Closing the Curious Case of Neural Text Degeneration
ICLR 2024(2023)
摘要
Despite their ubiquity in language generation, it remains unknown why truncation sampling heuristics like nucleus sampling are so effective. We provide a theoretical explanation for the effectiveness of the truncation sampling by proving that truncation methods that discard tokens below some probability threshold (the most common type of truncation) can guarantee that all sampled tokens have nonzero true probability. However, thresholds are a coarse heuristic, and necessarily discard some tokens with nonzero true probability as well. In pursuit of a more precise sampling strategy, we show that we can leverage a known source of model errors, the softmax bottleneck, to prove that certain tokens have nonzero true probability, without relying on a threshold. Based on our findings, we develop an experimental truncation strategy and the present pilot studies demonstrating the promise of this type of algorithm. Our evaluations show that our method outperforms its threshold-based counterparts under automatic and human evaluation metrics for low-entropy (i.e., close to greedy) open-ended text generation. Our theoretical findings and pilot experiments provide both insight into why truncation sampling works, and make progress toward more expressive sampling algorithms that better surface the generative capabilities of large language models.
更多查看译文
关键词
softmax,bottleneck,truncation,sampling,nucleus,top-k,theory,linear programming,linear algebra,decoding,generation,autoregressive,language model,NLP,open-ended generation
AI 理解论文
溯源树
样例
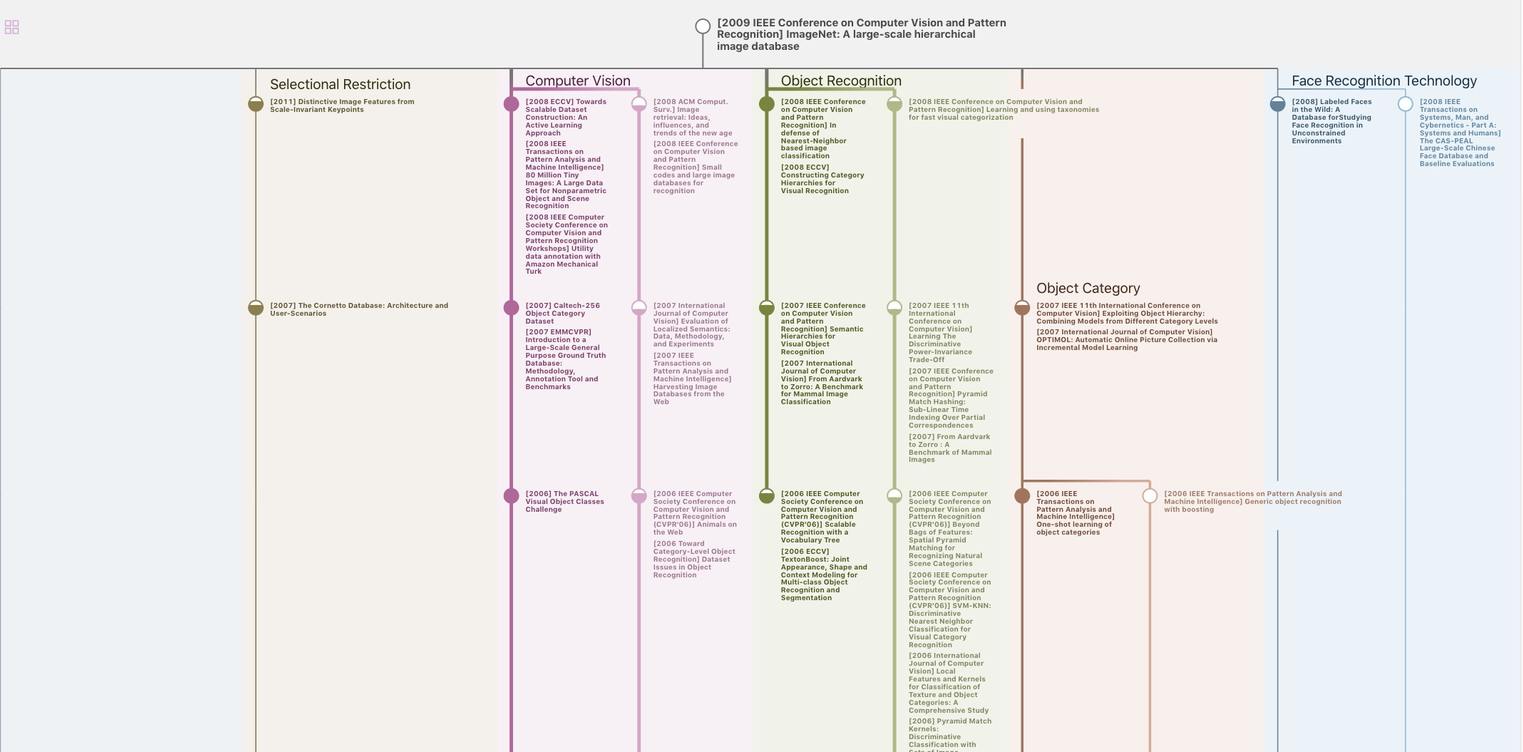
生成溯源树,研究论文发展脉络
Chat Paper
正在生成论文摘要