Asymptotic Analysis and Truncated Backpropagation for the Unrolled Primal-Dual Algorithm
2023 31st European Signal Processing Conference (EUSIPCO)(2023)
摘要
Algorithm unrolling combines the advantages of model based optimization with the flexibility of data-based methods by adapting a parameterized objective to a distribution of problem instances from a finite sample from that distribution. At inference time, a fixed number of iterations of a suitable optimization algorithm is used to make predictions on unseen data. To compute gradients for learning, the last iterate is differentiated with respect to the parameters by backpropagation schemes that get expensive when the number of unrolled iterations gets large. Therefore, only few unrolled iterations are used which compromises the claimed interpretability in terms of the underlying optimization objective. In this work, we consider convex objective functions, derive an explicit limit of the parameter gradients for a large number of unrolled iterations, derive a training procedure that is computationally tractable and retains interpretability, and show the effectiveness of the method using the example of speech dequantization.
更多查看译文
关键词
unrolling,learning to optimize,variational problems,convex optimization,speech dequantization
AI 理解论文
溯源树
样例
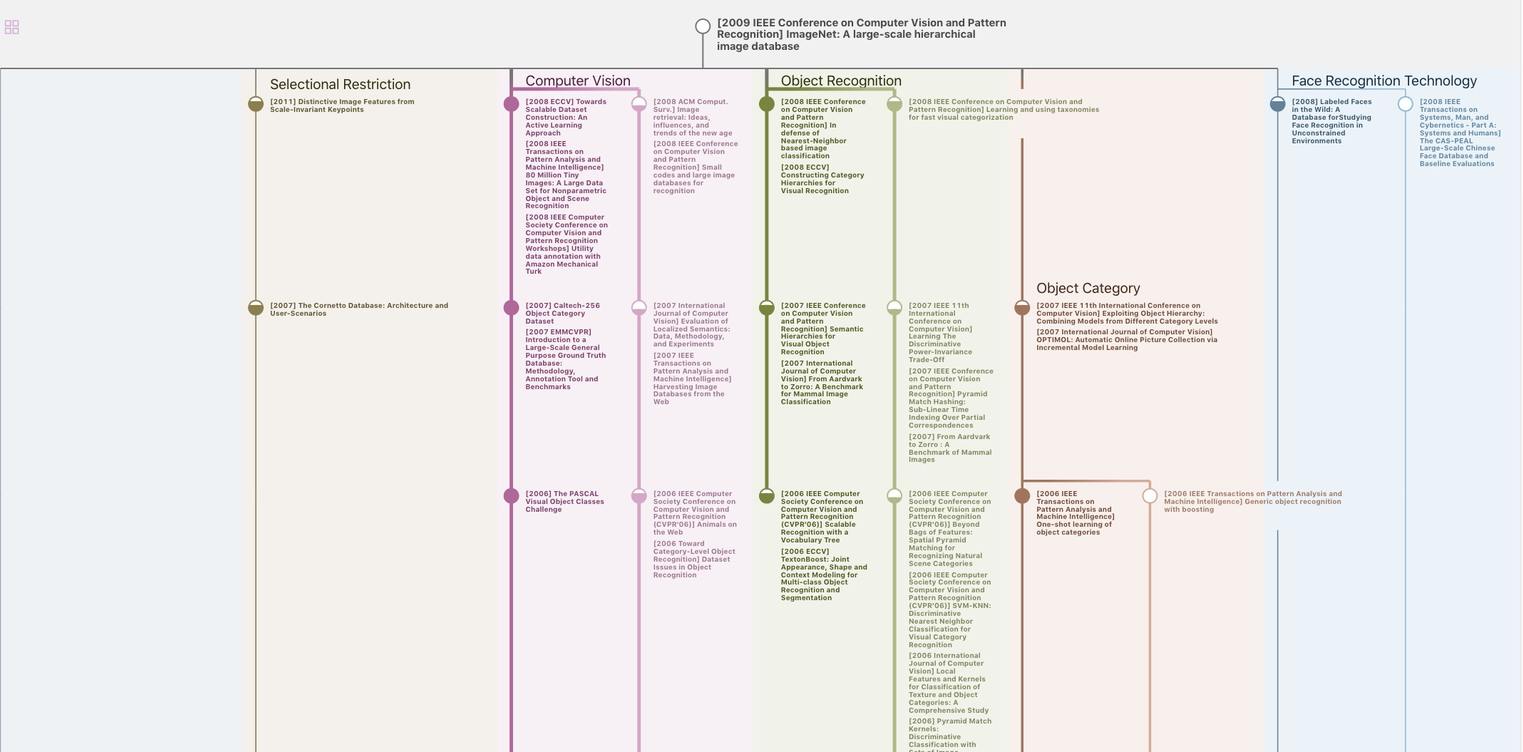
生成溯源树,研究论文发展脉络
Chat Paper
正在生成论文摘要