Interfacing Neuromorphic Hardware with Machine Learning Frameworks - A Review.
ICONS(2023)
摘要
With the emergence of neuromorphic hardware as a promising low-power parallel computing platform, the need for tools that allow researchers and engineers to efficiently interact with such hardware is rapidly growing. Machine learning frameworks like Tensorflow, PyTorch and JAX have been instrumental for the success of machine learning in recent years as they enable seamless interaction with traditional machine learning accelerators such as GPUs and TPUs. In stark contrast, interfacing with neuromorphic hardware remains difficult since the aforementioned frameworks do not address the challenges associated with mapping neural network models and algorithms to physical hardware. In this paper, we review the various strategies employed throughout the neuromorphic computing community to tackle these challenges and categorize them according to their methodologies and implementation effort. This classification serves as a guideline for device engineers and software developers alike to enable them to choose the best-fit solution in regard of their demands and available resources. Finally, we provide a JAX-based proof-of-concept implementation of a compilation pipeline tailored to the needs of researchers in the early stages of device development, where parts of the computational graph can be mapped onto custom hardware via operations exposed through a C++ or Python interface. The code is available at https://github.com/PGI15/xbarax.
更多查看译文
AI 理解论文
溯源树
样例
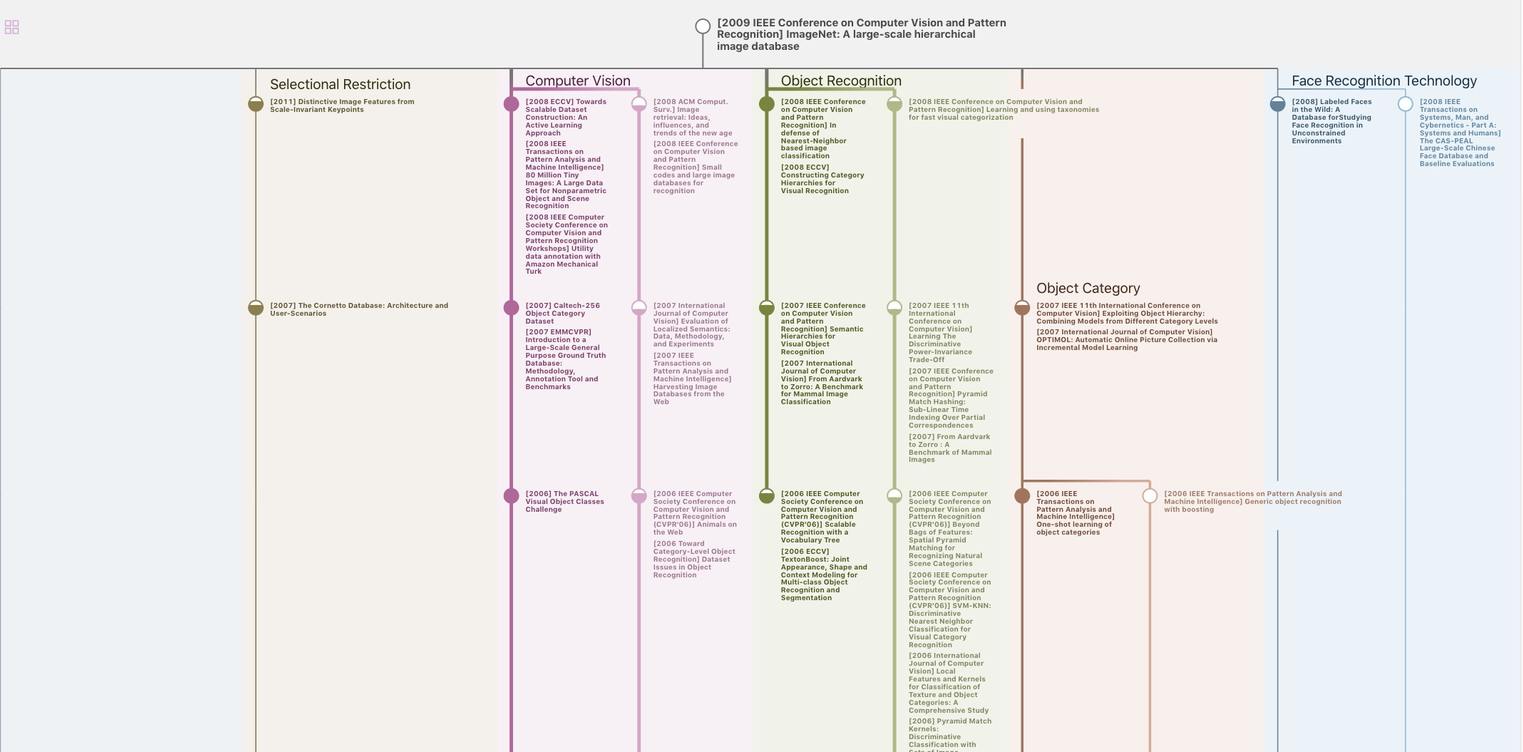
生成溯源树,研究论文发展脉络
Chat Paper
正在生成论文摘要