Graph Contrastive Learning with Wasserstein Distance for Recommendation
2023 8th International Conference on Intelligent Computing and Signal Processing (ICSP)(2023)
Abstract
Contrastive learning has been applied to graph collaborative filtering in recent years as a support task to boost efficiency. The majority of these approaches ignore global differences in favor of local variations among subgraphs. Additionally, different data augmentation strategies need to be developed for various datasets. Further, similarity metrics based on vector inner products might obscure sample-to-sample structural variations. To achieve this, we propose a method that adaptively samples subgraphs first, then uses a Wasserstein-based subgraph similarity measure to capture subgraph overall differences. To be more precise, we sample subgraph nodes from close to far, create subgraph edges using a similarity measure, and then build a contrastive loss based on Wasserstein distance to capture the distinction between pairs. Through extensive experimentation, we show the proposed method to be effective.
MoreTranslated text
Key words
Contrastive Learning,Recommendation System,Wasserstein Distance,Graph Neural Network
AI Read Science
Must-Reading Tree
Example
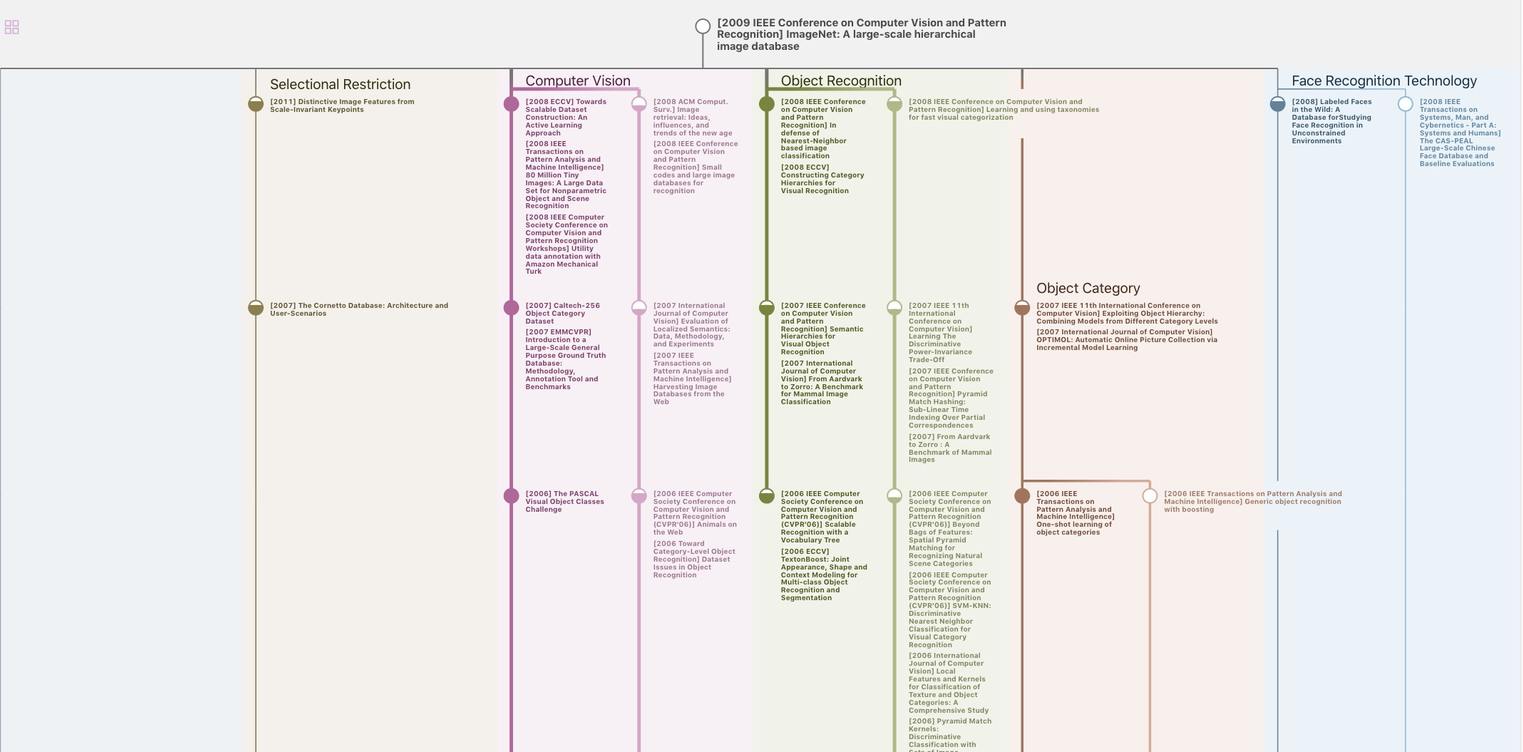
Generate MRT to find the research sequence of this paper
Chat Paper
Summary is being generated by the instructions you defined