Recommendation with Dynamic Natural Language Explanations.
IJCNN(2023)
摘要
Explaining recommendation with natural languages has shown to be an effective strategy to improve the recommendation pervasiveness and user satisfaction. While recent years have witnessed many promising models, they mostly consider the user-item interactions as independent samples. However, in real-world scenarios, the user preference is always dynamic and evolving, and the current user behaviors may have strong correlations with the previous ones. To bridge this gap, in this paper, we propose to build an explainable recommender model by considering the user dynamic preference. Our general idea is to build a sequential model to capture the user history behaviors, and then the explanations are generated by summarizing all the past interactions. In specific, we firstly deploy two independent components to model the user sequential interactions and reviews separately. Then, we design a duration-aware attention mechanism to discriminate the importance of different items and reviews. For more effectively modeling the history information, we introduce a denoising module to remove the user behaviors which are less important for the current prediction. We conduct extensive experiments to demonstrate the effectiveness of our model based on three real-world datasets, in which the best performance can be improved by about 13.3%, 6.5%, 5.0% and 1.9% on the metrics of BLEU-1, ROUGE-1, ROUGE-2 and MAE, respectively. In addition, we also evaluate the generated explanations from both qualitative and qualitative perspectives.
更多查看译文
关键词
recommendation system,explainable recommendation,dynamic user preference
AI 理解论文
溯源树
样例
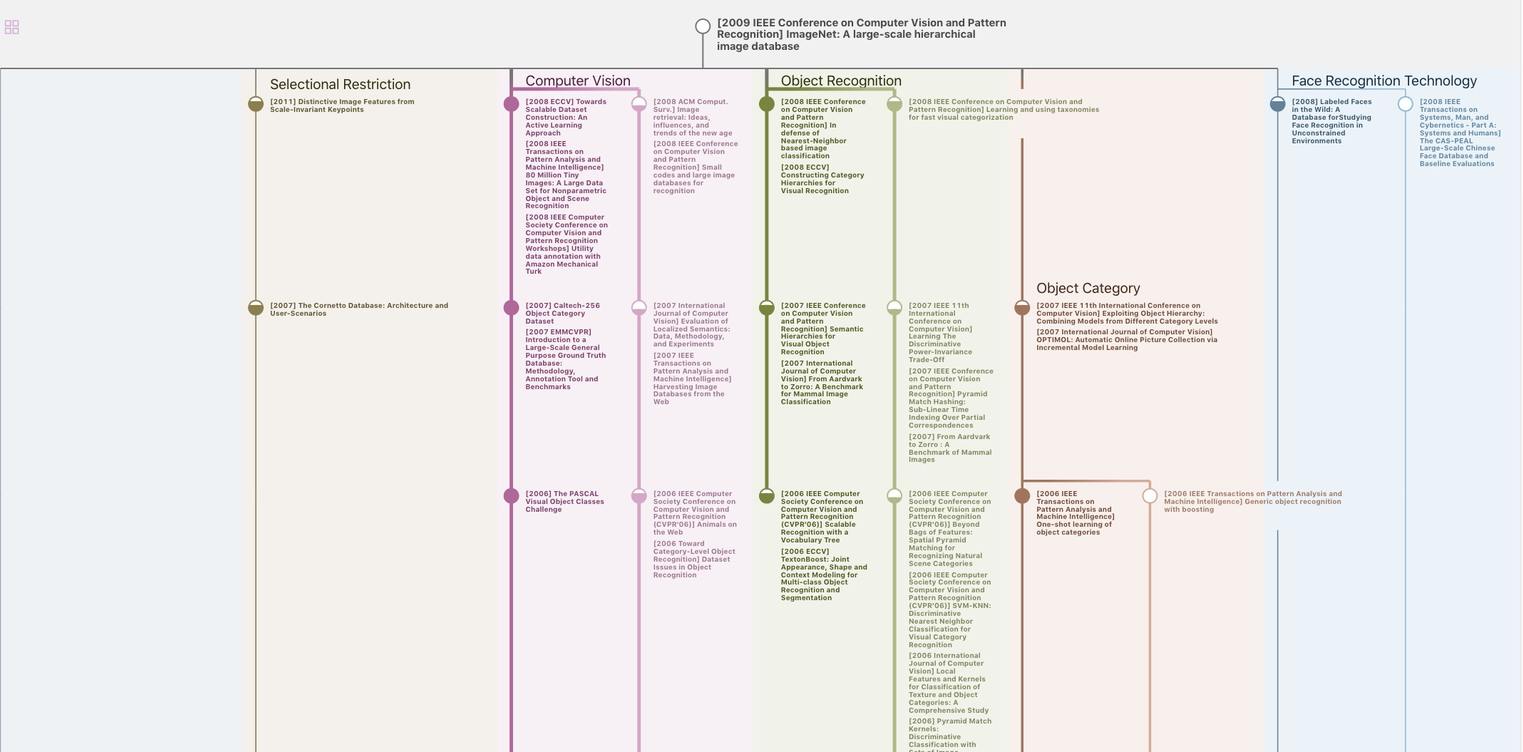
生成溯源树,研究论文发展脉络
Chat Paper
正在生成论文摘要