Structural Forecasting for Short-term Tropical Cyclone Intensity Guidance
arXiv (Cornell University)(2022)
摘要
Geostationary satellite (GOES) imagery provides a high temporal resolution window into tropical cyclone (TC) behavior. We present a new method for probabilistic forecasting not only of TC intensity but also TC convective structure as revealed by GOES. This paper describes a prototype model based solely on observed infrared imagery and operational intensity estimates up to 6 hours prior to the current time. These structural forecasts simulate the spatio-temporal evolution of the radial profiles of cloud-top temperatures over the subsequent 12 hours. Our structural forecasting pipeline applies a Deep Autoregressive Generative Model (PixelSNAIL) to create probabilistic forecasts of the evolution of these functions over time. A standard convolutional neural network (trained for ``nowcasting'', or predicting the current response based on prior response and current features) is then applied to simulated structural trajectories to estimate the current intensity and forecast intensities at +6 and +12 hours. Intensity guidance from our prototype model achieves a marginally higher error than the National Hurricane Center's official forecasts. However, our prototype model does not account for environmental factors such as vertical wind shear and sea surface temperature. We also demonstrate that it is possible to reasonably predict short-term evolution of TC convective structure as depicted by infrared imagery, producing interpretable structural forecasts that may be valuable for TC operational guidance.
更多查看译文
关键词
guidance,short-term
AI 理解论文
溯源树
样例
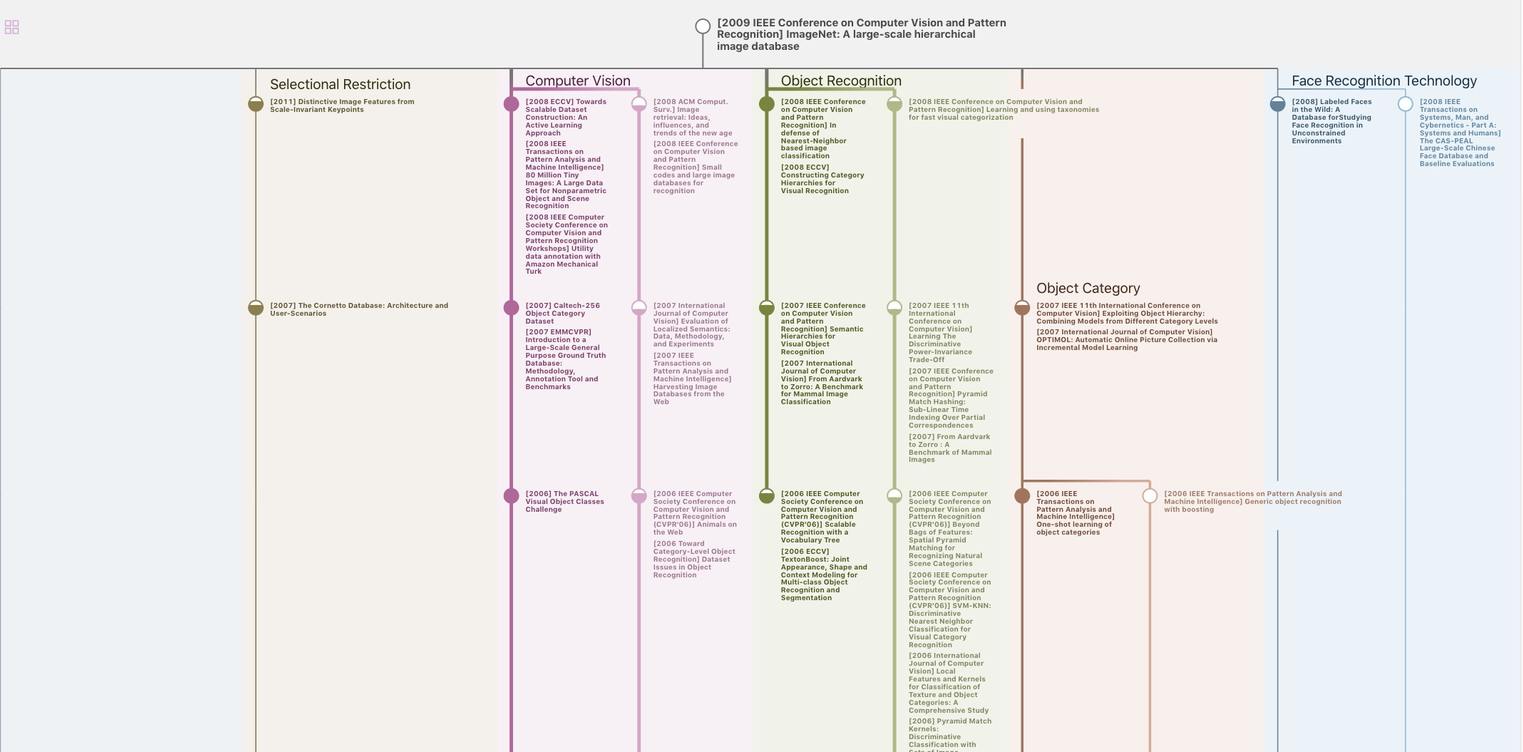
生成溯源树,研究论文发展脉络
Chat Paper
正在生成论文摘要