FHDnn
Proceedings of the 59th ACM/IEEE Design Automation Conference(2022)
摘要
The advent of IoT and advances in edge computing inspired federated learning, a distributed algorithm to enable on device learning. Transmission costs, unreliable networks and limited compute power all of which are typical characteristics of IoT networks pose a severe bottleneck for federated learning. In this work we propose FHDnn, a synergetic federated learning framework that combines the salient aspects of CNNs and Hyperdimensional Computing. FHDnn performs hyperdimensional learning on features extracted from a self-supervised contrastive learning framework to accelerate training, lower communication costs, and increase robustness to network errors by avoiding the transmission of the CNN and training only the hyperdimensional component. Compared to CNNs, we show through experiments that FHDnn reduces communication costs by 66X, local client compute and energy consumption by 1.5 - 6X, while being highly robust to network errors with minimal loss in accuracy.
更多查看译文
AI 理解论文
溯源树
样例
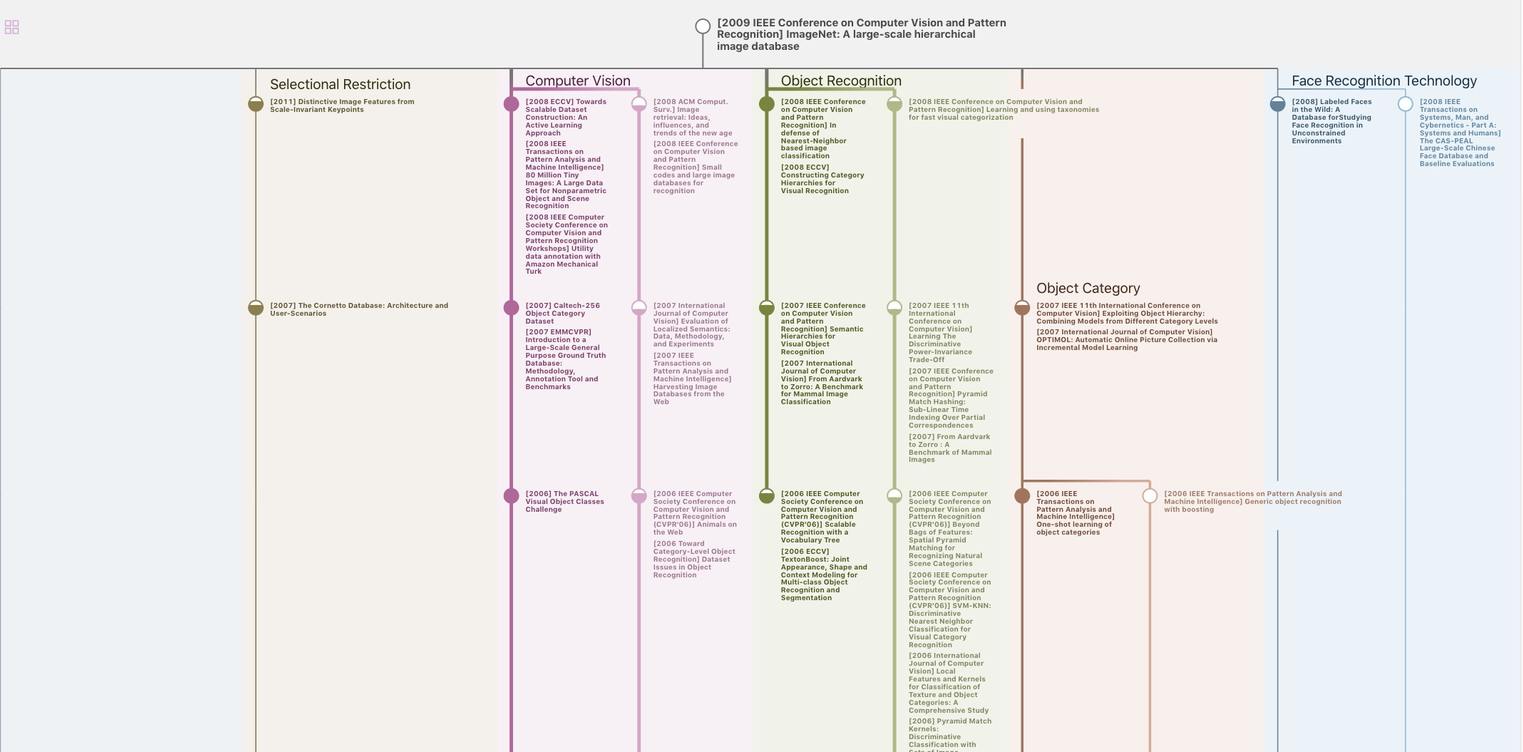
生成溯源树,研究论文发展脉络
Chat Paper
正在生成论文摘要