Large Pre-trained Time Series Models for Cross-Domain Time Series Analysis Tasks
arXiv (Cornell University)(2023)
摘要
Large pre-trained models have been vital in recent advancements in domains like language and vision, making model training for individual downstream tasks more efficient and provide superior performance. However, tackling time-series analysis tasks usually involves designing and training a separate model from scratch leveraging training data and domain expertise specific to the task. We tackle a significant challenge for pre-training a foundational time-series model from multi-domain time-series datasets: extracting semantically useful tokenized inputs to the model across heterogenous time-series from different domains. We propose Large Pre-trained Time-series Models (LPTM) that introduces a novel method of adaptive segmentation that automatically identifies optimal dataset-specific segmentation strategy during pre-training. This enables LPTM to perform similar to or better than domain-specific state-of-art model when fine-tuned to different downstream time-series analysis tasks and under zero-shot settings. LPTM achieves superior forecasting and time-series classification results taking up to 40 compared to state-of-art baselines.
更多查看译文
关键词
Time Series Forecasting,LSTM Networks,Forecasting Models
AI 理解论文
溯源树
样例
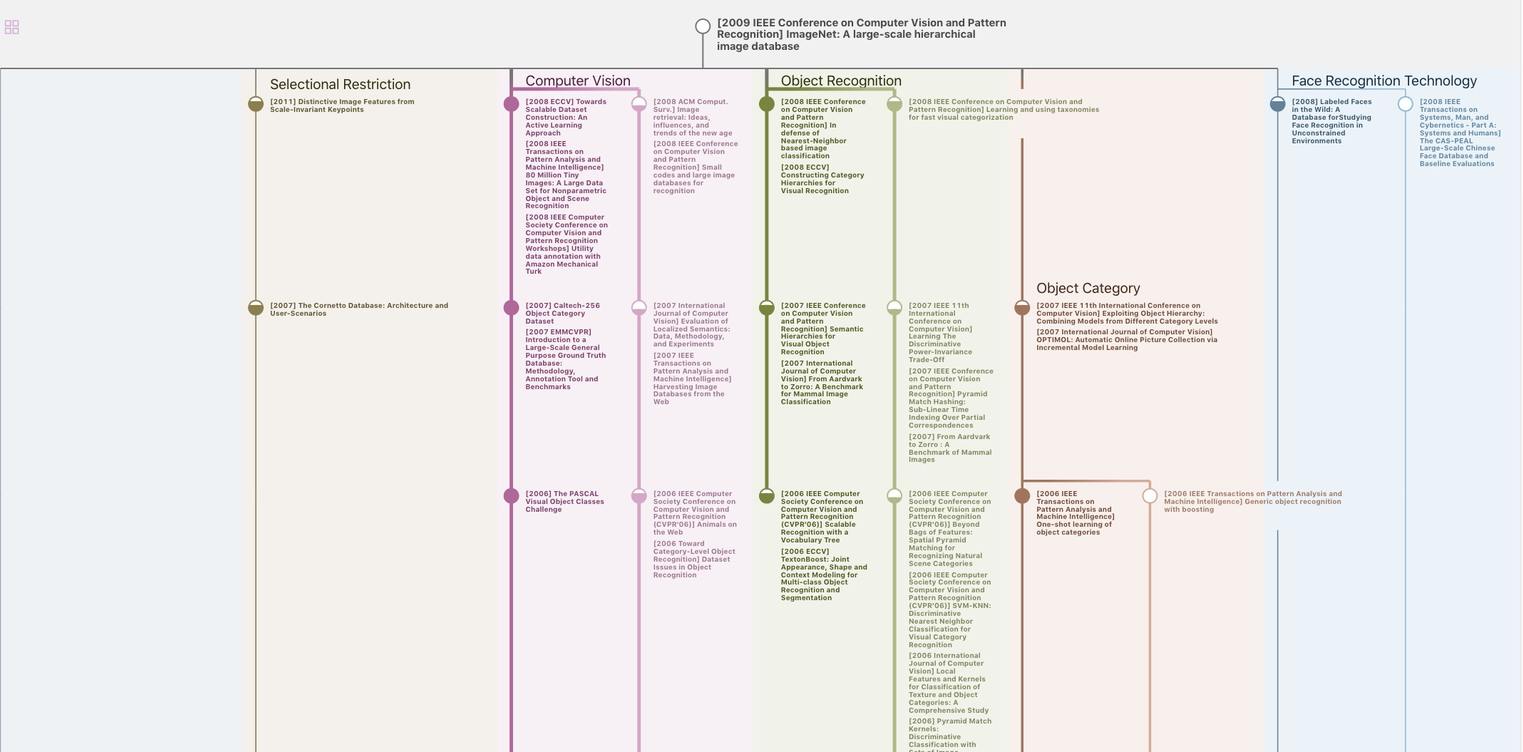
生成溯源树,研究论文发展脉络
Chat Paper
正在生成论文摘要