CPLFormer: Cross-scale Prototype Learning Transformer for Image Snow Removal
MM '23: Proceedings of the 31st ACM International Conference on Multimedia(2023)
摘要
Removing snow from a single image poses a significant challenge within the image restoration domain, as snowfall's effects are in various scales and forms. Existing methods have tried to tackle this issue by using multi-scale approaches, but their reliance on targeted design for handling each single-scale feature has resulted in unsatisfactory performance. This is primarily due to a lack of cross-scale knowledge, making it difficult to effectively handle degradations. To this end, we propose a novel approach, CPLFormer, which uses snow prototypes to own comprehensive clean scene understanding through learning from cross-scale features, outperforming convolutional network and vanilla transformer-based solutions. CPLFormer has several advantages: firstly, learnable snow prototypes learn global context information from multiple scales to uncover hidden clean cues; secondly, prototypes can propagate cross-scale information to each patch through cross-attention to assist with clean patch reconstruction; thirdly, CPLFormer surpasses advanced state-of-the-art desnowing networks and the prevalent universal image restoration transformers on six synthetic and real-world benchmark tests.
更多查看译文
AI 理解论文
溯源树
样例
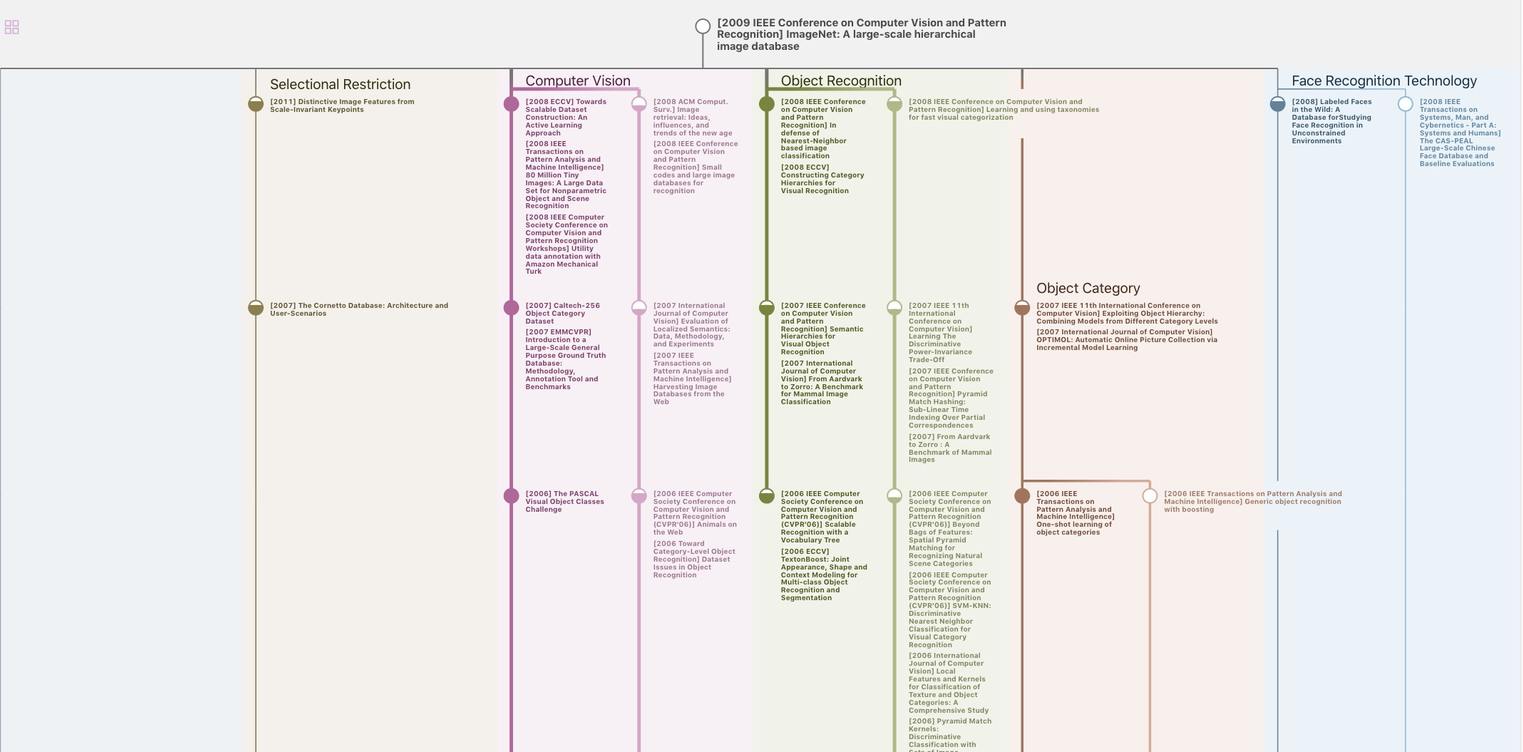
生成溯源树,研究论文发展脉络
Chat Paper
正在生成论文摘要