Scale-Adaptive Multi-area Representation for Instance Segmentation.
Image and Graphics : 12th International Conference, ICIG 2023, Nanjing, China, September 22–24, 2023, Proceedings, Part IV(2023)
摘要
For the instance segmentation task, instance representation directly determines the quality of generated masks, so achieving efficient and accurate instance representation is crucial. Grid-based or box-based instance representation contains redundant information from background or other instances, activation-based instance representation includes a small part of the instance. The instance representation based on current methods is not accurate enough. In order to represent more information of an instance under the condition of excluding irrelevant information, this paper proposes multi-area representation (MAR), which is in the form of a scale-adaptive multi-area activation map generated by a multi-branch structure. MAR can adapt to the structure and pose of an instance, thereby representing the shape and size of the instance. Experiments show that, compared with SparseInst, MARInst can improve the performance of instance segmentation and keep the inference speed and training memory almost unchanged. In particular, MARInst achieved 30.3% AP on the MS COCO 2017 val, and 1.6% AP higher than SparseInst when using the same ResNet-50 backbone, proving the effectiveness of the proposed method.
更多查看译文
关键词
instance segmentation,scale-adaptive,multi-area
AI 理解论文
溯源树
样例
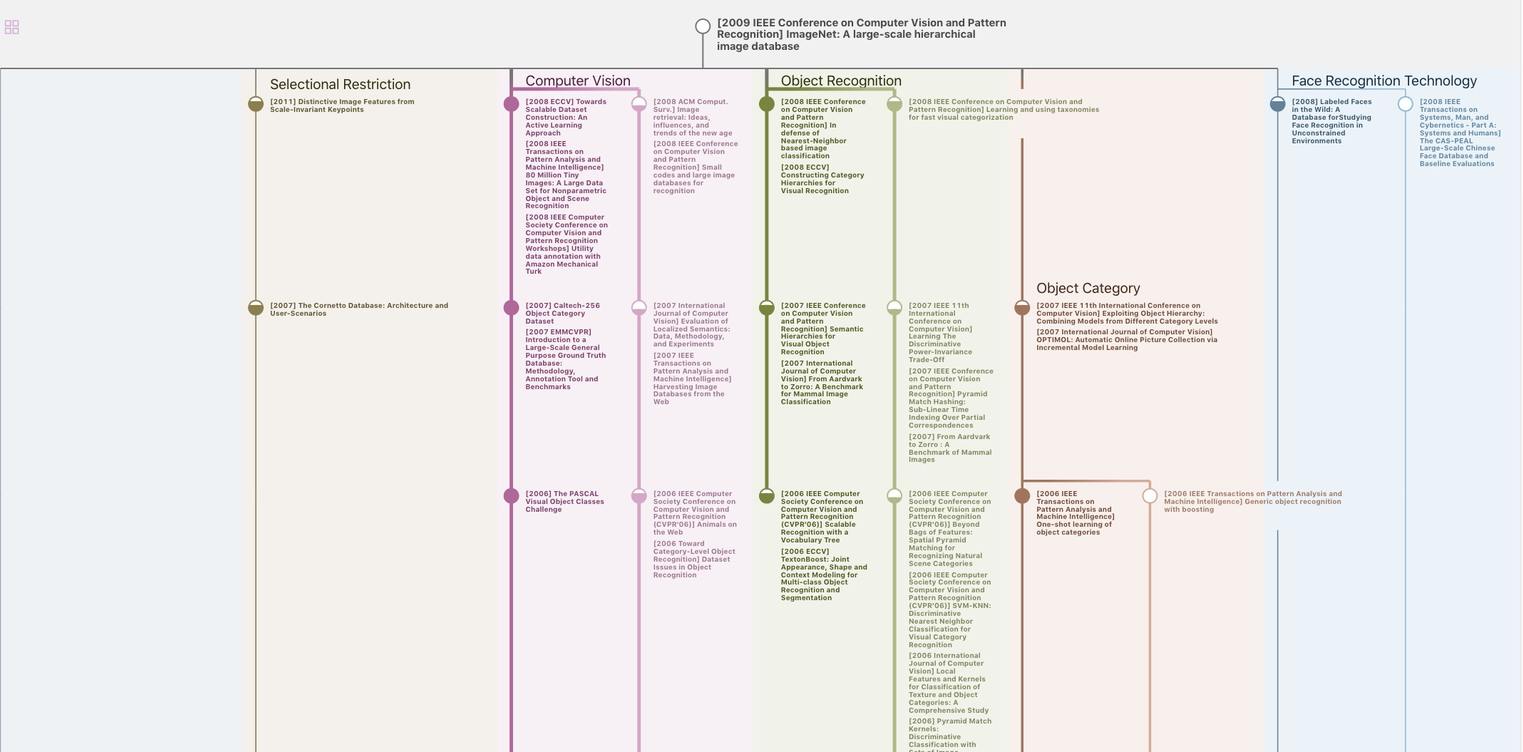
生成溯源树,研究论文发展脉络
Chat Paper
正在生成论文摘要