Customize Your NeRF: Adaptive Source Driven 3D Scene Editing Via Local-Global Iterative Training
2024 IEEE/CVF Conference on Computer Vision and Pattern Recognition (CVPR)(2024)
摘要
In this paper, we target the adaptive source driven 3D scene editing task by proposing a CustomNeRF model that unifies a text description or a reference image as the editing prompt. However, obtaining desired editing results conformed with the editing prompt is nontrivial since there exist two significant challenges, including accurate editing of only foreground regions and multi-view consistency given a single-view reference image. To tackle the first challenge, we propose a Local-Global Iterative Editing (LGIE) training scheme that alternates between foreground region editing and full-image editing, aimed at foreground-only manipulation while preserving the background. For the second challenge, we also design a class-guided regularization that exploits class priors within the generation model to alleviate the inconsistency problem among different views in image-driven editing. Extensive experiments show that our CustomNeRF produces precise editing results under various real scenes for both text- and image-driven settings.
更多查看译文
关键词
3D Scene,Training Strategy,Reference Image,Textual Descriptions,Precise Editing,Foreground Regions,Specific Topics,Diffusion Model,Latent Space,Image Generation,Baseline Methods,Background Regions,Local Stage,Global Stage,Image Editing,Conduct Ablation Studies,Latent Code,Appearance Information,3rd Row,Neural Field,Edition Staging,Special Token,MSE Loss
AI 理解论文
溯源树
样例
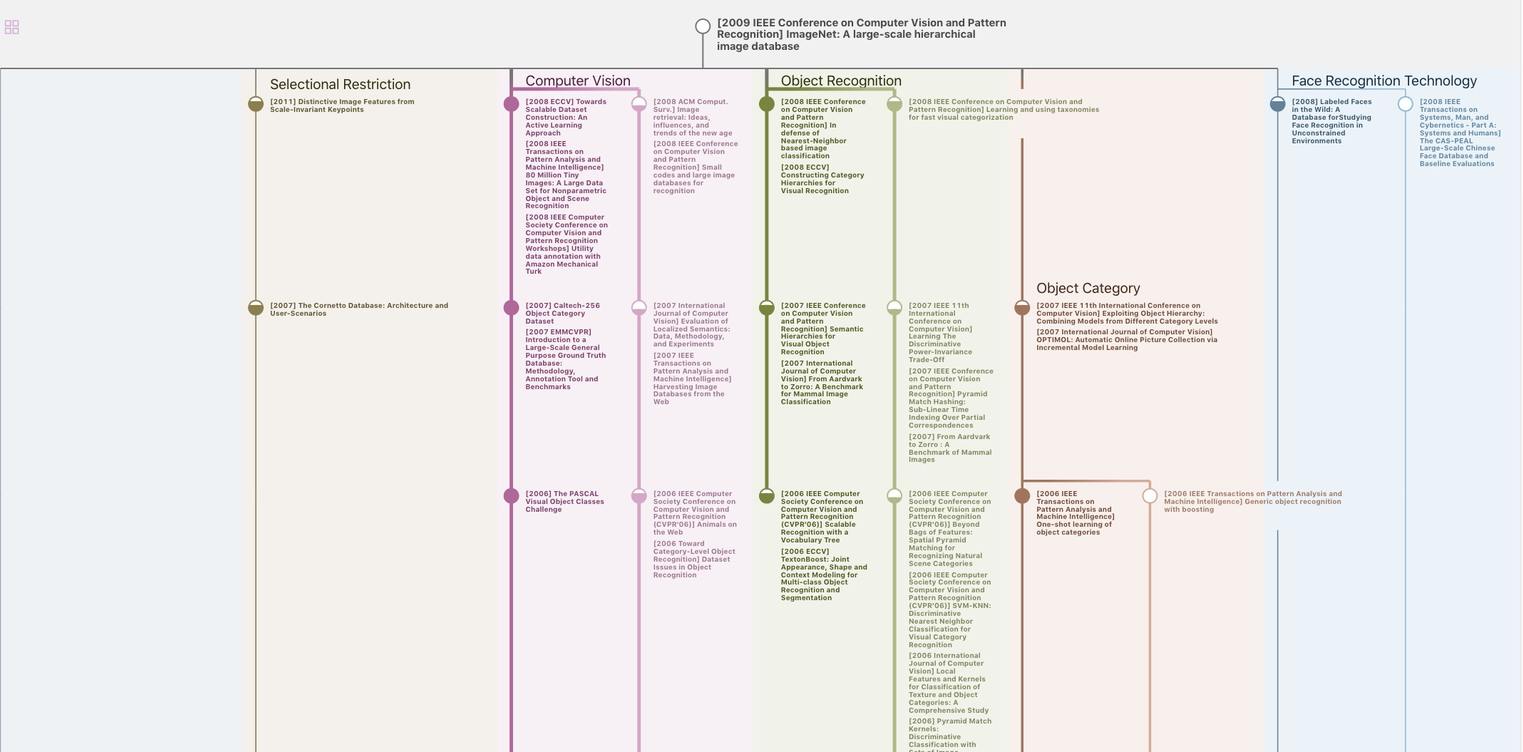
生成溯源树,研究论文发展脉络
Chat Paper
正在生成论文摘要