Calibrating Likelihoods towards Consistency in Summarization Models
CoRR(2023)
摘要
Despite the recent advances in abstractive text summarization, current summarization models still suffer from generating factually inconsistent summaries, reducing their utility for real-world application. We argue that the main reason for such behavior is that the summarization models trained with maximum likelihood objective assign high probability to plausible sequences given the context, but they often do not accurately rank sequences by their consistency. In this work, we solve this problem by calibrating the likelihood of model generated sequences to better align with a consistency metric measured by natural language inference (NLI) models. The human evaluation study and automatic metrics show that the calibrated models generate more consistent and higher-quality summaries. We also show that the models trained using our method return probabilities that are better aligned with the NLI scores, which significantly increase reliability of summarization models.
更多查看译文
关键词
likelihoods,consistency,models
AI 理解论文
溯源树
样例
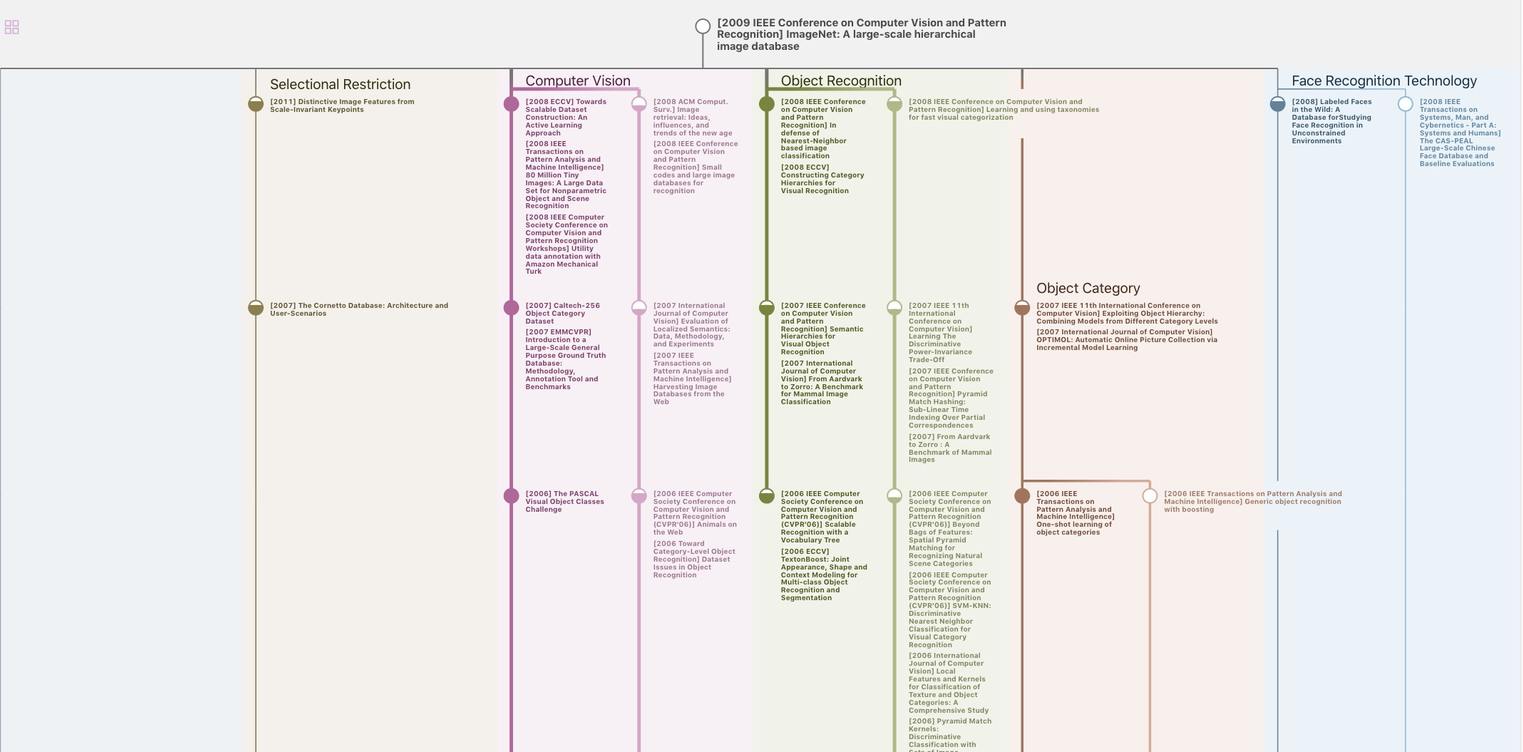
生成溯源树,研究论文发展脉络
Chat Paper
正在生成论文摘要