Chemical accuracy prediction of molecular solvation and partition in ionic liquids with educated estimators
JOURNAL OF MOLECULAR LIQUIDS(2023)
摘要
Ionic liquids (ILs) derivatives as novel green solvents are widely employed in laboratory and industrial applications. Many computational tools have been developed to compute various physiochemical properties of ILs species, e.g., mass density and viscosity. However, despite their central role as solvents, predictive tools for the solvation of external agents and the partition between water and ILs phases are rather limited. Common selections are atomistic simulations, especially the alchemical method. However, the relatively high computational costs could be harmful to large-scale applications. In this work, we develop a series of low-cost machine-learning estimators to exploit further the chemical-accuracy frontier. 1764 solvation and 1764 water-ILs transfer free energies involving 120 gaseous or drug-like solutes and many commonly applied ILs families are gathered from experimental references to form the dataset with a diverse coverage of chemical spaces. The best-performing tree-based method could predict simultaneously solvation and water-ILs biphasic partition thermodynamics with a state-of-the-art performance of - 0.13 kcal/mol MAE, -0.25 kcal/mol RMSE, -0.98 Pearson r and - 0.94 Kendall tau, beating transferable tools such as alchemical free energy calculations in a series of face-to-face comparisons.
更多查看译文
关键词
Ionic liquids,Machine learning regression,Solvation free energy,Partition coefficient,Chemical accuracy
AI 理解论文
溯源树
样例
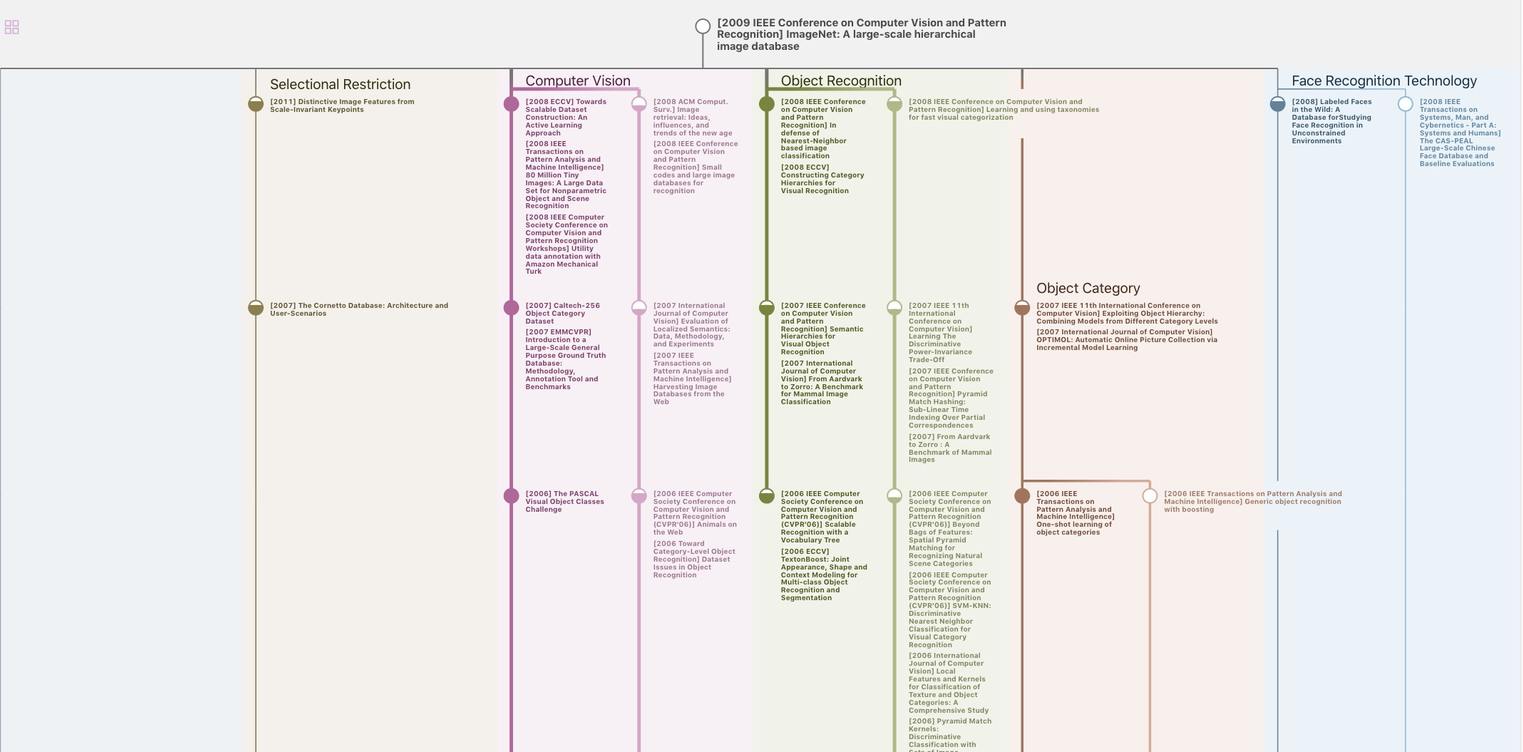
生成溯源树,研究论文发展脉络
Chat Paper
正在生成论文摘要