COMPOSER: Scalable and Robust Modular Policies for Snake Robots
ICRA 2024(2024)
摘要
Snake robots have showcased remarkable compliance and adaptability in their interaction with environments as their natural counterparts. While their hyper-redundant and high-dimensional characteristics add to this adaptability, they also pose great challenges to robot control. Instead of perceiving the hyper-redundancy and flexibility of snake robots as mere challenges, there lies an unexplored potential in leveraging these traits to enhance robustness and generalizability at the control policy level. We seek to develop a control policy that effectively breaks down the high dimensionality of snake robots while harnessing their redundancy. In this work, we consider the snake robot as a modular robot and formulate the control of the snake robot as a cooperative Multi-Agent Reinforcement Learning (MARL) problem. Each segment of the snake robot is an agent, using its local observation to independently determine its actions. Specifically, we incorporate a self-attention mechanism to enhance the cooperative behavior between agents. A high-level imagination policy is trained to provide additional rewards to guide the low-level control policy. We validate the proposed method COMPOSER with five snake robot tasks, including goal reaching, wall climbing, shape formation, tube crossing, and block pushing. COMPOSER achieves the highest success rate across all tasks when compared to a centralized baseline and four modular policy baselines. Additionally, we show enhanced robustness against module corruption and significantly superior zero-shot generalizability in our proposed method. The videos of this work are available on our project page: https://sites.google.com/view/composer-snake/.
更多查看译文
关键词
Bioinspired Robot Learning,Reinforcement Learning,Modeling, Control, and Learning for Soft Robots
AI 理解论文
溯源树
样例
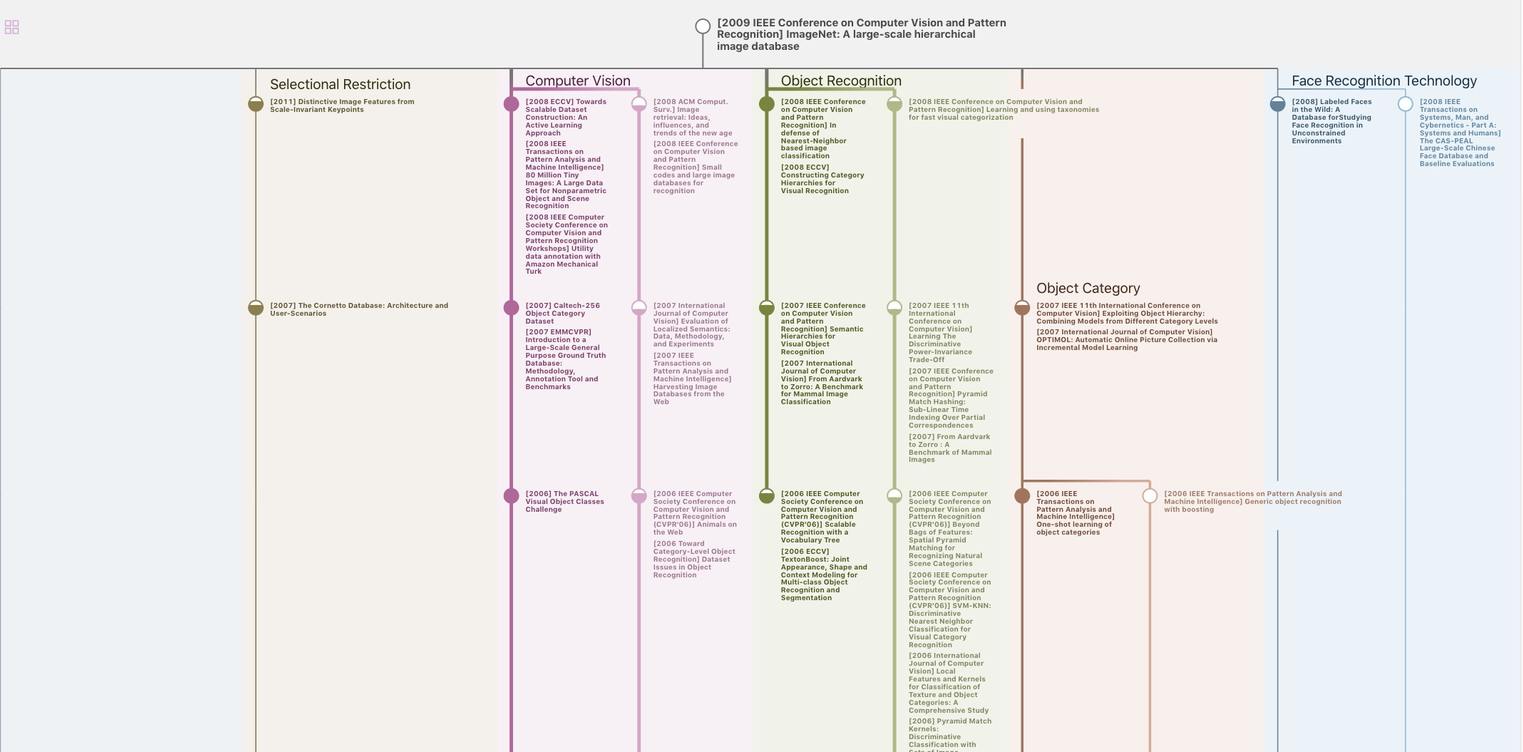
生成溯源树,研究论文发展脉络
Chat Paper
正在生成论文摘要