Gaining Biological Insights through Supervised Data Visualization
bioRxiv the preprint server for biology(2024)
摘要
Dimensionality reduction-based data visualization is pivotal in comprehending complex biological data. The most common methods, such as PHATE, t-SNE, and UMAP, are unsupervised and therefore reflect the dominant structure in the data, which may be independent of expert-provided labels. Here we introduce a supervised data visualization method called RF-PHATE, which integrates expert knowledge for further exploration of the data. RF-PHATE leverages random forests to capture intricate featurelabel relationships. Extracting information from the forest, RF-PHATE generates low-dimensional visualizations that highlight relevant data relationships while disregarding extraneous features. This approach scales to large datasets and applies to classification and regression. We illustrate RF-PHATE’s prowess through three case studies. In a multiple sclerosis study using longitudinal clinical and imaging data, RF-PHATE unveils a sub-group of patients with non-benign relapsingremitting Multiple Sclerosis, demonstrating its aptitude for time-series data. In the context of Raman spectral data, RF-PHATE effectively showcases the impact of antioxidants on diesel exhaust-exposed lung cells, highlighting its proficiency in noisy environments. Furthermore, RF-PHATE aligns established geometric structures with COVID-19 patient outcomes, enriching interpretability in a hierarchical manner. RF-PHATE bridges expert insights and visualizations, promising knowledge generation. Its adaptability, scalability, and noise tolerance underscore its potential for widespread adoption.
### Competing Interest Statement
The authors have declared no competing interest.
更多查看译文
AI 理解论文
溯源树
样例
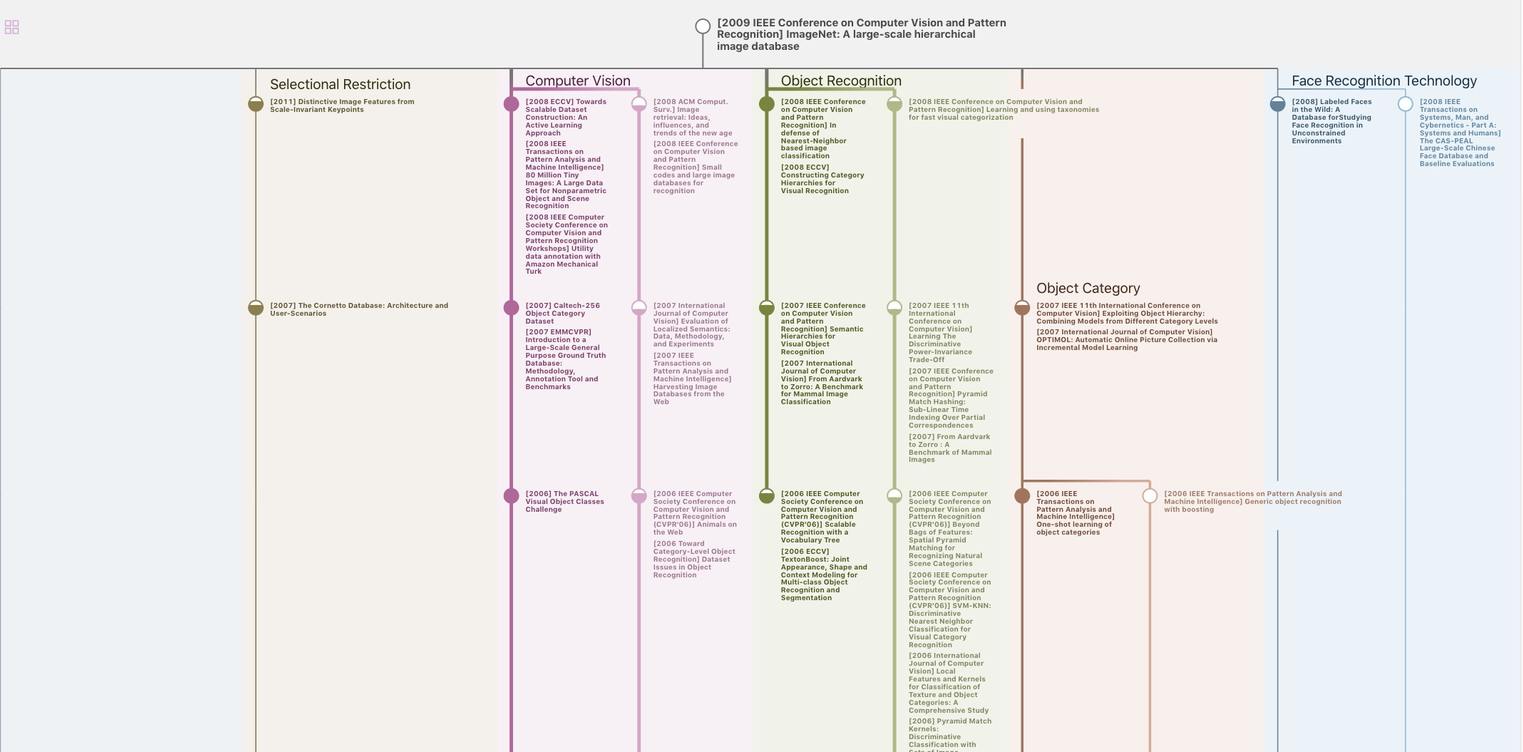
生成溯源树,研究论文发展脉络
Chat Paper
正在生成论文摘要