Multi-accent pronunciation assessment based on domain adversarial training
2023 ASIA PACIFIC SIGNAL AND INFORMATION PROCESSING ASSOCIATION ANNUAL SUMMIT AND CONFERENCE, APSIPA ASC(2023)
摘要
Second language learners are usually influenced by their first languages and thus produce varied and complex accents difficult for pronunciation assessment. Meanwhile, obtaining sufficient human-labeled data for accented speech is tedious and costly, which limits the robustness and accuracy of pronunciation assessment. In this paper, we propose an end-to-end (E2E) method for multi-accent pronunciation assessment. We utilize a cross-lingual pre-trained acoustic model to ensure the discriminative capability of feature representations for the assessment of different accents. Moreover, to improve the robustness of pronunciation assessment on low-resource or unannotated accented speech, we employ domain adversary training to make representations accent-invariant for assessment. Experimental results show the proposed method outperforms the baselines across multiple accents of English, including Chinese, Korean, German, and Indonesian, in the Pearson correlation coefficient (PCC). When assessing unseen English pronunciation with Japanese accents, it also shows superiority to other baselines.
更多查看译文
关键词
pronunciation assessment,multi-accent,multi-lingual pre-trained acoustic model,low-resource accented data,domain adversarial training
AI 理解论文
溯源树
样例
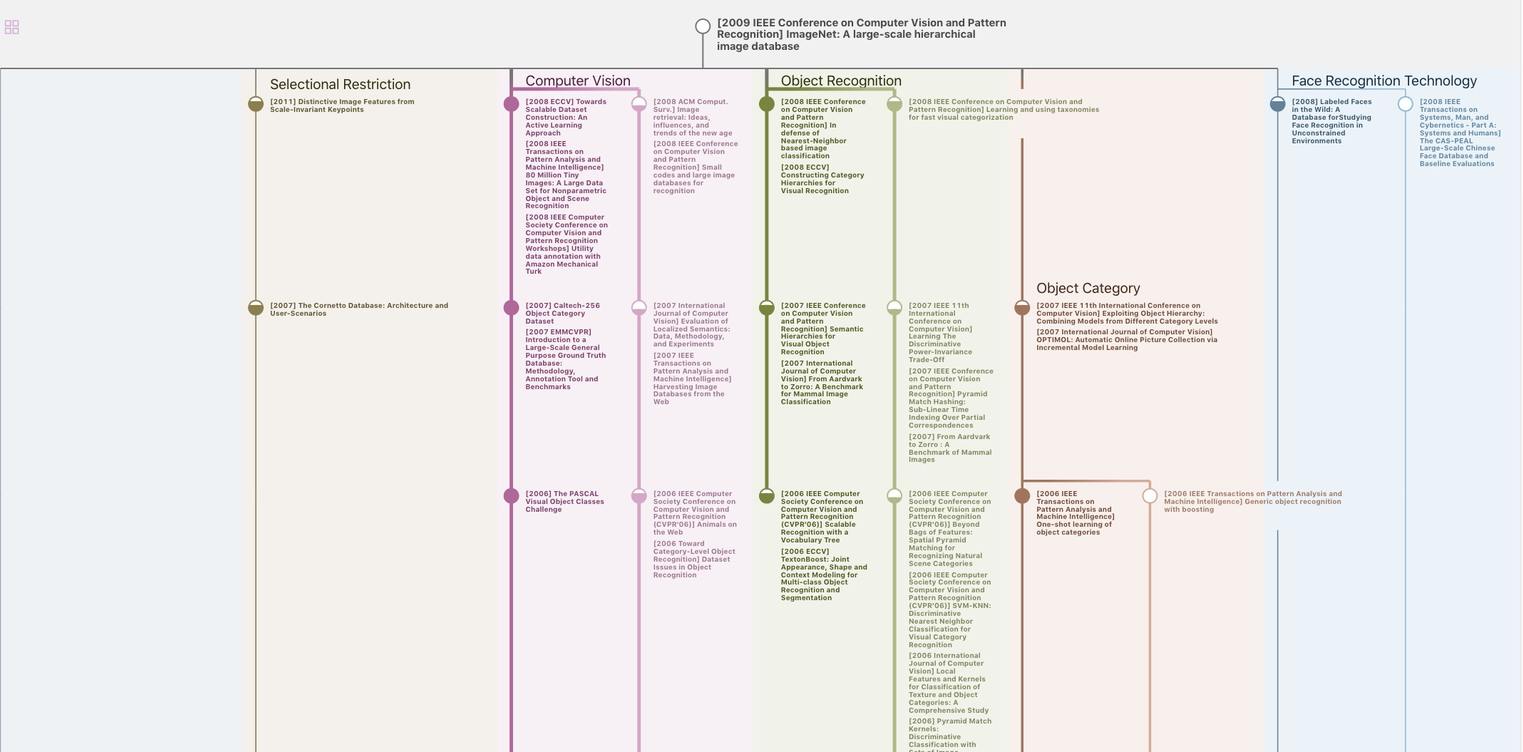
生成溯源树,研究论文发展脉络
Chat Paper
正在生成论文摘要