Contextual Gaps in Machine Learning for Mental Illness Prediction: The Case of Diagnostic Disclosures.
Proceedings of the ACM on Human-Computer Interaction(2023)
摘要
Getting training data for machine learning (ML) prediction of mental illness on social media data is labor intensive. To work around this, ML teams will extrapolate proxy signals, or alternative signs from data to evaluate illness status and create training datasets. However, these signals' validity has not been determined, whether signals align with important contextual factors, and how proxy quality impacts downstream model integrity. We use ML and qualitative methods to evaluate whether a popular proxy signal, diagnostic self-disclosure, produces a conceptually sound ML model of mental illness. Our findings identify major conceptual errors only seen through a qualitative investigation -- training data built from diagnostic disclosures encodes a narrow vision of diagnosis experiences that propagates into paradoxes in the downstream ML model. This gap is obscured by strong performance of the ML classifier (F1 = 0.91). We discuss the implications of conceptual gaps in creating training data for human-centered models, and make suggestions for improving research methods.
更多查看译文
关键词
mental illness prediction,diagnostic disclosures,mental illness,contextual gaps,machine learning
AI 理解论文
溯源树
样例
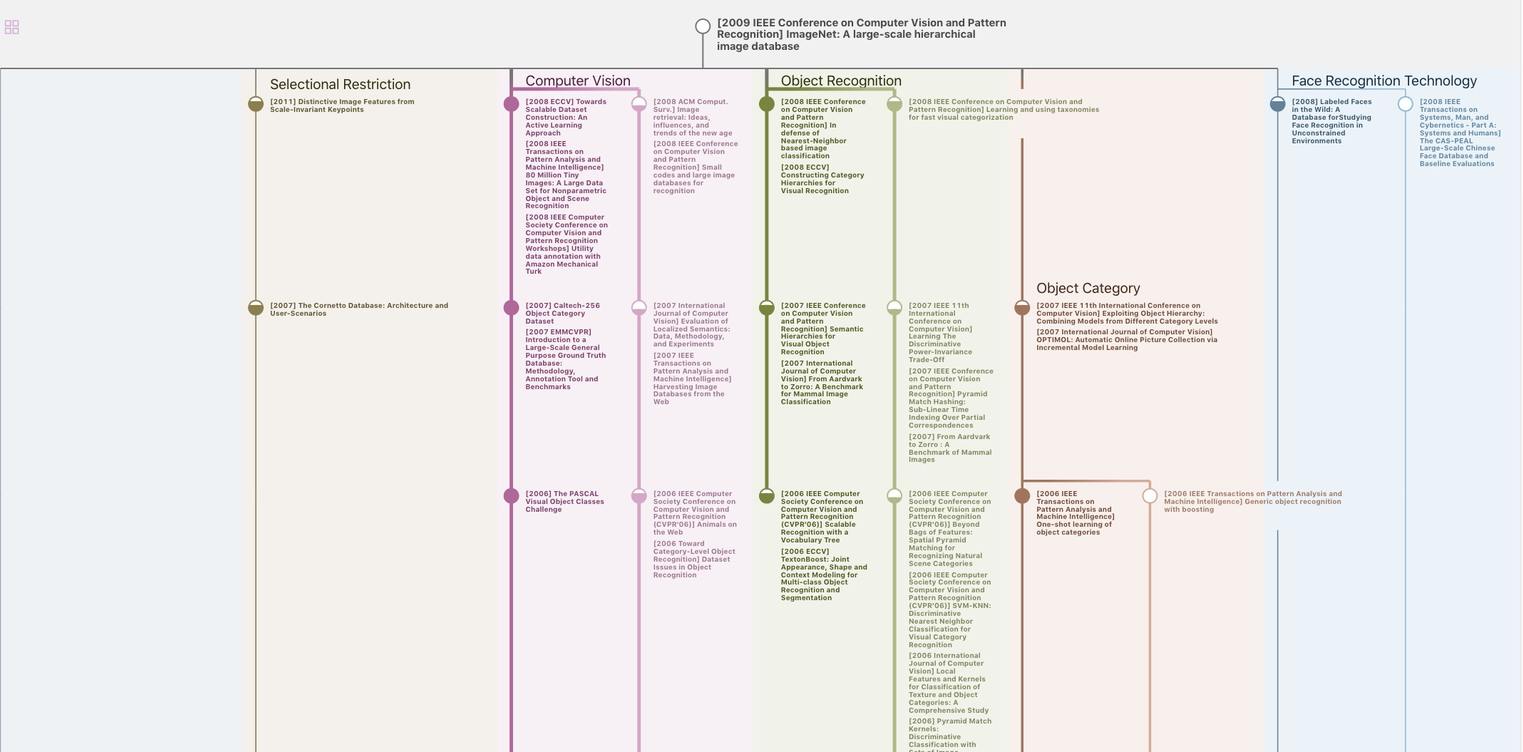
生成溯源树,研究论文发展脉络
Chat Paper
正在生成论文摘要