Memory Network-Based Interpreter of User Preferences in Content-Aware Recommender Systems
ACM TRANSACTIONS ON INTELLIGENT SYSTEMS AND TECHNOLOGY(2023)
摘要
This article introduces a novel architecture for two objectives recommendation and interpretability in a unified model. We leverage textual content as a source of interpretability in content-aware recommender systems. The goal is to characterize user preferences with a set of human-understandable attributes, each is described by a single word, enabling comprehension of user interests behind item adoptions. This is achieved via a dedicated architecture, which is interpretable by design, involving two components for recommendation and interpretation. In particular, we seek an interpreter, which accepts holistic user's representation from a recommender to output a set of activated attributes describing user preferences. Besides encoding interpretability properties such as fidelity, conciseness and diversity, the proposed memory network-based interpreter enables the generalization of user representation by discovering relevant attributes that go beyond her adopted items' textual content. We design experiments involving both human-and functionally-grounded evaluations of interpretability. Results on four real-world datasets show that our proposed model not only discovers highly relevant attributes for interpreting user preferences, but also enjoys comparable or better recommendation accuracy than a series of baselines.
更多查看译文
关键词
Interpretable user preferences,content-aware recommendation,memory network
AI 理解论文
溯源树
样例
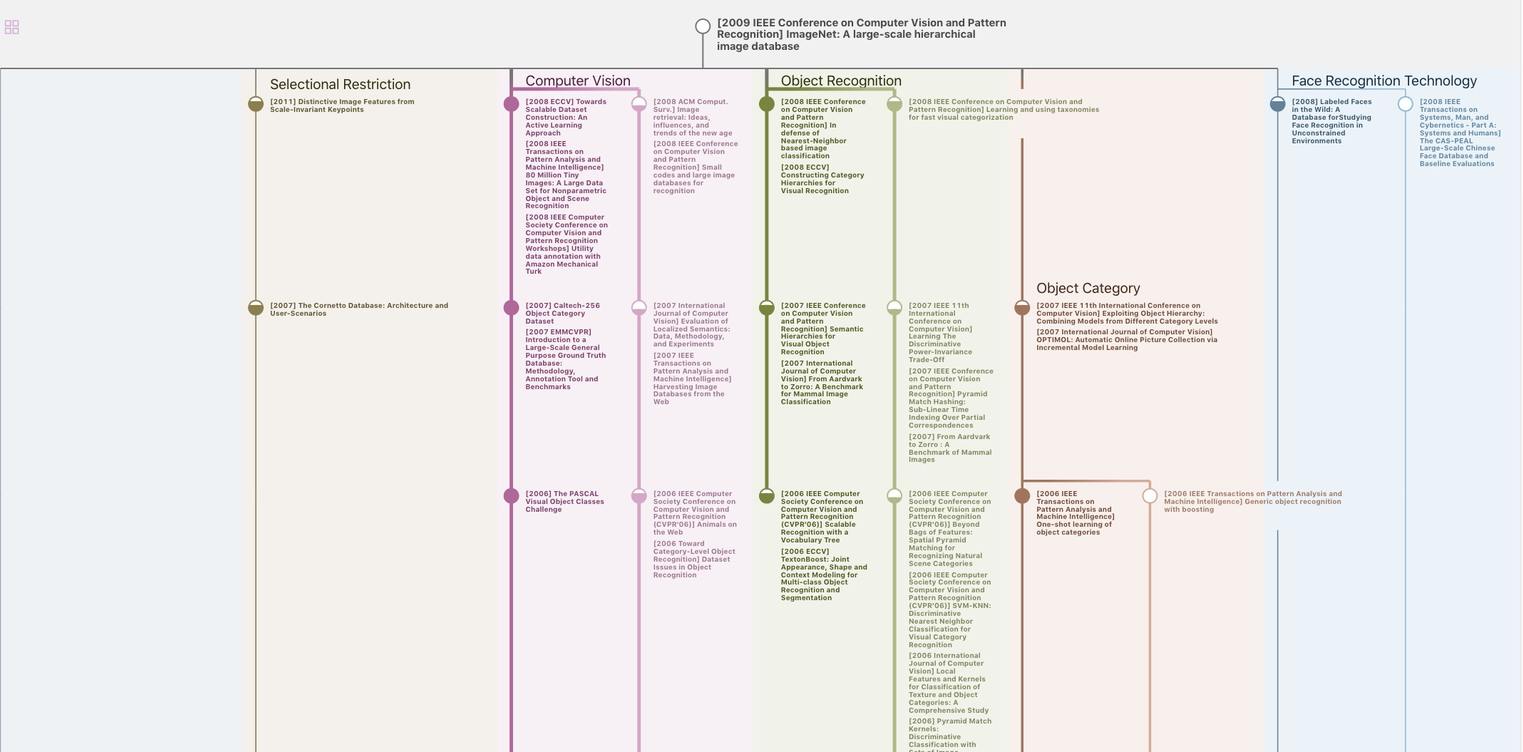
生成溯源树,研究论文发展脉络
Chat Paper
正在生成论文摘要