Deep Learning-Based Pilotless Spatial Multiplexing
CoRR(2023)
摘要
This paper investigates the feasibility of machine learning (ML)-based
pilotless spatial multiplexing in multiple-input and multiple-output (MIMO)
communication systems. Especially, it is shown that by training the transmitter
and receiver jointly, the transmitter can learn such constellation shapes for
the spatial streams which facilitate completely blind separation and detection
by the simultaneously learned receiver. To the best of our knowledge, this is
the first time ML-based spatial multiplexing without channel estimation pilots
is demonstrated. The results show that the learned pilotless scheme can
outperform a conventional pilot-based system by as much as 15-20% in terms of
spectral efficiency, depending on the modulation order and signal-to-noise
ratio.
更多查看译文
关键词
Spatial Multiplexing,Multiple-input Multiple-output,Order Modes,Spectral Efficiency,Channel Estimation,Neural Network,Activation Function,Deep Learning,Convolutional Neural Network,Artificial Neural Network,Likelihood Ratio Test,Output Layer,Hidden Layer,Weighting Factor,Physical Layer,Loss Term,Binary Cross Entropy,Binary Cross-entropy Loss,Orthogonal Frequency Division Multiplexing,Receiver Side,Block Error Rate,Orthogonal Frequency Division Multiplexing Symbol,Constellation Points,Linear Activation Function,Low-density Parity-check
AI 理解论文
溯源树
样例
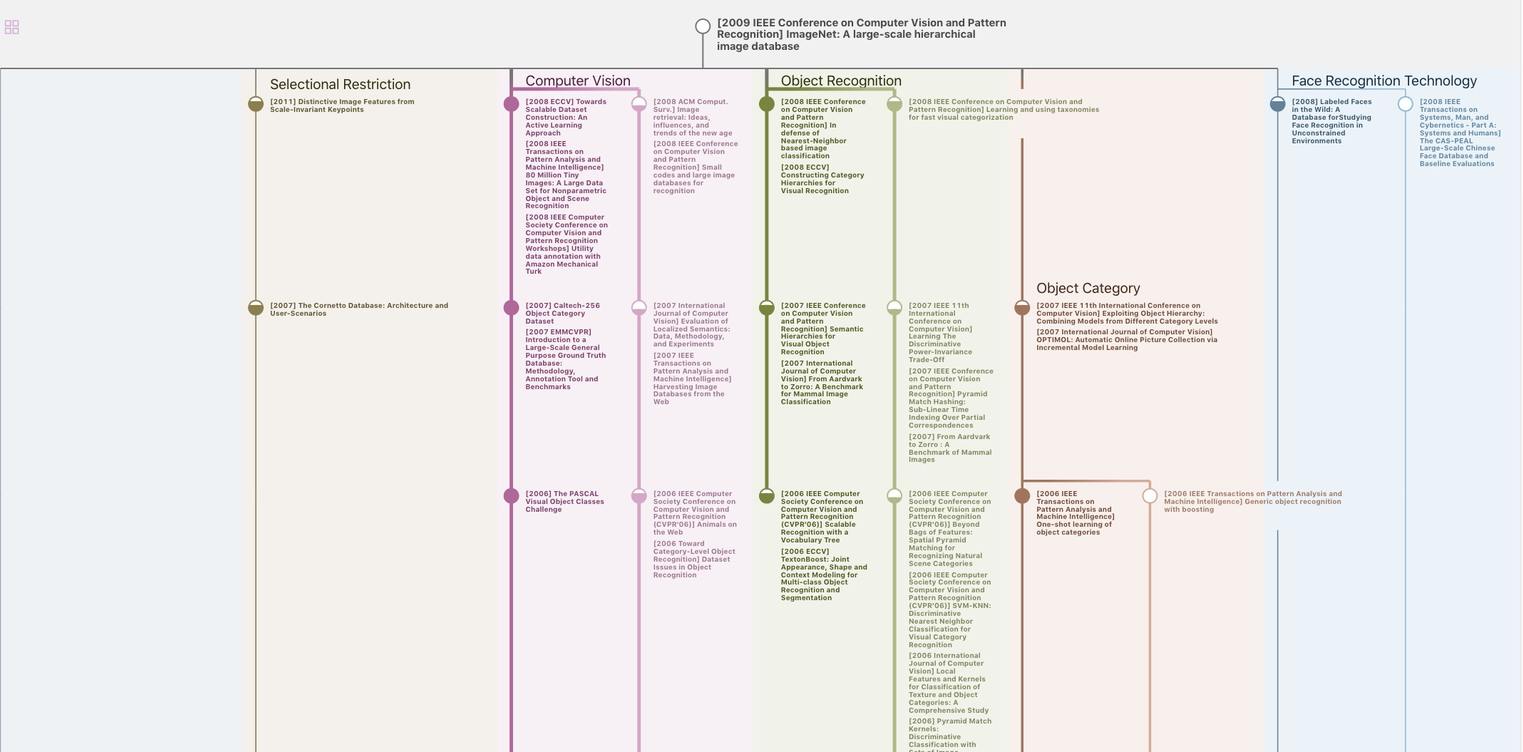
生成溯源树,研究论文发展脉络
Chat Paper
正在生成论文摘要