Localize, Retrieve and Fuse: A Generalized Framework for Free-Form Question Answering over Tables
Findings of the Association for Computational Linguistics: IJCNLP-AACL 2023 (Findings)(2023)
摘要
Question answering on tabular data (TableQA), which aims at generating answers to questions grounded on a given table, has attracted increasing attention in recent years. Existing work tends to generate factual short-form answers by extracting information from one or a few table cells without reasoning over selected table cells. However, the free-form TableQA, requiring a more complex relevant table cell selection strategy and the complex integration and inference of separate pieces of information, has been under-explored. To this end, this paper proposes a generalized three-stage approach: Table-to-Graph conversion and cell localizing, external knowledge retrieval and table-text fusion (called TAG-QA), addressing the challenge of inferring long free-form answer for generative TableQA. In particular, TAG-QA (1) locates relevant table cells using a graph neural network to gather intersecting cells between relevant rows and columns; (2) leverages external knowledge from Wikipedia and (3) generates answers by integrating both tabular data and natural linguistic information. Experiments with a human evaluation demonstrate that TAG-QA is capable of generating more faithful and coherent sentence when compared with several state-of-the-art baselines. Especially, TAG-QA outperforms the strong pipeline-based baseline TAPAS by 17% and 14%, in terms of BLEU-4 and PARENT F-score, respectively. Moreover, TAG-QA outperforms end-to-end model T5 by 16% and 12% on BLEU-4 and PARENT F-score.
更多查看译文
AI 理解论文
溯源树
样例
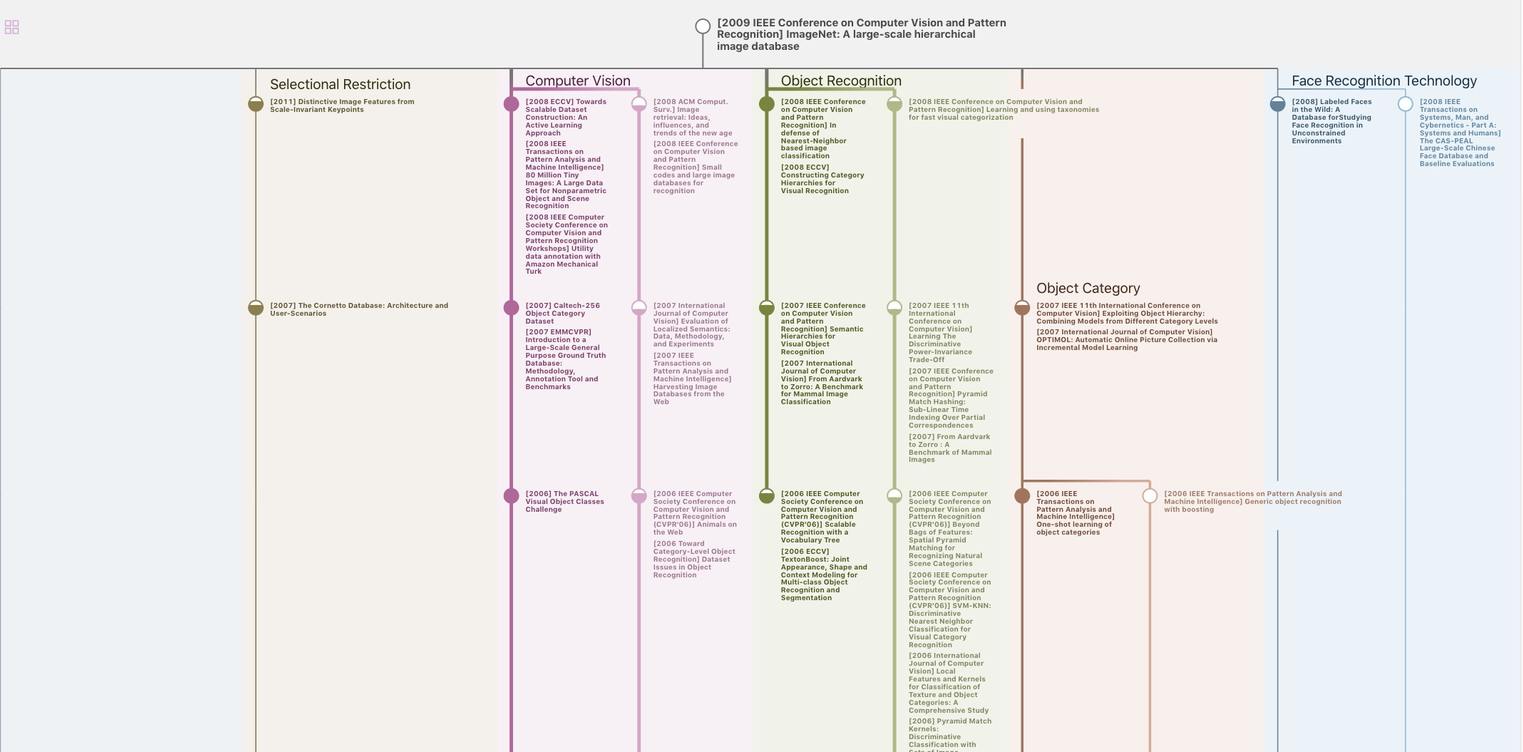
生成溯源树,研究论文发展脉络
Chat Paper
正在生成论文摘要