Attribution Guided Layerwise Knowledge Amalgamation from Graph Neural Networks
NEURAL INFORMATION PROCESSING, ICONIP 2023, PT I(2024)
摘要
Knowledge Amalgamation (KA), aiming to transfer knowledge from multiple well-trained teacher networks to a multi-talented and compact student, is gaining attention due to its crucial role in resource-constrained scenarios. Previous literature on KA, although exhibiting promising results, is primarily geared toward Convolutional Neural Networks (CNNs). However, when transferred to Graph Neural Networks (GNNs) with non-grid data, KA techniques face new challenges that can be difficult to overcome. Moreover, the layerwise aggregation of GNNs produces significant noise as they progress from a shallow to a deep level, which can impede KA students' deep-level semantic comprehension. This work aims to overcome this limitation and propose a novel strategy termed LAyerwIse Knowledge Amalgamation (LaiKA). It involves Hierarchical Feature Alignment between the teachers and the student, which enables the student to directly master the feature aggregation rules from teacher GNNs. Meanwhile, we propose a Selective Attribution Transfer (SAT) module that identifies task-relevant topological substructures to assist the capacity-limited student in mitigating noise and enhancing performance. Extensive experiments conducted on six datasets demonstrate that our proposed method equips a single student GNN to handle tasks from multiple teachers effectively and achieve comparable or superior results to those of the teachers without human annotations.
更多查看译文
关键词
Knowledge Amalgamation,Knowledge Transfer,Attribution Graph,Graph Neural Networks
AI 理解论文
溯源树
样例
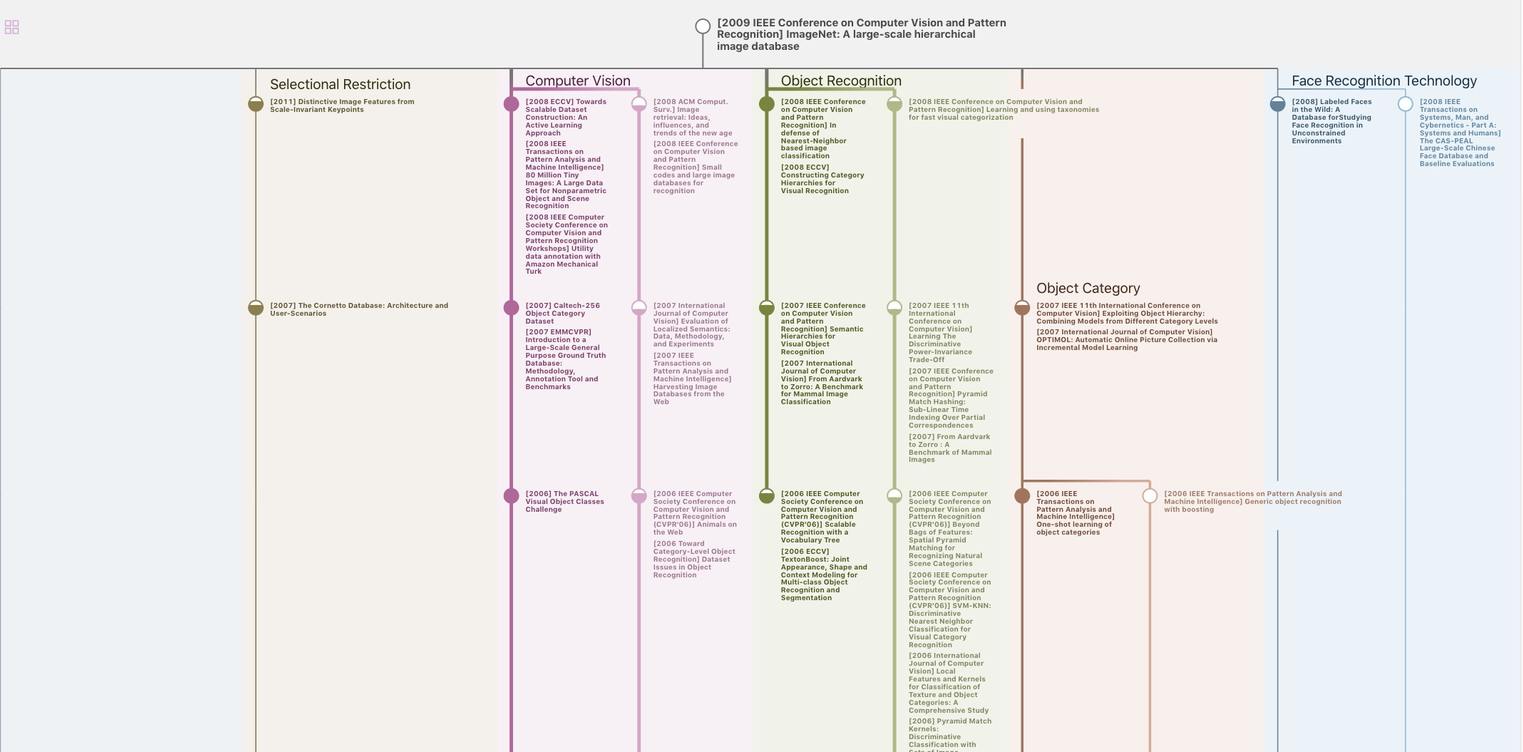
生成溯源树,研究论文发展脉络
Chat Paper
正在生成论文摘要