Better Together: Data-Free Multi-Student Coevolved Distillation
KNOWLEDGE-BASED SYSTEMS(2024)
摘要
Data-Free Knowledge Distillation (DFKD) aims to craft a customized student model from a pre-trained teacher model by synthesizing surrogate training images. However, a seldom-investigated scenario is to distill the knowledge to multiple heterogeneous students simultaneously. In this paper, we aim to study how to improve the performance by coevolving peer students, termed Data-Free Multi-Student Coevolved Distillation (DF-MSCD). Based on previous DFKD methods, we advance DF-MSCD by improving the data quality from the perspective of synthesizing unbiased, informative and diverse surrogate samples: 1) Unbiased. The disconnection of image synthesis among different timestamps during DFKD will lead to an unnoticed class imbalance problem. To tackle this problem, we reform the prior art into an unbiased variant by bridging the label distribution of the synthesized data among different timestamps. 2) Informative. Different from single-student DFKD, we encourage the interactions not only between teacher-student pairs, but also within peer students, driving a more comprehensive knowledge distillation. To this end, we devise a novel Inter-Student Adversarial Learning method to coevolve peer students with mutual benefits. 3) Diverse. To further promote Inter-Student Adversarial Learning, we develop Mixture-of-Generators, in which multiple generators are optimized to synthesize different yet complementary samples by playing min-max games with multiple students. Experiments are conducted to validate the effectiveness and efficiency of the proposed DF-MSCD, surpassing the existing state-of-the-arts on multiple popular benchmarks. To emphasize, our method can obtain heterogeneous students by training once, which is superior to single-student DFKD methods in terms of both training time and testing accuracy.
更多查看译文
关键词
Knowledge distillation,Adversarial training,Model inversion,Surrogate images,Mutual learning
AI 理解论文
溯源树
样例
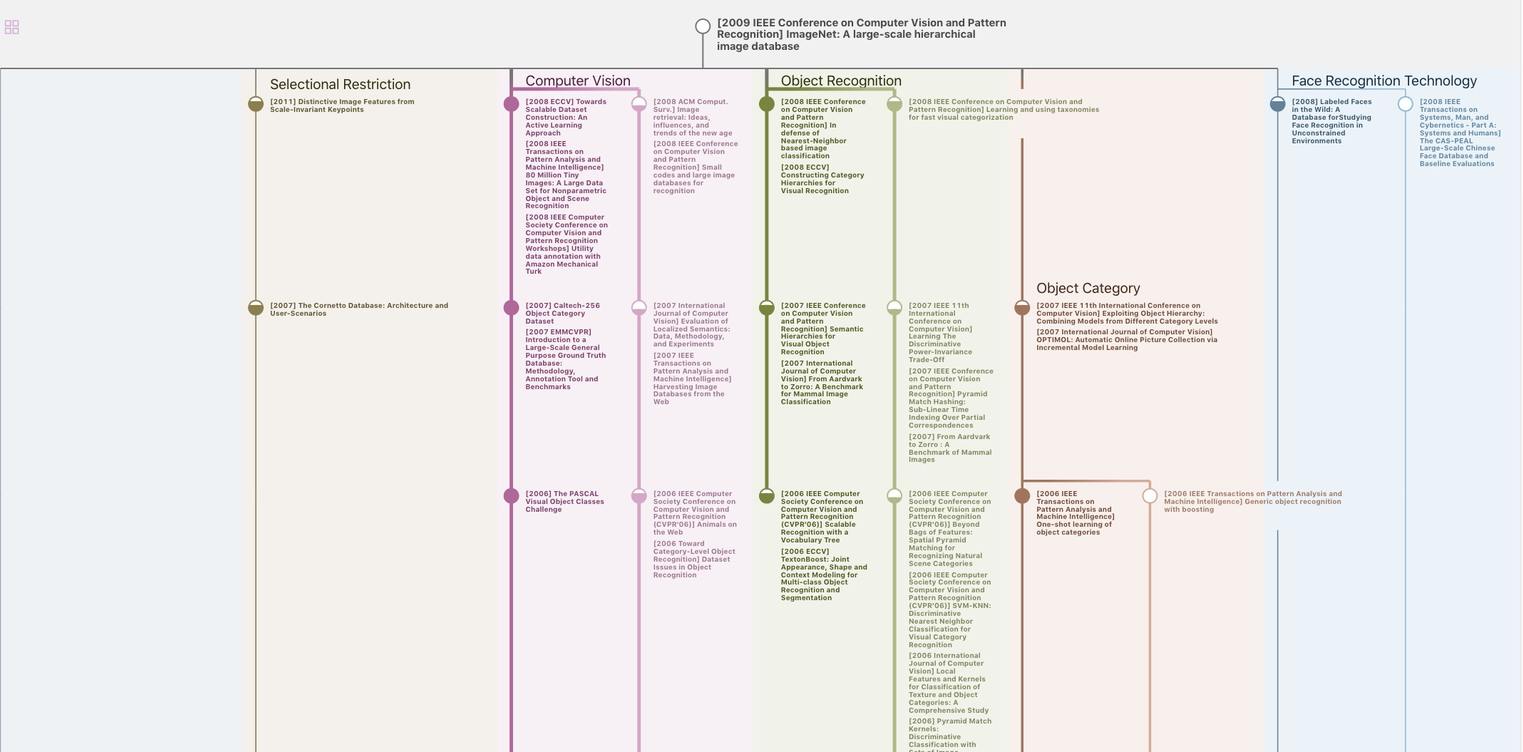
生成溯源树,研究论文发展脉络
Chat Paper
正在生成论文摘要