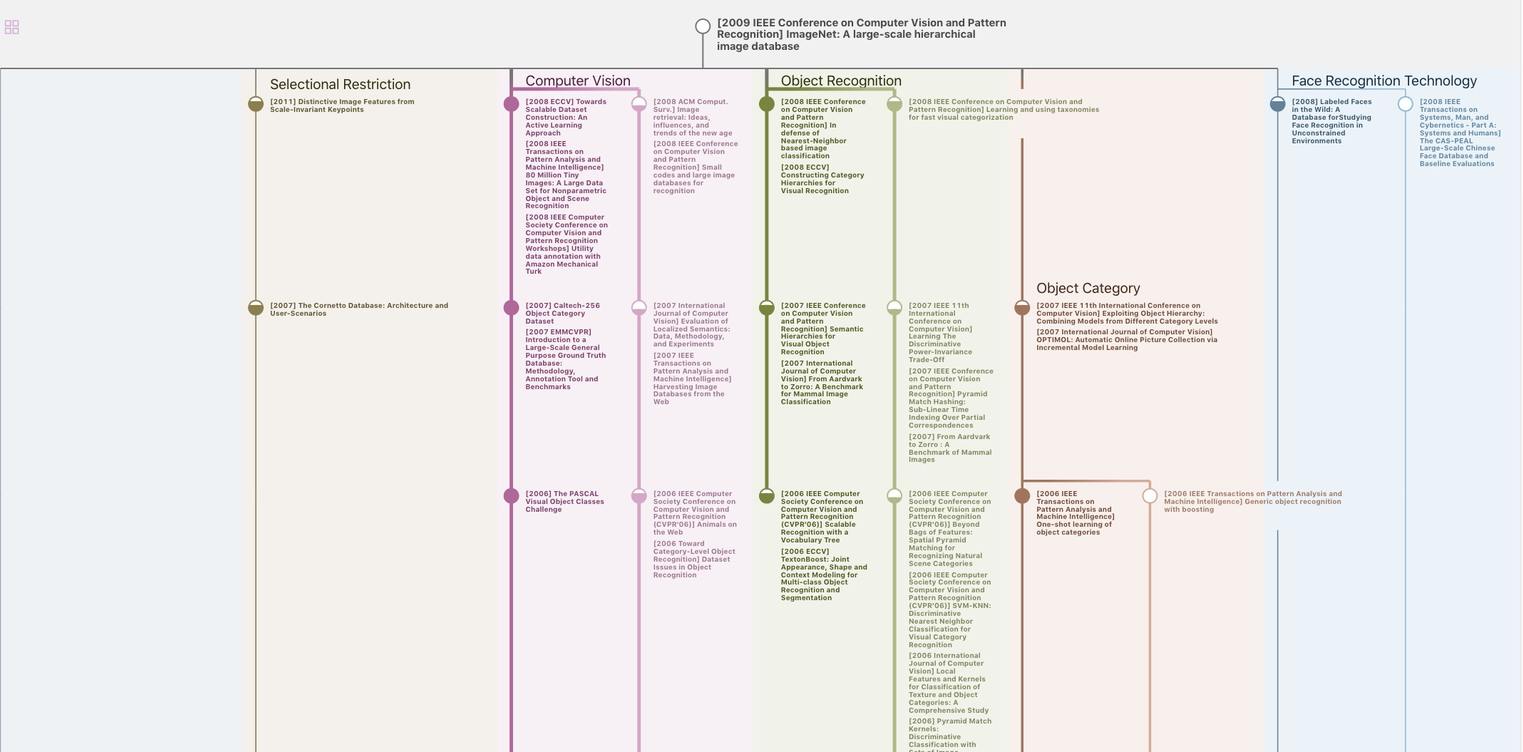
生成溯源树,研究论文发展脉络
Chat Paper
正在生成论文摘要