Supervised Kernel Principal Component Analysis for Forecasting
Finance research letters(2023)
Abstract
Principal component analysis (PCA) is a versatile tool for dimension reduction with many applications in finance, economics, and machine learning. This paper proposes a new approach to improving the forecasting ability of the Principal Components (PCs) of observed high-dimensional predictors in a factor-augmented forecasting framework. The approach is carried out in a three-step procedure, where we first perform a nonparametric kernel regression of the target variable on each predictor to obtain a new high-dimensional predictor vector formed by stacking all estimated kernel regression functions, we then extract PCs from these new predictors using PCA, and finally, we employ the extracted PCs as predictors to forecast the target variable. A real example on macroeconomic forecasting is analyzed and numerical results show that the proposed kernel PCA can outperform some commonly used forecasting approaches in out-of-sample prediction.
MoreTranslated text
Key words
Forecasting,Nonparametric regression,Principal component analysis,Dimension reduction
AI Read Science
Must-Reading Tree
Example
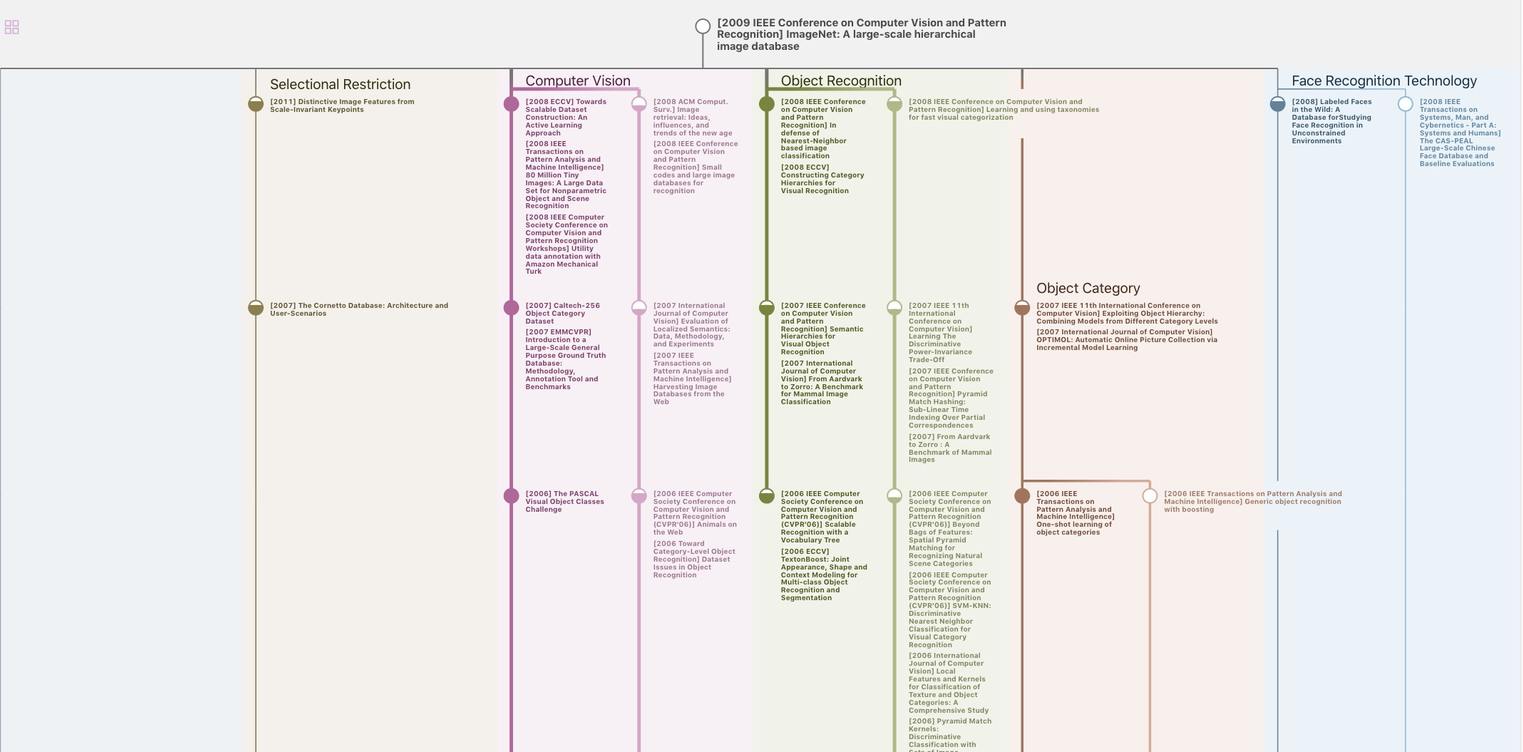
Generate MRT to find the research sequence of this paper
Chat Paper
Summary is being generated by the instructions you defined