Open-World Graph Active Learning for Node Classification
ACM TRANSACTIONS ON KNOWLEDGE DISCOVERY FROM DATA(2024)
摘要
The great power of Graph Neural Networks (GNNs) relies on a large number of labeled training data, but obtaining the labels can be costly in many cases. Graph Active Learning (GAL) is proposed to reduce such annotation costs, but the existing methods mainly focus on improving labeling efficiency with fixed classes, and are limited to handle the emergence of novel classes. We term the problem as Open-World Graph Active Learning (OWGAL) and propose a framework of the same name. The key is to recognize novel-class as well as informative nodes in a unified framework. Instead of a fully connected neural network classifier, OWGAL employs prototype learning and label propagation to assign high uncertainty scores to the targeted nodes in the representation and topology space, respectively. Weighted sampling further suppresses the impact of unimportant classes by weighing both the node and class importance. Experimental results on four large-scale datasets demonstrate that our framework achieves a substantial improvement of 5.97% to 16.57% on Macro-F1 over state-of-the-art methods.
更多查看译文
关键词
Graph active learning,open-world learning,prototype learning
AI 理解论文
溯源树
样例
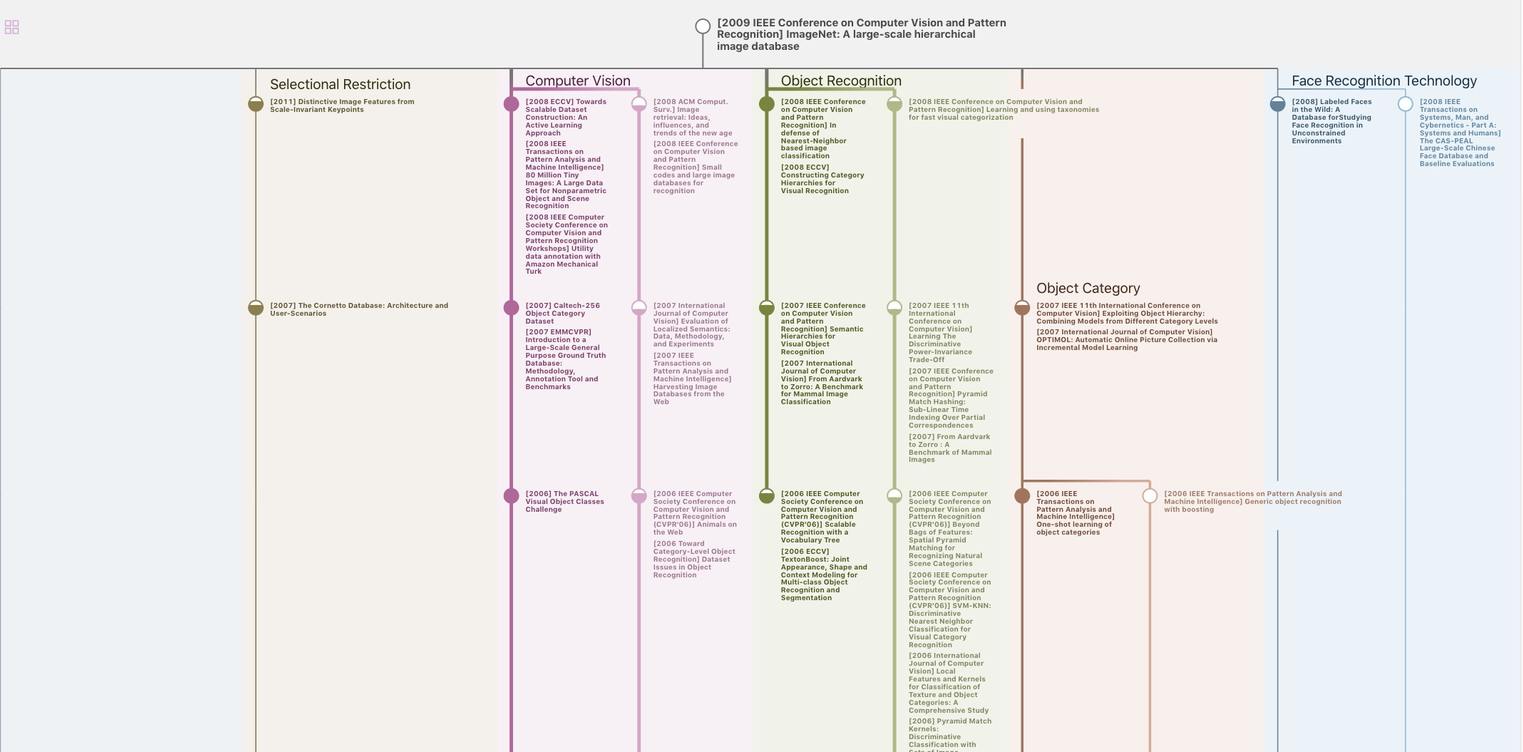
生成溯源树,研究论文发展脉络
Chat Paper
正在生成论文摘要