Def-Grasp: A Robot Grasping Detection Method for Deformable Objects Without Force Sensor
NEURAL PROCESSING LETTERS(2023)
摘要
Learning-based robot grasping of deformable objects without force sensors is a challenging task. This paper proposes a method without force sensors to predict deformation properties and the grasp poses of objects to solve the problem of deformable objects damaged by the gripper. Firstly, the deformation representation of the object is defined according to the deformation ratio of the object grasped. Then, the lightweight dataset with object deformation properties is produced based on the truncated signed distance function technique. The dataset can render images from any perspective for the training network. Subsequently, we investigate the synergies between grasp pose and gripper closure and use the above lightweight dataset to train deep network. The resulting network, Def-Grasp, can predict object grasp poses and deformation properties, the gripper closure is calculated using the network predictions. Finally, the expected experimental results show that the deformable objects in the cluttered scene are not damaged by the gripper during the 6DoF grasping process.
更多查看译文
关键词
Robot grasp,Deformable object,Deep learning,Pose estimation
AI 理解论文
溯源树
样例
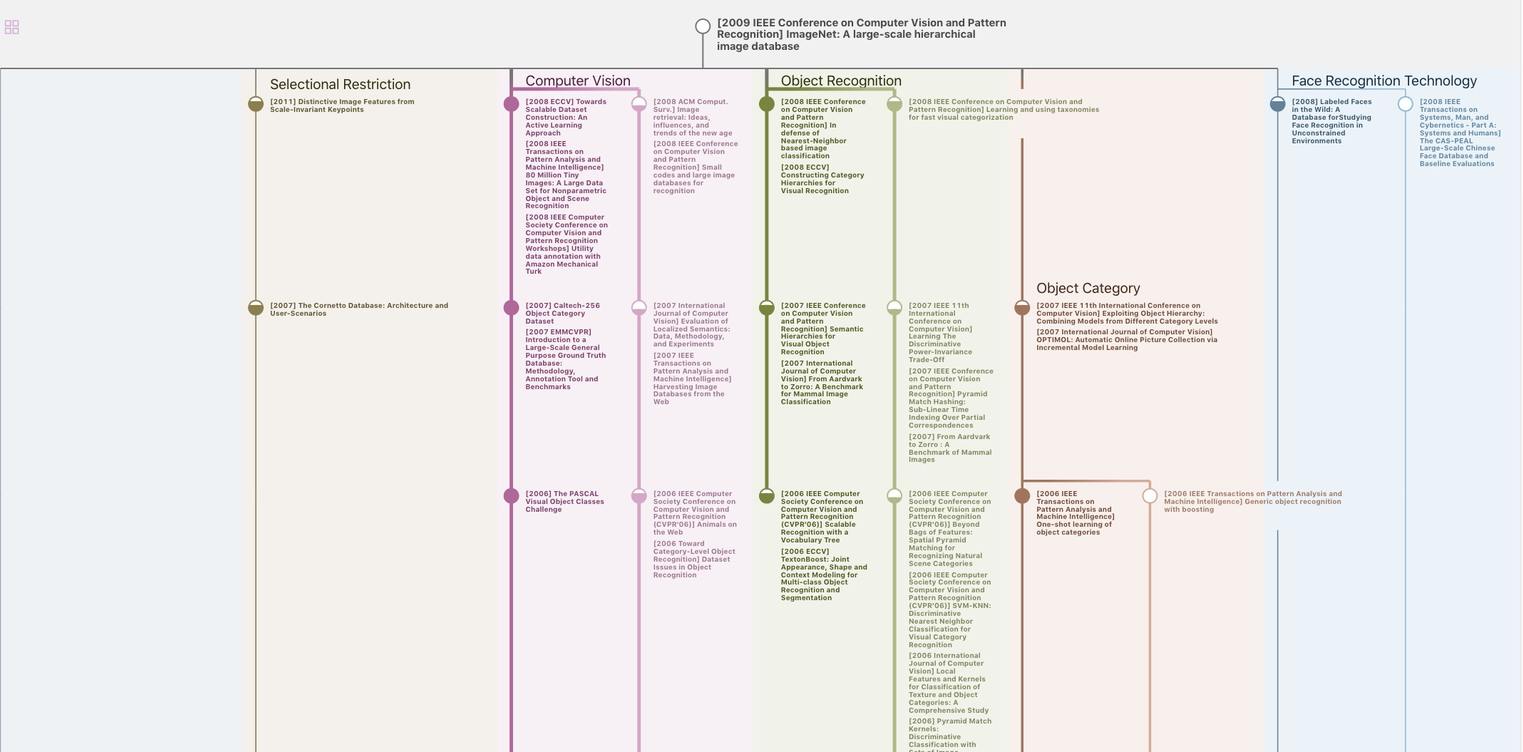
生成溯源树,研究论文发展脉络
Chat Paper
正在生成论文摘要