Multi-Step Hindsight Experience Replay with Bias Reduction for Efficient Multi-Goal Reinforcement Learning
2023 International Conference on Frontiers of Robotics and Software Engineering (FRSE)(2023)
摘要
Multi-goal reinforcement learning has emerged as a powerful approach for planning and robot manipulation tasks, but it faces challenges such as sparse rewards and sample inefficiency. Hindsight Experience Replay (HER) has been proposed as a solution to these challenges by relabeling goals, but it still requires a large number of samples and significant computation. To address these issues, we propose Multi-step Hindsight Experience Replay (MHER), which incorporates multi-step relabeling to improve sample efficiency. Despite the advantages of $n -$step relabeling, we theoretically and experimentally prove the off-policy $n -$step bias introduced by $n -$step relabeling may lead to poor performance in many environments. To address this issue, two bias-reduced MHER algorithms, MHER $( \lambda )$ and Model-based MHER (MMHER) are presented. MHER $( \lambda )$ exploits the $\lambda$ return while MMHER benefits from model-based value expansions. Experimental results on numerous multi-goal robotic tasks show that our solutions can successfully alleviate the off-policy $n -$step bias and achieve significantly higher sample efficiency than previous multi-goal RL baselines with little additional computation beyond HER.
更多查看译文
关键词
Multi-goal Reinforcement Learning,hindsight experience replay,off-policy evaluation
AI 理解论文
溯源树
样例
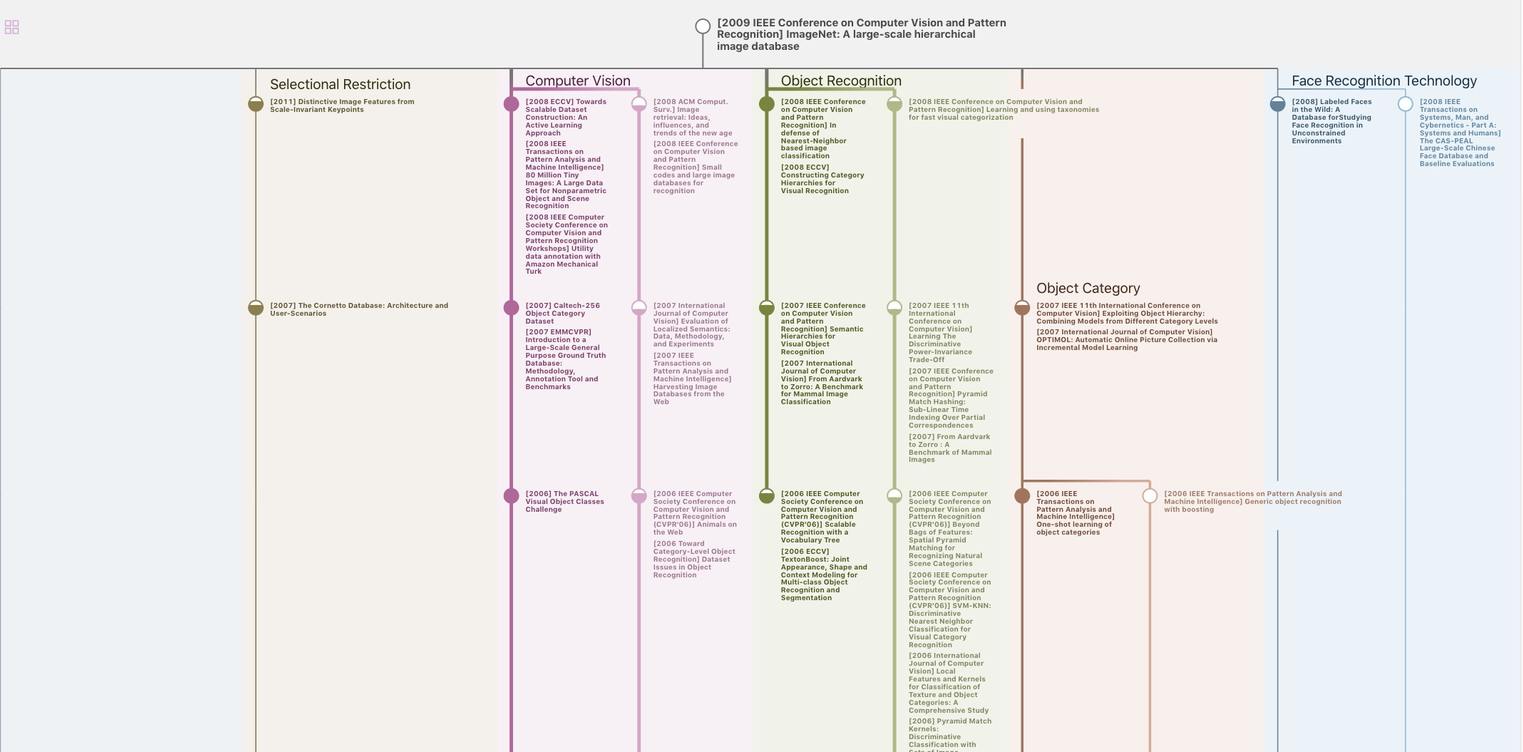
生成溯源树,研究论文发展脉络
Chat Paper
正在生成论文摘要