CoSaR: Combating Label Noise Using Collaborative Sample Selection and Adversarial Regularization
PROCEEDINGS OF THE 32ND ACM INTERNATIONAL CONFERENCE ON INFORMATION AND KNOWLEDGE MANAGEMENT, CIKM 2023(2023)
摘要
Learning with noisy labels is nontrivial for deep learning models. Sample selection is a widely investigated research topic for handling noisy labels. However, most existing methods face challenges such as imprecise selection, a lack of global selection capabilities, and the need for tedious hyperparameter tuning. In this paper, we propose CoSaR (Collaborative Selection and adversarial Regularization), a twin-networks based model that performs globally adaptive sample selection to tackle label noise. Specifically, the collaborative selection estimates the average distribution distances between predictions and generation labels through the collaboration of two networks to address the bias of the average distribution distances and the manual tuning of hyperparameters. Adversarial regularization is integrated into CoSaR to restrict the network's tendency to fit and memorize noisy labels, thereby enhancing its collaborative selection capability. In addition, we employ a label smoothing regularization and two types of data augmentation to enhance the robustness of the model further. Extensive experiments on both synthetic and real-world noisy datasets demonstrate that the proposed model outperforms baseline methods remarkably, with an accuracy improvement ranging between +0.56% and +15.14%.
更多查看译文
关键词
Label-noise learning,Collaborative selection,Adversarial regularization
AI 理解论文
溯源树
样例
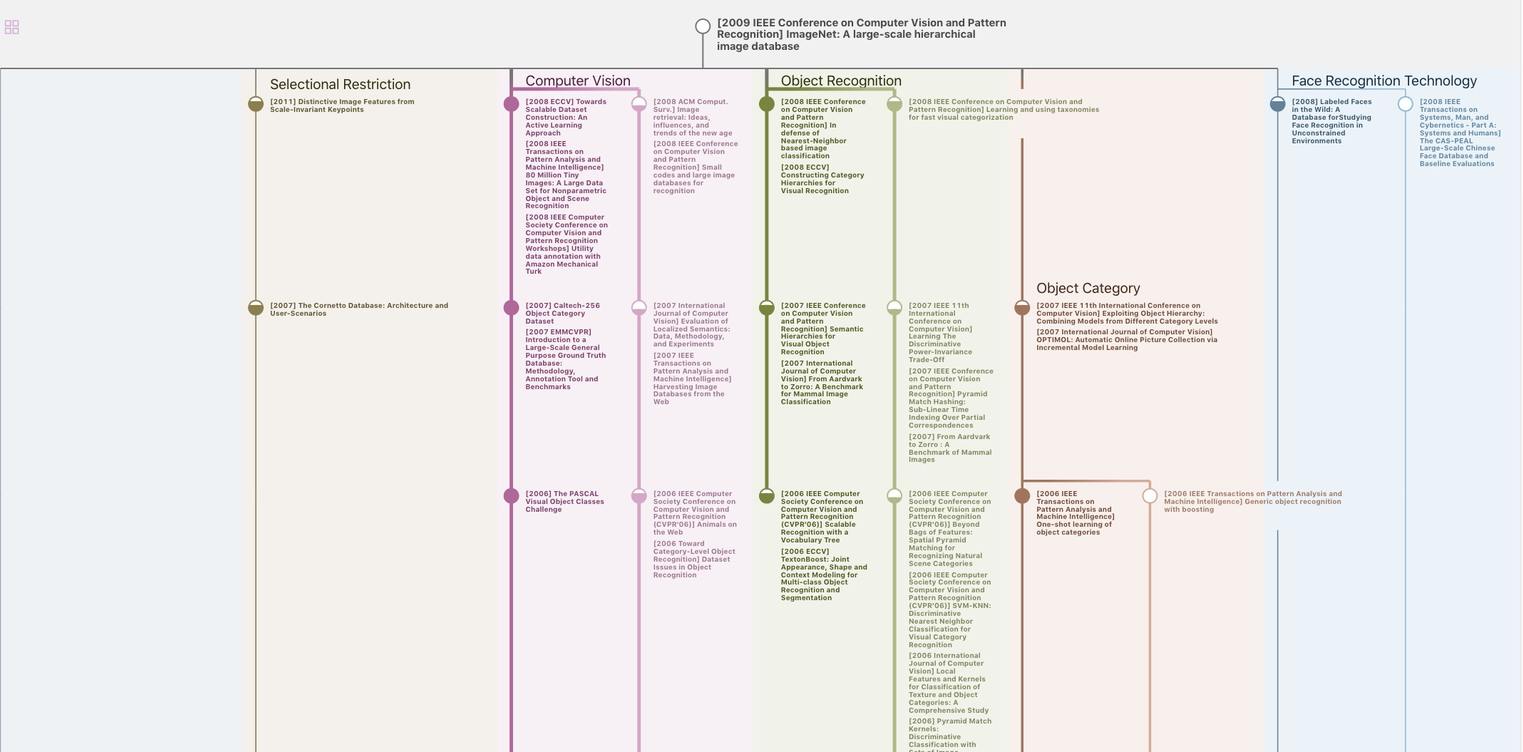
生成溯源树,研究论文发展脉络
Chat Paper
正在生成论文摘要