Unsupervised Domain Adaptive Person Re-Identification with Adaptive Structure Learning
2023 IEEE International Conference on Image Processing (ICIP)(2023)
摘要
Unsupervised domain adaptive person re-identification has garnered considerable attention due to its practical significance. Previous research has focused on utilizing the teacher-student framework within the clustering and fine-tuning paradigm to reduce the domain gap between different person re-identification datasets. Building upon these recent advancements, we propose a novel approach to further explore the structural information of the teacher and student networks from multiple perspectives, including adaptive sample updating, global feature arrangement, and local discrepancy learning. By integrating these three components, we introduce the Adaptive Structure Learning Framework (ASL) for unsupervised domain adaptive person re-identification. Compared to the baseline method, our approach achieves a significant improvement of 7.3% in mean Average Precision (mAP) on the Market2MSMT task. Furthermore, our experimental results across three benchmark datasets provide further evidence of the effectiveness of our proposed method.
更多查看译文
关键词
Unsupervised Domain Adaptive,Person Re-identification,Knowledge Distillation
AI 理解论文
溯源树
样例
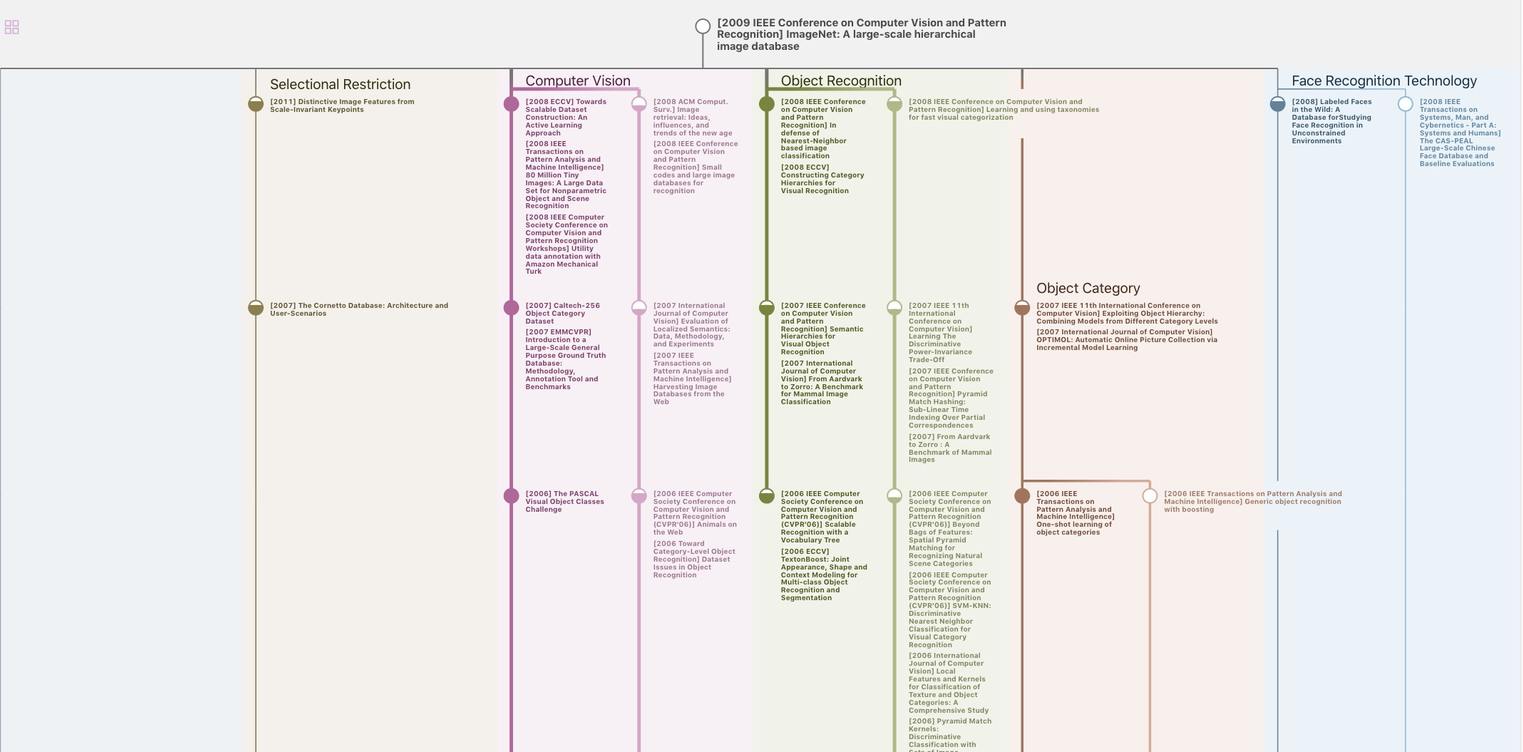
生成溯源树,研究论文发展脉络
Chat Paper
正在生成论文摘要