OCC-VO: Dense Mapping Via 3D Occupancy-Based Visual Odometry for Autonomous Driving
arXiv (Cornell University)(2023)
摘要
Visual Odometry (VO) plays a pivotal role in autonomous systems, with a principal challenge being the lack of depth information in camera images. This paper introduces OCC-VO, a novel framework that capitalizes on recent advances in deep learning to transform 2D camera images into 3D semantic occupancy, thereby circumventing the traditional need for concurrent estimation of ego poses and landmark locations. Within this framework, we utilize the TPV-Former to convert surround view cameras' images into 3D semantic occupancy. Addressing the challenges presented by this transformation, we have specifically tailored a pose estimation and mapping algorithm that incorporates Semantic Label Filter, Dynamic Object Filter, and finally, utilizes Voxel PFilter for maintaining a consistent global semantic map. Evaluations on the Occ3D-nuScenes not only showcase a 20.6% improvement in Success Ratio and a 29.6% enhancement in trajectory accuracy against ORB-SLAM3, but also emphasize our ability to construct a comprehensive map. Our implementation is open-sourced and available at: https://github.com/USTCLH/OCC-VO.
更多查看译文
关键词
Mapping,SLAM
AI 理解论文
溯源树
样例
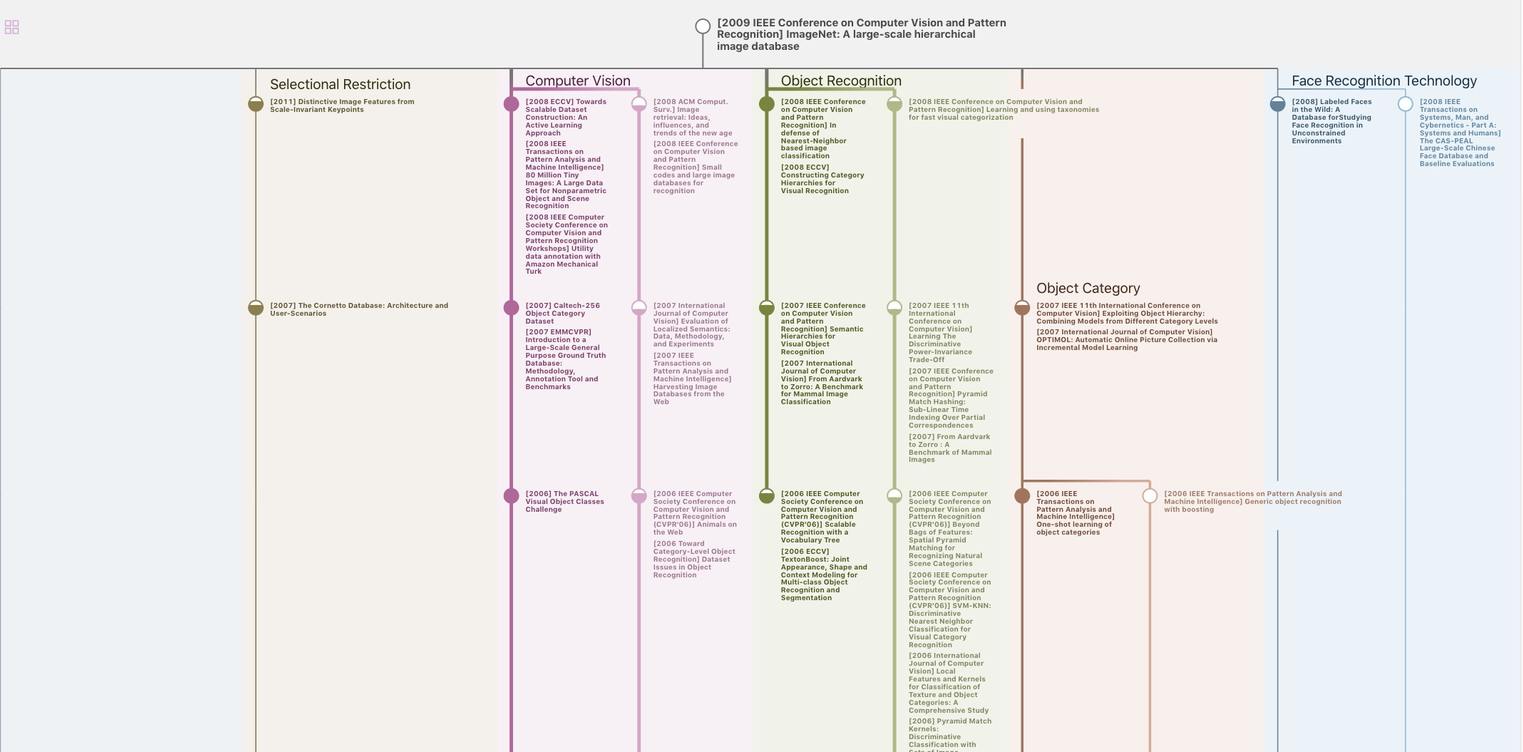
生成溯源树,研究论文发展脉络
Chat Paper
正在生成论文摘要