Formally Specifying the High-Level Behavior of LLM-Based Agents
CoRR(2023)
摘要
Autonomous, goal-driven agents powered by LLMs have recently emerged as
promising tools for solving challenging problems without the need for
task-specific finetuned models that can be expensive to procure. Currently, the
design and implementation of such agents is ad hoc, as the wide variety of
tasks that LLM-based agents may be applied to naturally means there can be no
one-size-fits-all approach to agent design. In this work we aim to alleviate
the difficulty of designing and implementing new agents by proposing a
minimalistic generation framework that simplifies the process of building
agents. The framework we introduce allows the user to define desired agent
behaviors in a high-level, declarative specification that is then used to
construct a decoding monitor which guarantees the LLM will produce an output
exhibiting the desired behavior. Our declarative approach, in which the
behavior is described without concern for how it should be implemented or
enforced, enables rapid design, implementation, and experimentation with
different LLM-based agents. We demonstrate how the proposed framework can be
used to implement recent LLM-based agents (e.g., ReACT), and show how the
flexibility of our approach can be leveraged to define a new agent with more
complex behavior, the Plan-Act-Summarize-Solve (PASS) agent. Lastly, we
demonstrate that our method outperforms other agents on multiple popular
reasoning-centric question-answering benchmarks.
更多查看译文
关键词
agents,behavior,high-level high-level,llm-based
AI 理解论文
溯源树
样例
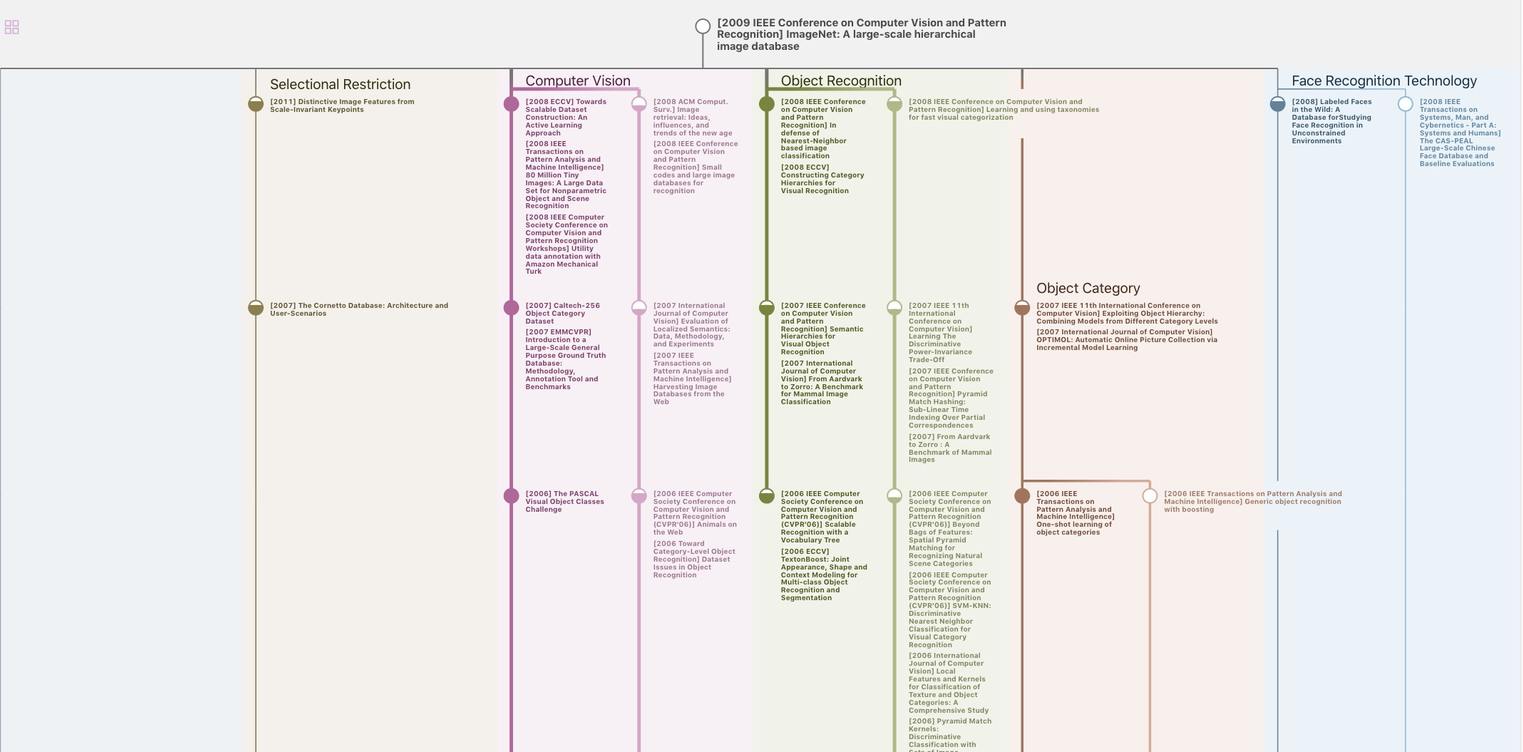
生成溯源树,研究论文发展脉络
Chat Paper
正在生成论文摘要