Diffusion-Enhanced PatchMatch: A Framework for Arbitrary Style Transfer with Diffusion Models
CVPR Workshops(2023)
摘要
Diffusion models have gained immense popularity in recent years due to their impressive ability to generate high-quality images. The opportunities that diffusion models provide are numerous, from text-to-image synthesis to image restoration and enhancement, as well as image compression and inpainting. However, expressing image style in words can be a challenging task, making it difficult for diffusion models to generate images with specific style without additional optimization techniques. In this paper, we present a novel method, Diffusion-Enhanced PatchMatch (DEPM), that leverages Stable Diffusion for style transfer without any finetuning or pretraining. DEPM captures high-level style features while preserving the fine-grained texture details of the original image. By enabling the transfer of arbitrary styles during inference, our approach makes the process more flexible and efficient. Moreover, its optimization-free nature makes it accessible to a wide range of users.
更多查看译文
关键词
arbitrary style transfer,arbitrary styles,DEPM captures high-level style features,diffusion models,Diffusion-Enhanced PatchMatch,high-quality images,image compression,image restoration,image style,inpainting,specific style,Stable Diffusion,text-to-image synthesis
AI 理解论文
溯源树
样例
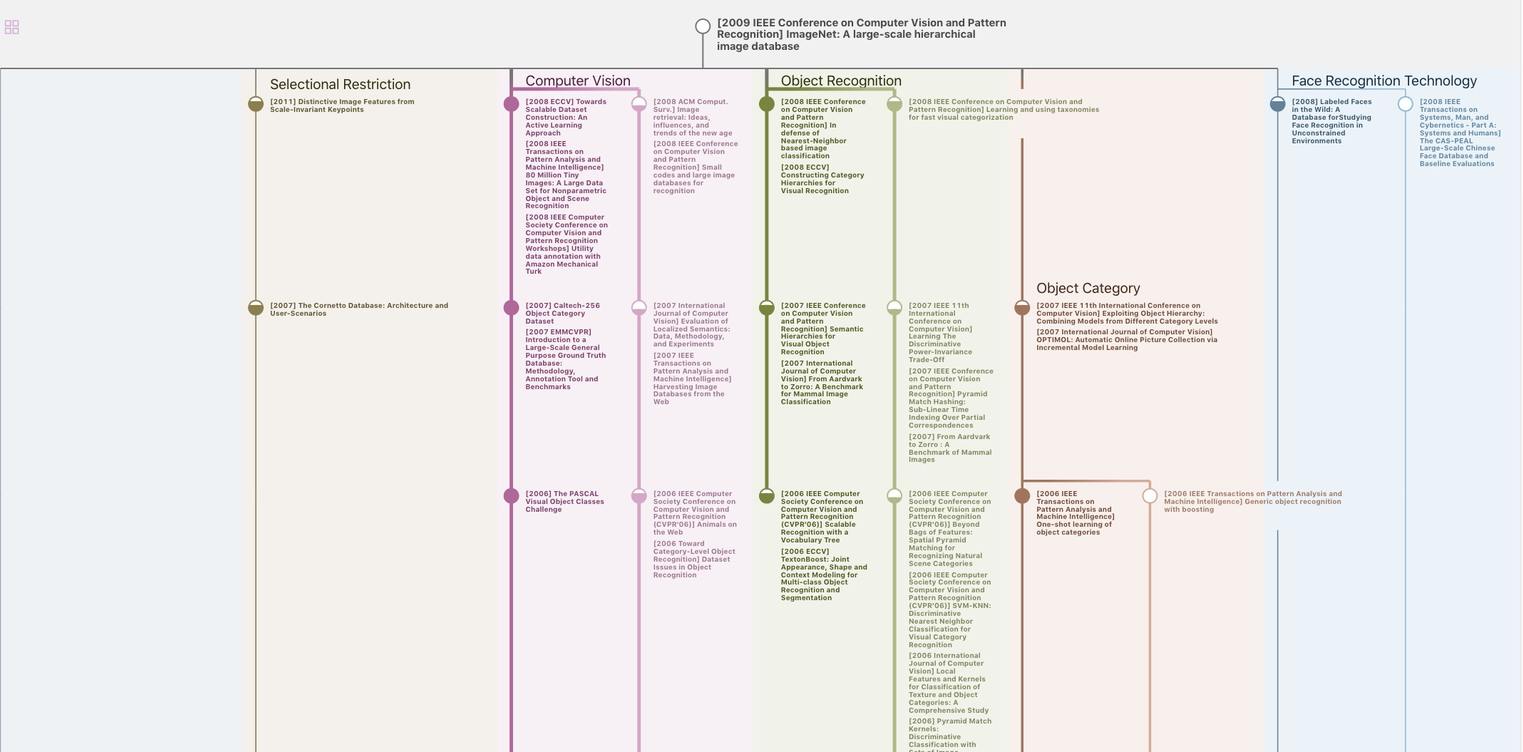
生成溯源树,研究论文发展脉络
Chat Paper
正在生成论文摘要