Clinical Applications, Methodology, and Scientific Reporting of Electrocardiogram Deep-Learning Models: A Systematic Review
JACC: Advances(2023)
摘要
The electrocardiogram (ECG) is one of the most common diagnostic tools available to assess cardiovascular health. The advent of advanced computational techniques such as deep learning has dramatically expanded the breadth of clinical problems that can be addressed using ECG data, leading to increasing popularity of ECG deep-learning models aimed at predicting clinical endpoints. The purpose of this study was to define the current landscape of clinically relevant ECG deep-learning models and examine practices in the scientific reporting of these studies. We performed a systematic review of PubMed and EMBASE databases to identify clinically relevant ECG deep-learning models published through July 1, 2022. We identified 44 manuscripts including 53 unique, clinically relevant ECG deep-learning models. The rate of publication of ECG deep-learning models is increasing rapidly. The most common clinical applications of ECG deep learning were identification of cardiomyopathy (14/53 [26%]), followed by arrhythmia detection (9/53 [17%]). Methodologic reporting varied; while 33/44 (75%) publications included model architecture diagrams, complete information required to reproduce these models was provided in only 10/44 (23%). Saliency analysis was performed in 20/44 (46%) of publications. Only 18/53 (34%) models were tested within external validation cohorts. Model code or resources allowing for model implementation by external groups were available for only 5/44 (11%) publications. While ECG deep-learning models are increasingly clinically relevant, their reporting is highly variable, and few publications provide sufficient detail for methodologic reproduction or model validation by external groups. The field of ECG deep learning would benefit from adherence to a set of standardized scientific reporting guidelines.
更多查看译文
关键词
deep-learning deep-learning models,deep-learning deep-learning,clinical applications
AI 理解论文
溯源树
样例
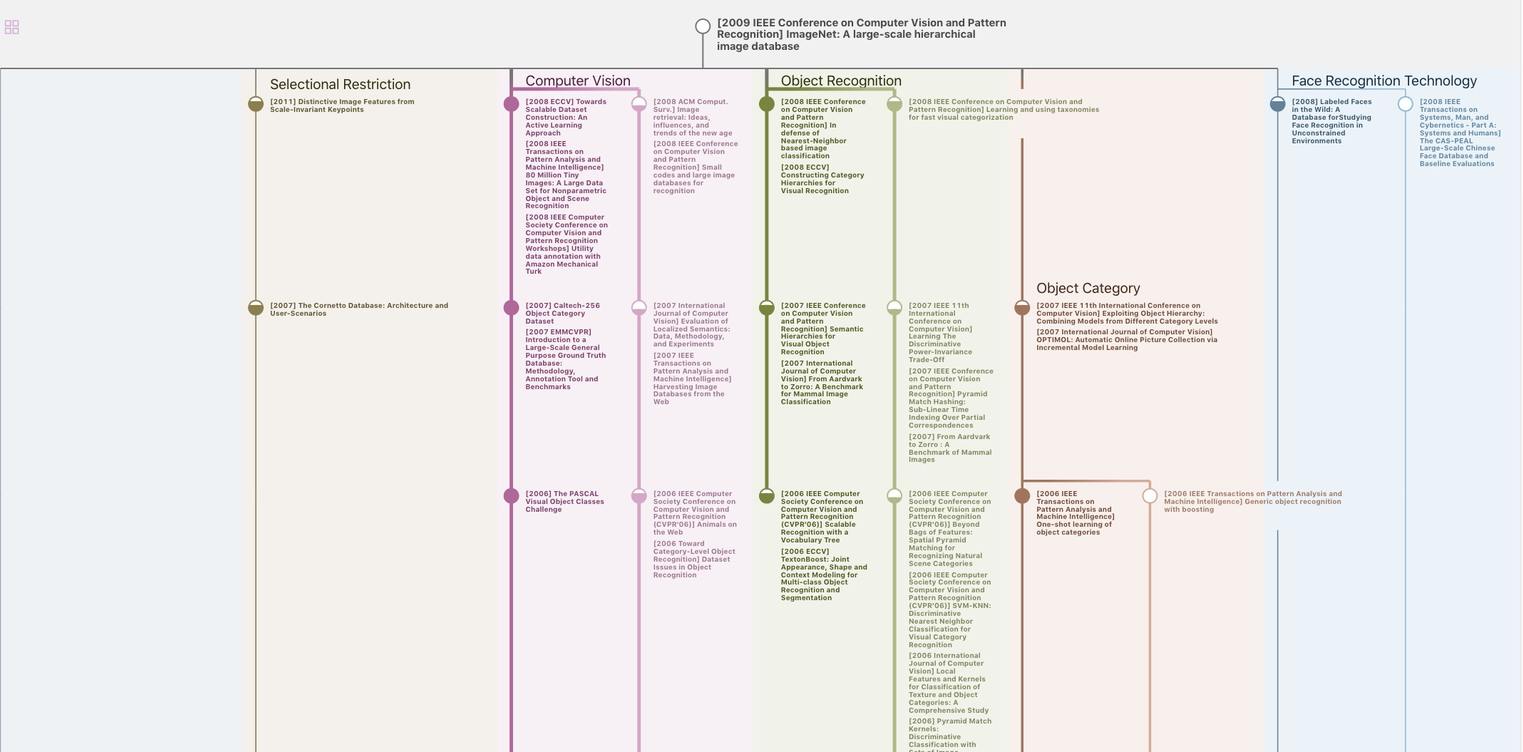
生成溯源树,研究论文发展脉络
Chat Paper
正在生成论文摘要