Dropping Pathways Towards Deep Multi-View Graph Subspace Clustering Networks
Proceedings of the 31st ACM International Conference on Multimedia(2023)
摘要
Multi-view graph clustering aims to leverage different views to obtain consistent information and improve clustering performance by sharing the graph structure. Existing multi-view graph clustering algorithms generally adopt a single-pathway network reconstruction and consistent feature extraction, building on top of auto-encoders and graph convolutional networks (GCN). Despite their promising results, these single-pathway methods may ignore the significant complementary information between different layers and the rich multi-level context inside. On the other hand, GCN usually employs a shallow network structure (2-3 layers) due to the over-smoothing with the increase of network depth, while few multi-view graph clustering methods explore the performance of deep networks. In this work, we propose a novel Dropping Pathways strategy toward building a deep Multi-view Graph Subspace Clustering network, namely DPMGSC, to fully exploit the deep and multi-level graph network representations. The proposed method implements a multi-pathway self-expressive network to capture pairwise affinities of graph nodes among multiple views. Moreover, we empirically study the impact of a series of dropping methods on deep multi-pathway networks. Extensive experiments demonstrate the effectiveness of the proposed DPMGSC compared with its deep counterpart and state-of-the-art methods.
更多查看译文
关键词
Multi-view clustering,multi-pathway networks,graph convolutional networks
AI 理解论文
溯源树
样例
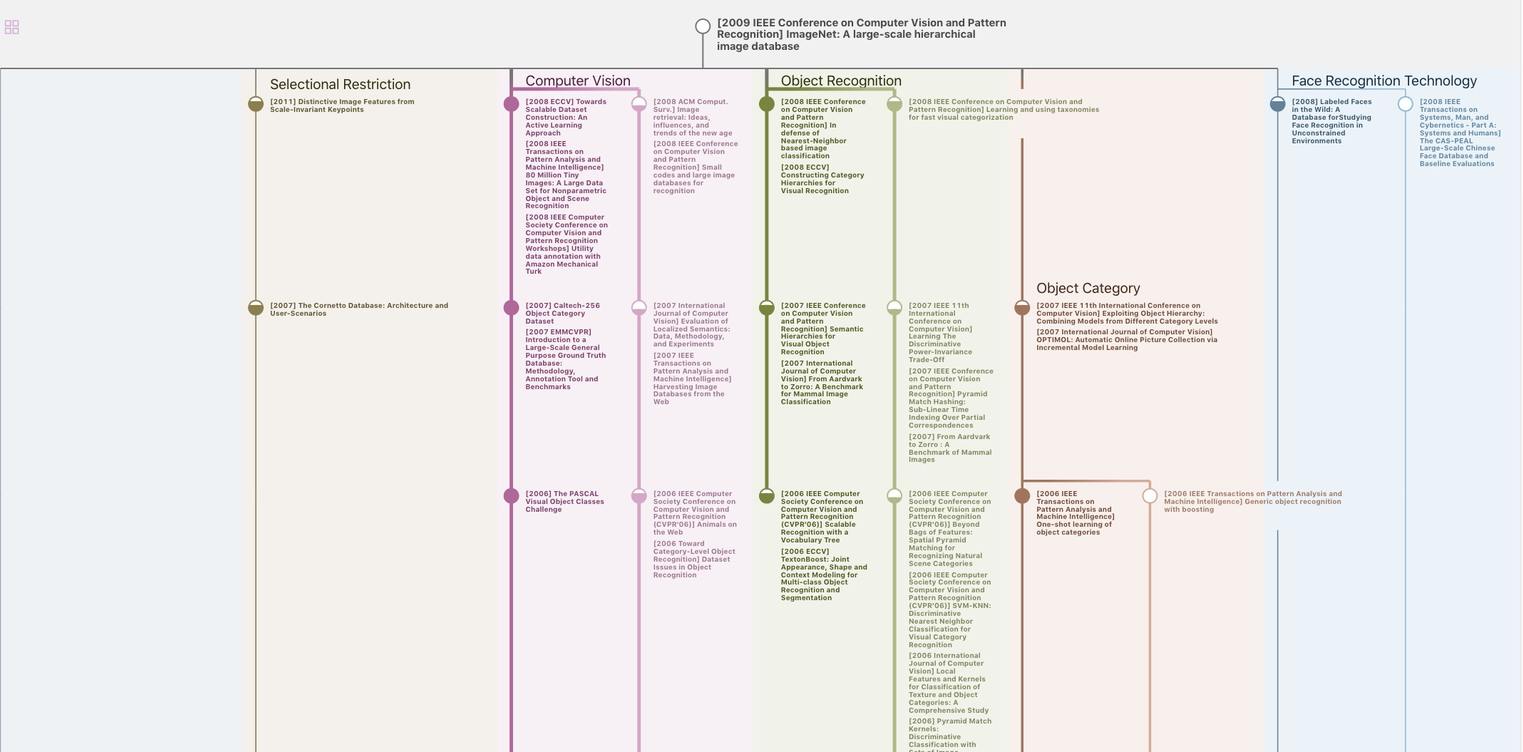
生成溯源树,研究论文发展脉络
Chat Paper
正在生成论文摘要