Characterizing the Informativity of Level II Book Data for High Frequency Trading.
CCTA(2023)
摘要
High-Frequency Trading (HFT) algorithms are automated feedback systems that interact with markets to maximize investment returns. These systems can process varying resolutions of market information at any given time, with Level I information providing basic equity data—primarily its price—and Level II information offering the complete order book for that equity at a specific moment. This paper presents a study on the application of Recurrent Neural Network (RNN) models to predict the spread of the DOW Industrial 30 index traded on NASDAQ, using both Level I and Level II data as inputs.The results suggest that the use of raw Level II data, without preprocessing, does not enhance spread prediction. This finding implies that HFT algorithms should not directly utilize raw Level II information; instead, computational resources should be allocated towards transforming Level II data into a less noisy signal if it is to improve trading performance. Alternatively, when computational resources are limited, HFT algorithms may benefit from omitting Level II data.
更多查看译文
关键词
High Frequency Trading,Data Informativity,Recurrent Neural Network,Spread Prediction,Limit Order Book
AI 理解论文
溯源树
样例
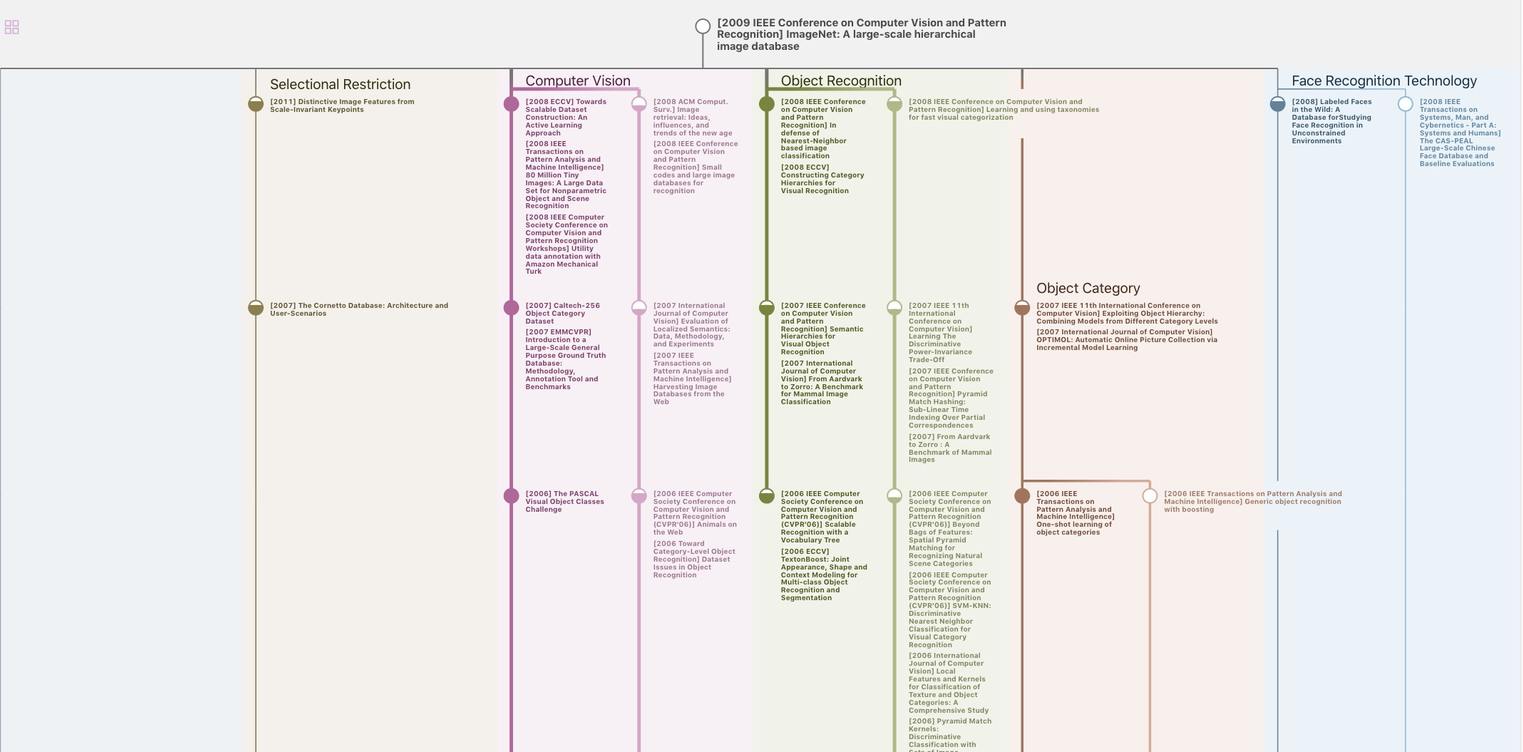
生成溯源树,研究论文发展脉络
Chat Paper
正在生成论文摘要