User-level Differentially Private Stochastic Convex Optimization: Efficient Algorithms with Optimal Rates
International Conference on Artificial Intelligence and Statistics(2023)
摘要
We study differentially private stochastic convex optimization (DP-SCO) under user-level privacy, where each user may hold multiple data items. Existing work for user-level DP-SCO either requires super-polynomial runtime [Ghazi et al. (2023)] or requires the number of users to grow polynomially with the dimensionality of the problem with additional strict assumptions [Bassily et al. (2023)]. We develop new algorithms for user-level DP-SCO that obtain optimal rates for both convex and strongly convex functions in polynomial time and require the number of users to grow only logarithmically in the dimension. Moreover, our algorithms are the first to obtain optimal rates for non-smooth functions in polynomial time. These algorithms are based on multiple-pass DP-SGD, combined with a novel private mean estimation procedure for concentrated data, which applies an outlier removal step before estimating the mean of the gradients.
更多查看译文
关键词
private stochastic convex optimization,optimal rates,efficient algorithms,user-level
AI 理解论文
溯源树
样例
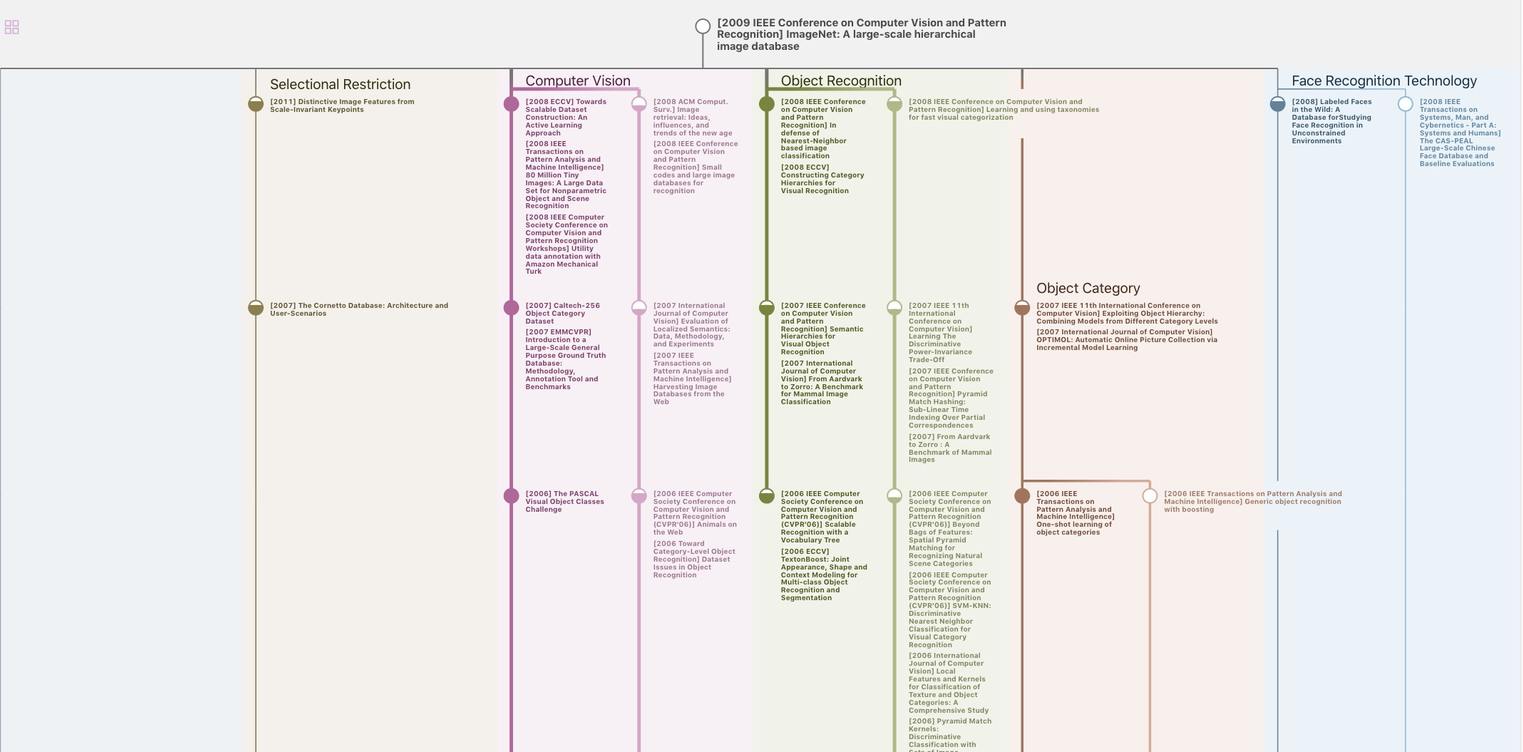
生成溯源树,研究论文发展脉络
Chat Paper
正在生成论文摘要