Collaborative Learning of Diverse Experts for Source-free Universal Domain Adaptation
MM '23: Proceedings of the 31st ACM International Conference on Multimedia(2023)
摘要
Source-free universal domain adaptation (SFUniDA) is a challenging yet practical problem that adapts the source model to the target domain in the presence of distribution and category shifts without accessing source domain data. Most existing methods are developed based on a single-expert target model for both known- and unknown-class data training, such that the known- and unknown-class data in the target domain may not be separated well from each other. To address this issue, we propose a novel Cobllaborative Learning of Diverse Experts (CoDE) method for SFUniDA. In our method, unknown-class compatible source model training is designed to reserve space for the potential target unknown-class data. Two diverse experts are learned to better recognize the target known- and unknown-class data respectively by the specialized entropy discrimination. We improve the transferability of both experts by collaboratively correcting the possible misclassification errors with consistency and diversity learning. The final prediction with high confidence is obtained by gating the diverse experts based on soft neighbor density. Extensive experiments on four publicly available benchmarks demonstrate the superiority of our method compared to the state of the art.
更多查看译文
AI 理解论文
溯源树
样例
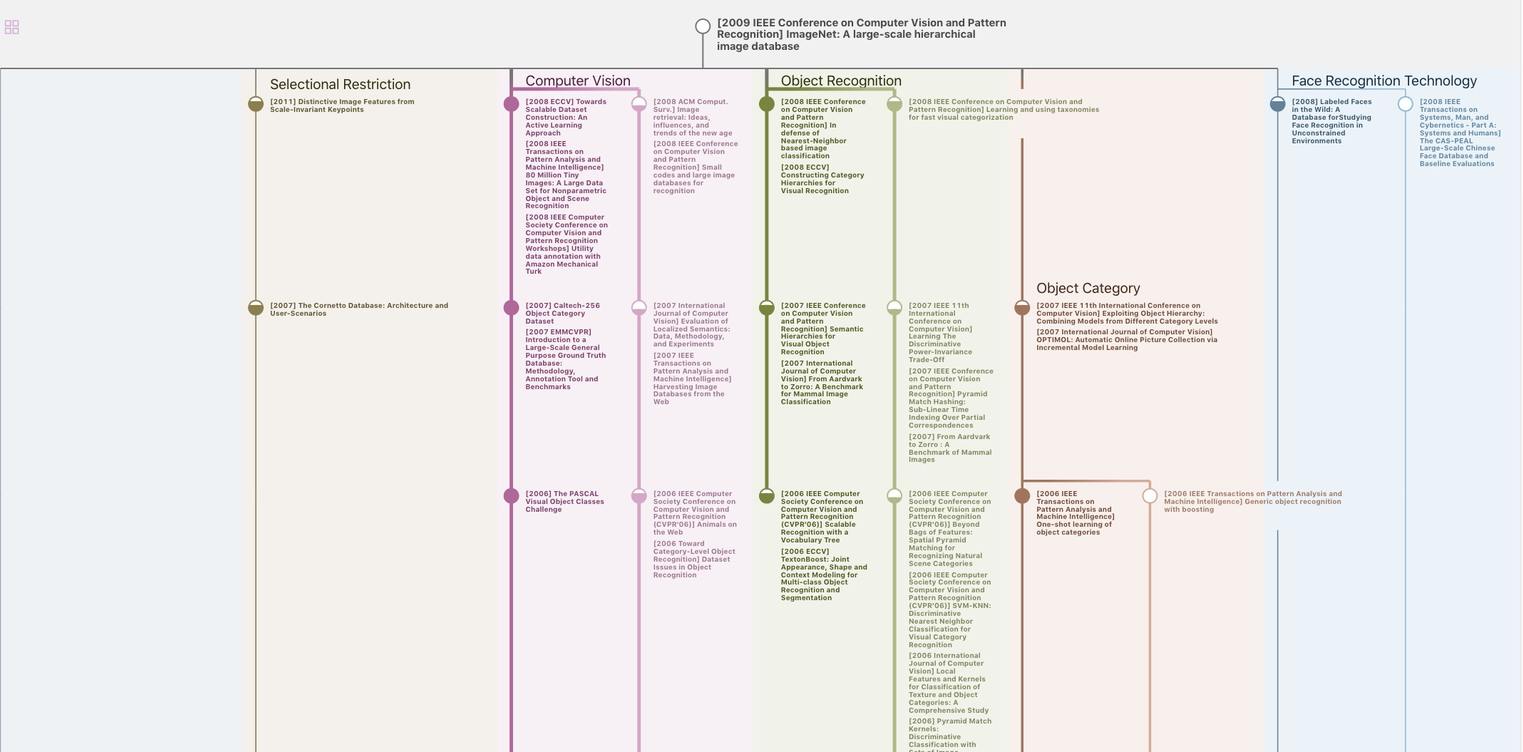
生成溯源树,研究论文发展脉络
Chat Paper
正在生成论文摘要