Enabling Scalability in the Cloud for Scientific Workflows: An Earth Science Use Case
2023 IEEE 16th International Conference on Cloud Computing (CLOUD)(2023)
摘要
Scientific discovery increasingly relies on interoperable, multimodular workflows generating intermediate data. The complexity of managing intermediate data may cause performance losses or unexpected costs. This paper defines an approach to composing these scientific workflows on cloud services, focusing on workflow data orchestration, management, and scalability. We demonstrate the effectiveness of our approach with the SOMOSPIE scientific workflow that deploys machine learning (ML) models to predict high-resolution soil moisture using an HPC service (LSF) and an open-source cloud-native service (K8s) and object storage. Our approach enables scientists to scale from coarse-grained to fine-grained resolution and from a small to a larger region of interest. Using our empirical observations, we generate a cost model for the execution of workflows with hidden intermediate data on cloud services.
更多查看译文
关键词
Intermediate data,Workflows,Cloud service,High-performance computing,Object storage,Cost model
AI 理解论文
溯源树
样例
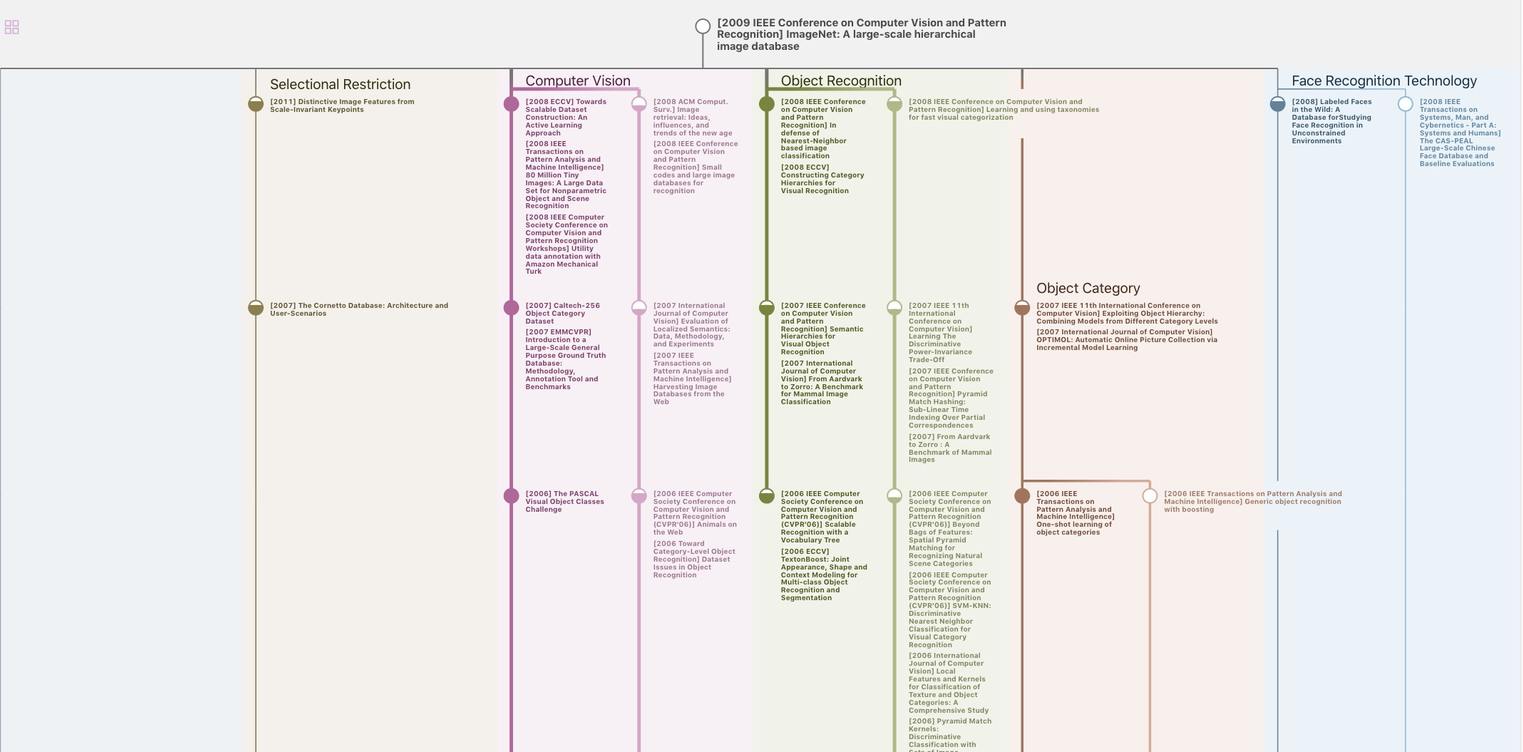
生成溯源树,研究论文发展脉络
Chat Paper
正在生成论文摘要