Understanding the Inner-workings of Language Models Through Representation Dissimilarity.
CoRR(2023)
摘要
As language models are applied to an increasing number of real-world applications, understanding their inner workings has become an important issue in model trust, interpretability, and transparency. In this work we show that representation dissimilarity measures, which are functions that measure the extent to which two model's internal representations differ, can be a valuable tool for gaining insight into the mechanics of language models. Among our insights are: (i) an apparent asymmetry in the internal representations of model using SoLU and GeLU activation functions, (ii) evidence that dissimilarity measures can identify and locate generalization properties of models that are invisible via in-distribution test set performance, and (iii) new evaluations of how language model features vary as width and depth are increased. Our results suggest that dissimilarity measures are a promising set of tools for shedding light on the inner workings of language models.
更多查看译文
关键词
language models,representation dissimilarity
AI 理解论文
溯源树
样例
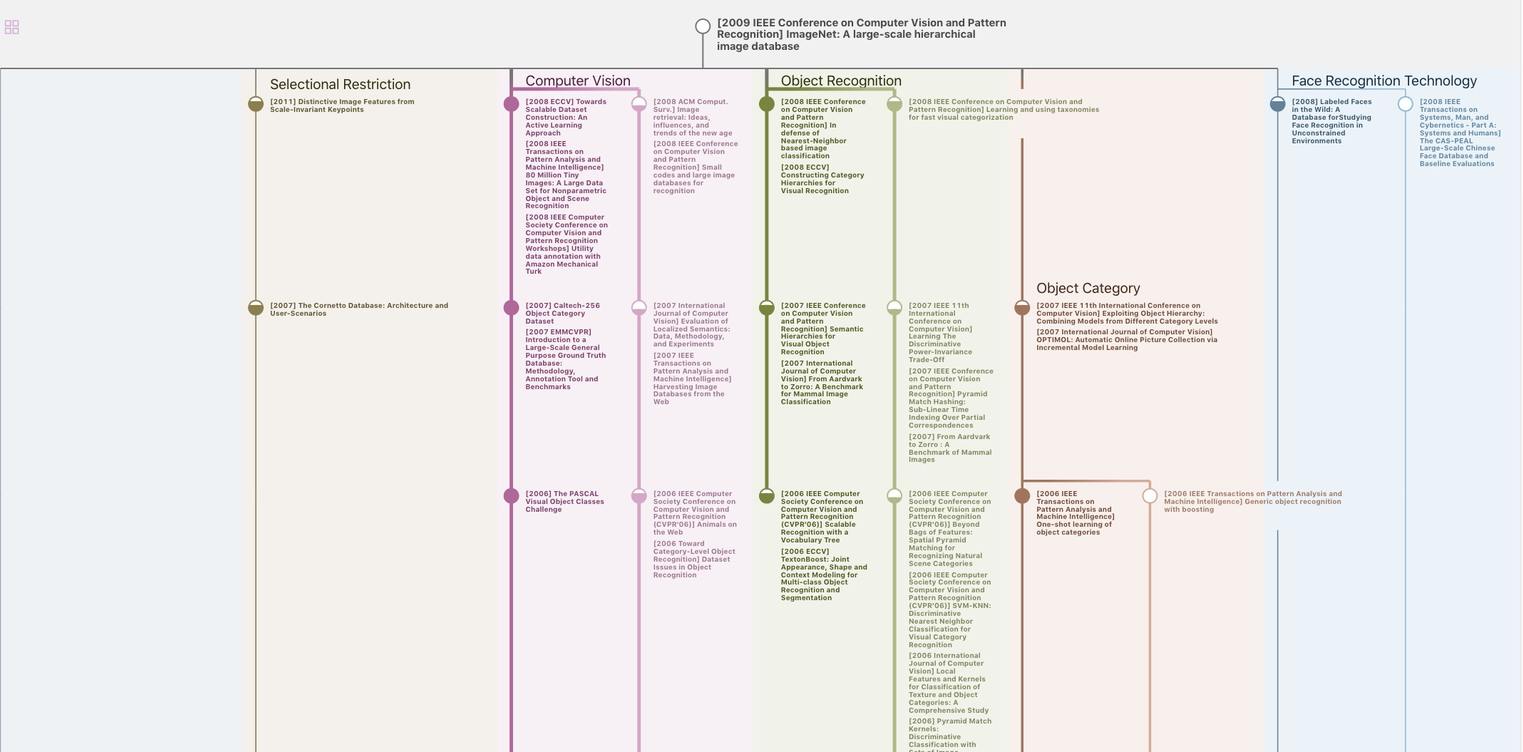
生成溯源树,研究论文发展脉络
Chat Paper
正在生成论文摘要