Run and Chase: Towards Accurate Source-Free Domain Adaptive Object Detection
2023 IEEE INTERNATIONAL CONFERENCE ON MULTIMEDIA AND EXPO, ICME(2023)
摘要
Recently, there has been increasing interest in the Source-Free Domain Adaptive Object Detection task, which involves training an object detector on the unlabeled target data using a pre-trained source model without accessing the source data. Most related methods are developed from the mean-teacher framework, which aims to train the student model closer to the teacher model via a pseudo labeling manner, where the teacher model is the exponential-moving-average of the student models at different time-steps. Following this line of works, we propose a Run-and-Chase Mutual-Learning method to strengthen the interactions between the student model and the teacher model in both feature and prediction levels. In our method, the student model is optimized to run away from the teacher model at the feature level, while chasing the teacher model at the prediction level. In this way, the student model is forced to be distinguishable at different time-steps, so that the teacher model can acquire more diverse task-related information and produce higher-accuracy pseudo labels. As the training goes, the student and teacher models are updated iteratively and promoted mutually, which can prevent the model collapse problem. Extensive experiments are conducted to validate the effectiveness of our method.
更多查看译文
关键词
Object Detection,Transfer Learning,Unsupervised Domain Adaptation
AI 理解论文
溯源树
样例
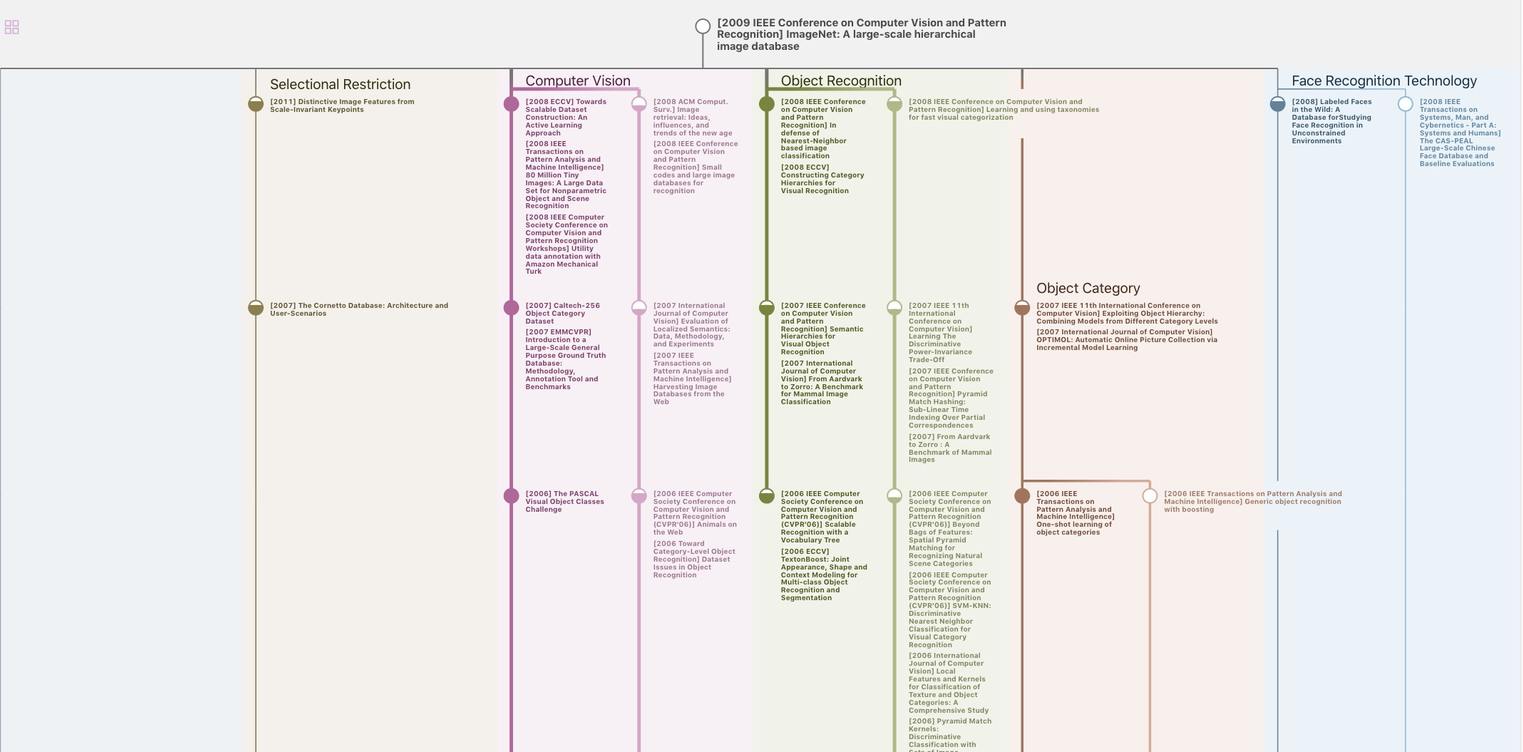
生成溯源树,研究论文发展脉络
Chat Paper
正在生成论文摘要