Semantic Role Assisted Natural Language Rule Formalization for Intelligent Vehicle.
RuleML+RR(2023)
摘要
This paper proposes a novel pipeline to translate natural language rules and instructions for intelligent vehicles into temporal logic. The pipeline uses semantic role labeling (SRL), soft rule-based selection restrictions, and large language models (LLMs) to extract predicates, arguments, and temporal aspects from natural language rules and instruction. We then use the language understanding capability of LLMs to generate temporal logic rules from unstructured natural language text and additional information provided by SRL. We envision our model as a human-in-the-loop system that can facilitate the automated rule formalization for planning and verification systems in automated driving and drone planning. We demonstrate that our method can generate semantically correct temporal logic formulas from natural language text and provide implicit explanations of the output by showing the intermediate reasoning steps involved. This paper illustrates the integration of additional semantic knowledge and LLM and its application for the intelligent system domain of automated driving and drone planning. Our generalizable pipeline can easily extend to new logic formalization types, traffic rules, drone planning instructions, and application domains.
更多查看译文
关键词
rule
AI 理解论文
溯源树
样例
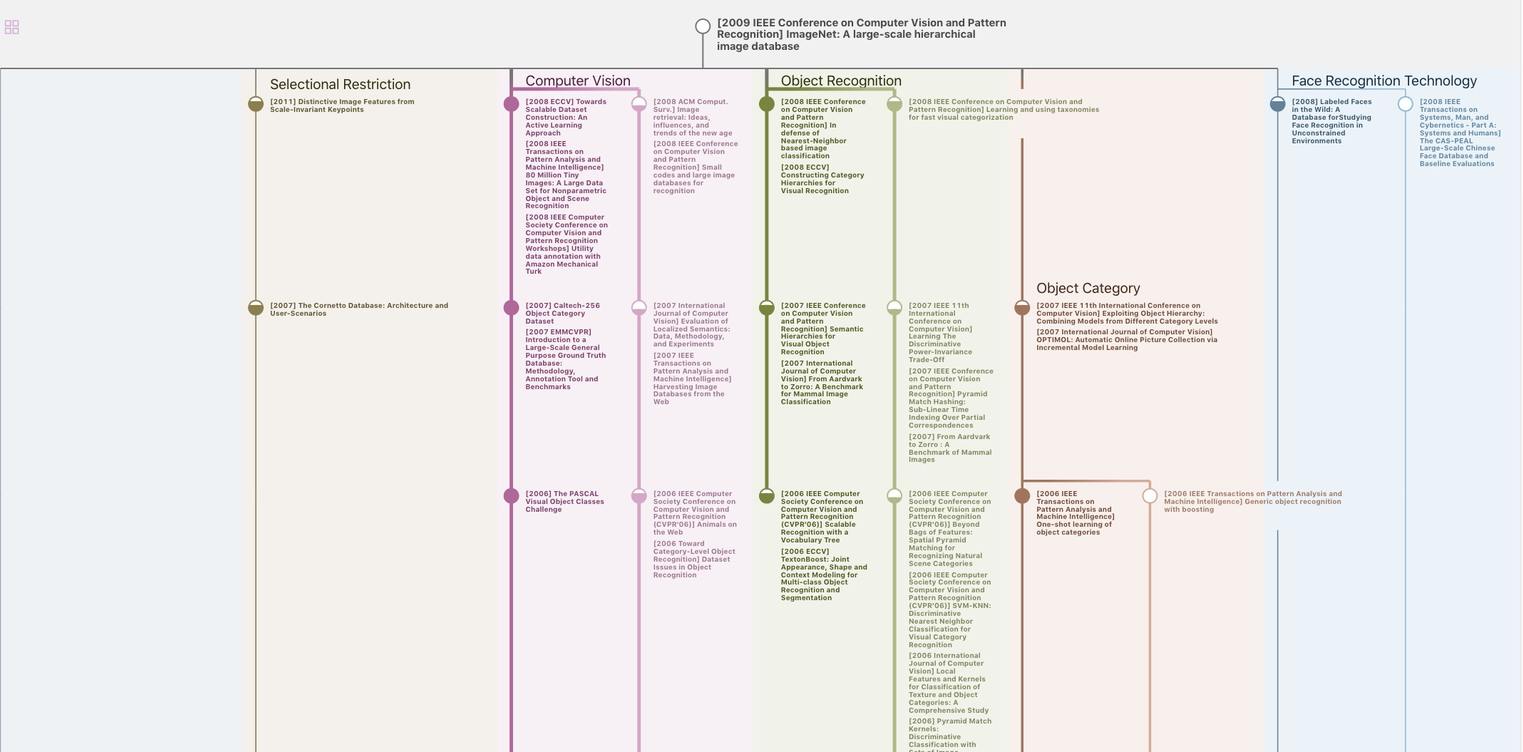
生成溯源树,研究论文发展脉络
Chat Paper
正在生成论文摘要