NeuralHOFusion: Neural Volumetric Rendering under Human-object Interactions
arXiv (Cornell University)(2022)
摘要
4D modeling of human-object interactions is critical for numerous applications. However, efficient volumetric capture and rendering of complex interaction scenarios, especially from sparse inputs, remain challenging. In this paper, we propose NeuralHOFusion, a neural approach for volumetric human-object capture and rendering using sparse consumer RGBD sensors. It marries traditional non-rigid fusion with recent neural implicit modeling and blending advances, where the captured humans and objects are layerwise disentangled. For geometry modeling, we propose a neural implicit inference scheme with non-rigid key-volume fusion, as well as a template-aid robust object tracking pipeline. Our scheme enables detailed and complete geometry generation under complex interactions and occlusions. Moreover, we introduce a layer-wise human-object texture rendering scheme, which combines volumetric and image-based rendering in both spatial and temporal domains to obtain photo-realistic results. Extensive experiments demonstrate the effectiveness and efficiency of our approach in synthesizing photo-realistic free-view results under complex human-object interactions.
更多查看译文
关键词
neuralhofusion volumetric,human-object
AI 理解论文
溯源树
样例
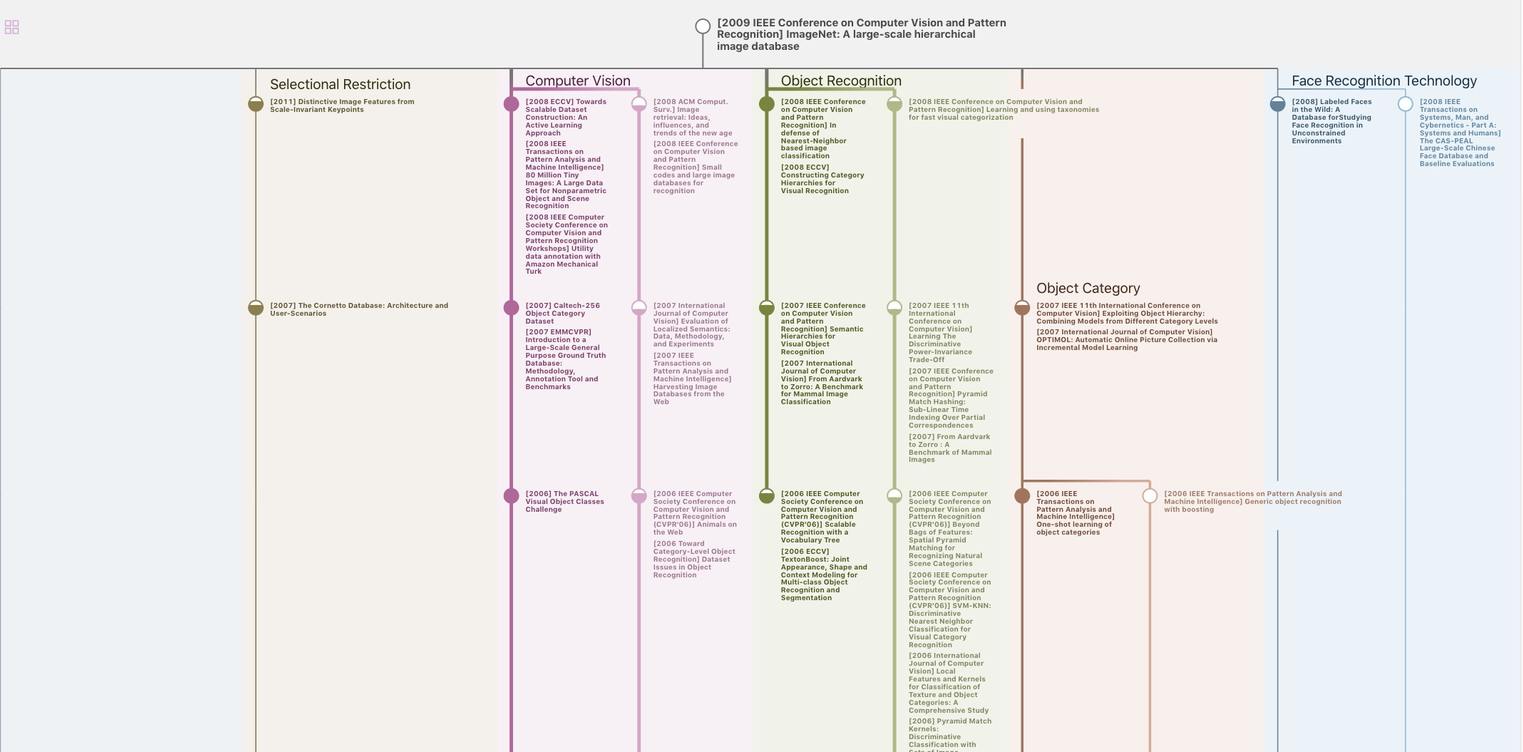
生成溯源树,研究论文发展脉络
Chat Paper
正在生成论文摘要