A Few-Shot Approach to Resume Information Extraction via Prompts
arXiv (Cornell University)(2023)
摘要
Prompt learning's fine-tune performance on text classification tasks has attracted the NLP community. This paper applies it to resume information extraction, improving existing methods for this task. We created manual templates and verbalizers tailored to resume texts and compared the performance of Masked Language Model (MLM) and Seq2Seq PLMs. Also, we enhanced the verbalizer design for Knowledgeable Prompt-tuning, contributing to prompt template design across NLP tasks. We present the Manual Knowledgeable Verbalizer (MKV), a rule for constructing verbalizers for specific applications. Our tests show that MKV rules yield more effective, robust templates and verbalizers than existing methods. Our MKV approach resolved sample imbalance, surpassing current automatic prompt methods. This study underscores the value of tailored prompt learning for resume extraction, stressing the importance of custom-designed templates and verbalizers.
更多查看译文
关键词
resume information extraction,few-shot
AI 理解论文
溯源树
样例
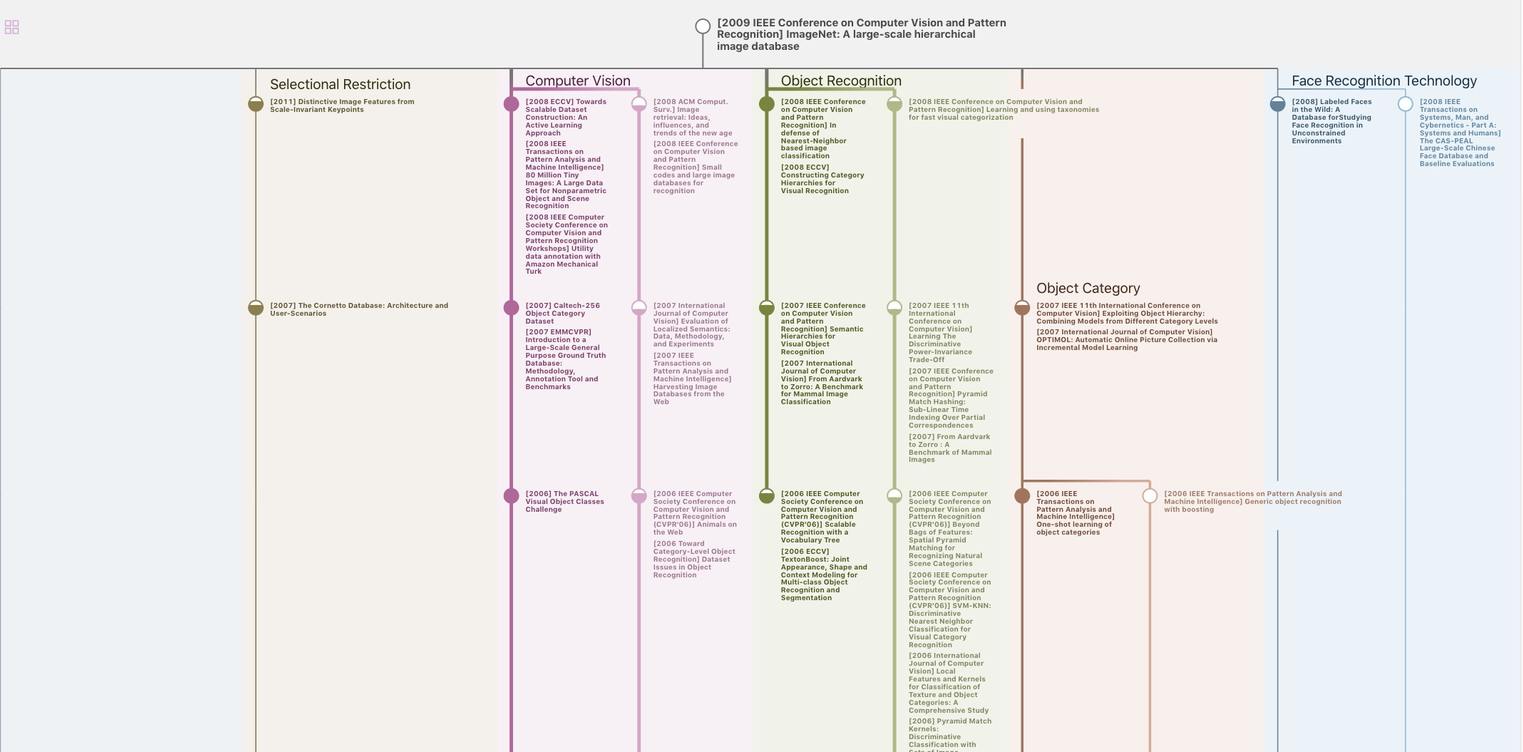
生成溯源树,研究论文发展脉络
Chat Paper
正在生成论文摘要