STDM-transformer: Space-time dual multi-scale transformer network for skeleton-based action recognition
NEUROCOMPUTING(2024)
摘要
Transformer-based methods have currently demonstrated impressive results in the field of skeleton-based action recognition. Nevertheless, how to effectively model multi-scale features with transformers remains a challenging problem, which is crucial to distinguish various actions. In this paper, we propose a Space- time Dual Multi-scale transformer (STDM-transformer) to explore the multi-scale collaborative representation employing both fine and coarse scale motion information. In contrast to existing approaches which typically propagate information between scales in a single fusion manner, our Space-time Dual Multi-scale method stratifies the space-time multi-scale into dual levels. One level is to construct fine-grained local motion interactions. In detail, the space-time multi-scale partition strategy and the novel intra-inter space-time transformer module are proposed to extract and aggregate the feature in part scale and body scale, respectively. The other is aimed at modeling coarse-grained global motion contexts, in which the layer-wise multi -scale progressive fusion strategy is designed. Extensive experimental results demonstrate that the proposed STDM-transformer achieves the SOTA performance on large-scale datasets.
更多查看译文
关键词
Skeleton-based action recognition,Transformer,Dual multi-scale network
AI 理解论文
溯源树
样例
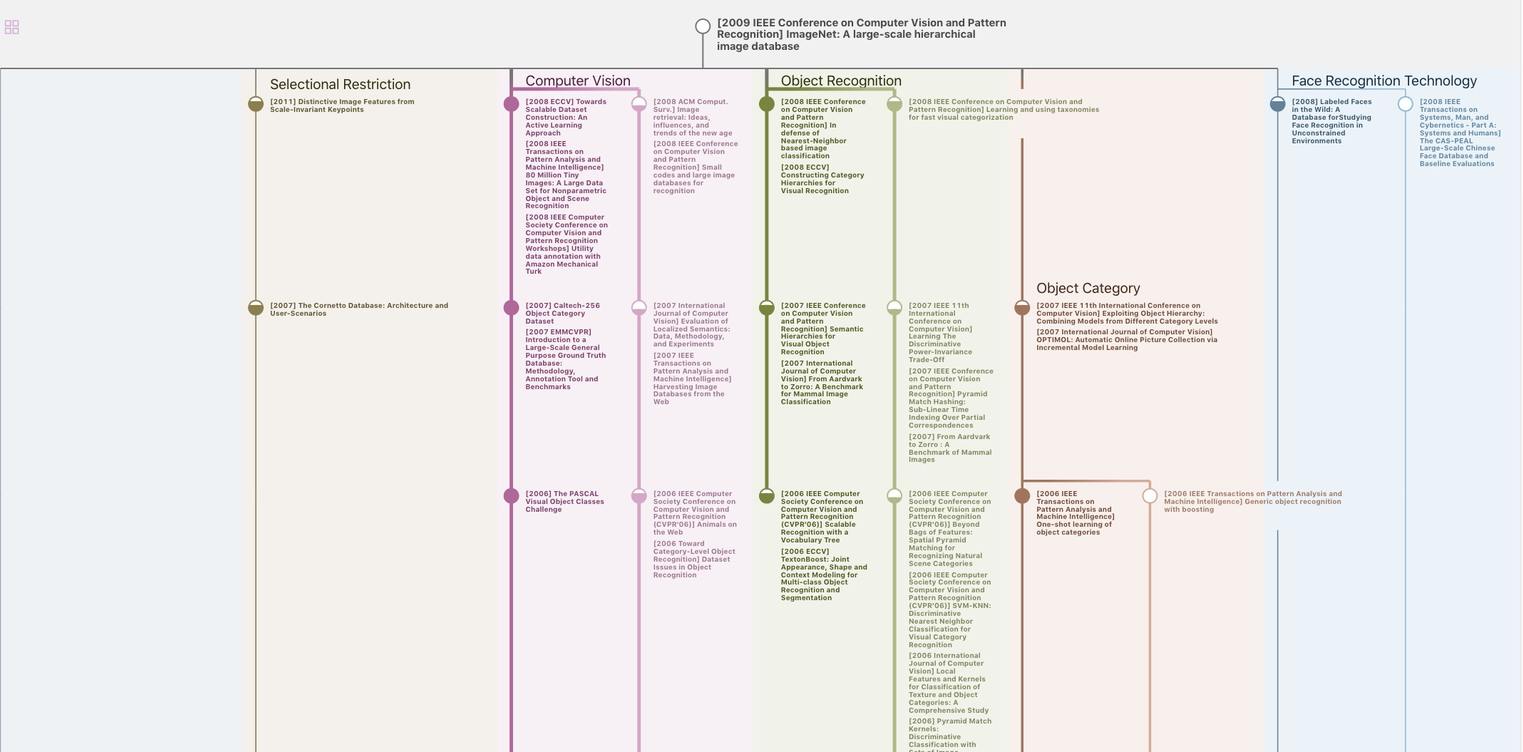
生成溯源树,研究论文发展脉络
Chat Paper
正在生成论文摘要